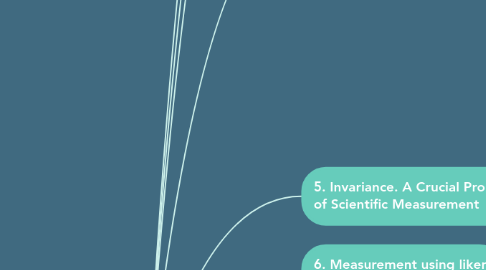
1. 1. Why Measurement is fundamental
1.1. 1.1 Children can construct meausures
1.2. 1.2 Statistics and/or Measurement
1.2.1. Stevens's framework
1.2.2. statistical analysis has dominated social sciences
1.3. 1.3 Why Fundamental Measurement?
1.4. 1.4 Derived Measures
1.5. 1.5 Conjoint Measurement
1.6. 1.6 The Rasch Model for Measurement
1.7. 1.7 A Suitable Analogy for Measurement in the Human Sciences
1.8. 1.8 In Conclusion
1.8.1. Authors
1.8.1.1. Alder (2002)
1.8.1.2. Keay (2000)
1.8.1.3. Sobel (1996)
1.8.1.4. Chang(2004)
1.8.1.4.1. Metrologist
1.8.1.5. Sherry (2011)
1.8.1.6. Michell (1999)
1.8.1.6.1. (1999)
1.8.1.6.2. (2003)
1.8.1.7. Bond (2001)
1.8.2. Relationship between Scientific measurement and the ideas of Luce and Rasch
1.8.3. Fundamental
1.8.3.1. Fundamental measurement
1.8.3.1.1. Scales with iterative unit values
1.8.3.1.2. many measurement scales in the physically sciences
1.8.3.2. Principles and properties of conjoint measurement that would bring the same sort of rigorous measurement to the human science as to the physical science
1.8.3.2.1. The Rasch Model of measurement is the closest generally accesible approximation of these
1.8.3.3. The fundamental and derived measurement systems of the are special cases of conjoint measurement
1.8.3.4. Conjoint measurement = a new type of fundamental measurement
1.9. 1.9 Summary
1.9.1. measurement vs statistics
2. 2. Important principles of measurement made explicit
2.1. Introductory
2.1.1. all of our investigatory observations are qualitative, and the classification or identification of events deals with data at the nominal level.
2.1.2. Nominal level data:
2.1.2.1. We observe just those events that are the focus of our enquiry and not others
2.1.2.2. We classify observations into types
2.1.3. Ordinal level data:
2.1.3.1. We record which of those observed events is better than another
2.1.3.2. We classify observations into levels
2.1.4. Interval scale
2.1.4.1. The distances between scale units are made equal and meaningful
2.1.5. Guttman pattern
2.1.6. Prediction of success
2.1.6.1. The further the item is embedded in the student´s zone of success, the more likely it is that the student will succeed on that item.
2.1.6.2. The likelihood of failure increases the further the item is embedded in the student's zone of failure
2.1.7. The real problem
2.1.7.1. We routinely mistake the distaces between fraction or percentage scores as having direct interval scale meaning, when all whe really may infer from these data is the ordering of the persons or the items
2.1.8. we need a sound way of interpeting the size of the gaps between the scores so that we are able to say that a student shows more ability than another in this and many test
2.1.9. Data matrix
2.1.9.1. Original
2.1.9.2. ordered data
2.1.9.3. Matrix boolean data
2.2. Moving from observation to measures
2.2.1. Work of Thurstone about mathematical procedure for better representing the relative distances between the raw scores
2.2.1.1. involves converting the raw score summary to its natural logarithm
2.2.2. The basic Rasch assumptions are that
2.2.2.1. a) each person is characterized by an ability, and
2.2.2.2. b) each item is characterized by a difficulty that
2.2.2.3. c) can be expressed by numbers along one line. Finally,
2.2.2.4. d) from the difference between the numbers (and nothing else), the probability of observing any particular scored response can be computed.
2.2.3. properties that is necessary to consider
2.2.3.1. It should be sensitive to the ordered acquisition of the skills or abilities under investigation
2.2.3.1.1. it should aim at uncovering the order of development or acquisition
2.2.3.2. It should be capable of estimating the developmental distances between the ordered skills or persons
2.2.3.2.1. How much Person A is more ... than person B
2.2.3.3. it should allow us to determine whether the general developmental pattern shown among items and persons is sufficient to account for the pattern of development shown by every item and every person
2.3. Summary
2.3.1. logical and practical steps necessary to construct interval-level measures from ordinal level observations
2.3.2. Steps to construct a linear measure of an ability
2.3.2.1. transform the ordinal-level data to interval-level measures
2.3.2.1.1. when the responses are merely qualitative observations and total 'number of correct' responses forms merely an ordinal level score, we can proceed to:
2.3.2.1.2. eliminate the compression at the ends of raw scale
2.3.3. we can preceed to examine a host of useful diagnostics to aid us in uncovering the extent to which the persons and items actually form an interval-scale linear measure
2.4. Suggested readings
2.4.1. Introductory
2.4.2. Advanced
2.5. Activities
3. 3. Basic Principles of the Rasch Model
3.1. The Path analogy
3.2. Unidimensionality
3.3. Item Fit
3.4. Difficulty / Ability Estimation and Error
3.5. Reliability
3.6. A Basic Framework for Measurement
3.7. Estimation (Difficulty, Ability and Precision)
3.8. Fit (Quality Control)
3.9. The Rasch Model
3.10. References
4. 4. Building a set of items for measurement
4.1. The Nature of the Data
4.1.1. What happens when to mistake the dichotomous level of data as being nominal?
4.1.2. What happens with coding a rating scale?
4.2. Analyzing Dichotomous Data: The BLOT
4.2.1. Bond´s Logical Operations Tests
4.3. A Simple Rasch Summary: The Item Pathway
4.4. Item Statistics
4.5. Item Fit
4.6. The Wright Map
4.6.1. Targeting
4.7. Comparing Persons and Items
4.7.1. Figure 4.3
4.7.1.1. Map
4.7.2. Table 4.3 Sumary of the BLOT Analysis Results
4.7.2.1. Table
4.8. Summary
4.8.1. The item-person Wrigtht map shows person and item distribution but not SE or fit.
4.8.2. Reliability of item is driven primarily by N persons and vice versa
4.9. Activities
4.10. Extended Understanding- Chapter 4
4.10.1. The problem of Guessing
4.10.2. Difficulty, Ability and Fit
4.10.3. The Theory-Practice Dialogue
4.10.3.1. What is the PRTIII?
4.10.4. Summary
4.10.4.1. Guessing is an off-trait behavior that might be prevalent in multiple-choice
4.10.4.1.1. ... and is not an item property but, more likely, a property of particular item difficulty-person ability combinations
4.10.4.2. What is ICCs?
4.10.4.3. What is Fit statistics?
4.10.4.4. How both can be used diagnostically to investigate the existence and potential impact of guessing?
4.10.5. Activities-Extended Understandig
4.11. References
5. 5. Invariance. A Crucial Property of Scientific Measurement
5.1. A Crucial Property of Scientific Measurement
5.1.1. "You can´t measure change with a measure that changes"
5.1.2. The measures should be invariant.
5.1.2.1. So the invariance requirement is that values (measures) atributed to variables by any measurement system should be independet of the particular measurment instrument used (as long as the instrument is appropiate to the purpose)
5.1.2.2. For any one device, ther readings will remain invariant across all suitable contexts. and for any one context, all suitably calibrated devices will yield invariant readings
5.2. Person and Item Invariance
5.2.1. The group of test items themselves is merely a sample of all possible test items in the same way that the group of persons who took the test is only a sample of the population...
5.2.1.1. relative ease of capturing a new sample of suitable test candidates and the relative difficulty of constructing a new sample of suitable test items.
5.2.1.2. In Rasch measurement, the principles and logic of analysis and interpretation for persons completely mirror the analytical principles for items.
5.2.2. The Rasch model supports direct comparisons of person ability and item difficulty estimates
5.2.2.1. Simply divide your sample of persons in two according to their ability and estimate the item difficulties for each half of the rest.
5.2.2.1.1. Simply divide your test into two and estimate the person abilities for each half of the test: Person ability estimates should remain invariant relative to each other regardless of which half of the sample test items is used for the person estimation
5.2.2.2. The Rasch measurement instantiates interval-level measurement, so it is the size of the intervals which must remain invariant.
5.2.2.2.1. Independently of the distribution of those abilities and difficulties in the particular samples of persons and items under examination.
5.3. Common Item Linking
5.3.1. Figure 5.1 Item difficulty invariance - BOND
5.3.1.1. Figure 5.1 Item difficulty invariance - BOND
5.3.2. Key Points to Keep in Mind
5.3.2.1. Table 5.1 BLOT Item Estimates and Errors Based on Split Half Subsamples. Errors Increase Dramatically With OFF-Target (Hig-Ability) Respondents.
5.3.2.1.1. Table 5.1
5.3.2.2. Figure 5.2 Item difficulty invariance - BLOT UK vs Australia
5.3.2.2.1. Figure 5.2
5.4. Anchoring Item Values
5.5. Common-person Linking
5.5.1. Figure 5.3 Invariance of BLOT person abilities, each estimated with half the items (odd vs even)
5.5.1.1. Figure 5.3
5.6. Invariace of Person Estimate Across Tests: Concurrent Validity
5.7. The PRTIII-Pendulum
5.7.1. What is mean of PRTIII-Pendulum?
5.7.1.1. Why ...III?
5.7.1.2. Why Pendulum?
5.7.2. Figure 5.4 Variable pathway for PRTIII-Pendulum
5.7.2.1. Figure 5.4
5.8. Common-Person Linking (2)
5.8.1. Table 5.2 Item Statistics for PRTIII-Pendulum
5.8.1.1. Table 5.2
5.8.2. Figure 5.5 Common person linking BLOT and PRTIII
5.8.2.1. Figure 5.5
5.8.3. Relationship between PRTIII ability and BLOT ability
5.8.3.1. Equation
5.8.4. Table 5.3 Raw Scores and Ability Estimates With Errors on the PRTIII and BLOT for a Subsample of Students
5.8.4.1. Table 5.3
5.8.5. Figure 5.6 (a) good correlation/low invariance (b) low correlation/good invariance
5.8.5.1. Figure 5.6
5.9. The Theory-Practice Dialogue
5.10. Measurement Invariance: Where It Really Matters
5.11. Failures of Invariance: DIF
5.11.1. Figure 5.7 Comparisons of boys' and girls' performances on BLOT items: #3 (no-DIF) and # 35 (gender-DIF)
5.11.1.1. Figure 5.7
5.12. Summary
5.12.1. Person and items measures should remain invariant (within error) across all appropiate measurement conditions.
5.12.2. Instruments measuring one latent trait should retain their calibrations (item difficulties) in all appropriate measuremet conditions (within error)
5.12.3. Person measures on one latent trait should remain identical (within error) regardles of which appropriate instrument is used.
5.12.4. In Rasch analysis, the computational logic applied to persons in relation to items is exactly the same as the logic applied to items in relation to persons.
5.12.5. Anchoring item values establishes measurement invariance for those items in the related analysis, but invariance must be monitored empirically to detect item 'drift' and to diagnose its likely causes.
5.12.5.1. What is the item "drift"?
5.12.6. Failures of invariance shoud alert us to potencital problems with the measurment instrument or to new understandings about the underlying latent trait.
5.13. Questions
5.13.1. In Rasch measurement, the principles and logic of analysis and interpretation for persons completely mirror the analytical principles for items.
6. 6. Measurement using likert scales
7. 7. The Partial Credit Rasch Model (PCM)
7.1. Introduction
7.1.1. The principles of measurement require that the part marks be awareded in an ordered way, so that each increasing score represents an increase in the underlying ability being tested.
7.1.2. How we can to establish the number of thresholds?
7.2. Clinical Interview Analysis: A Rasch-Inspired Breakhhrough
7.3. Scoring Interview Transcripts
7.3.1. Ordered Performance Criteria for 18 Aspects of the Pendulum Interview Task
7.4. Partial Credit Model Results (PCM)
7.5. Interpretation
7.6. The theory-Practice Dialogue
7.7. Unidimensionality
7.8. Summary
7.8.1. The PCM allows for the number and calibrations of response thresholds to vary across items
7.8.2. Application of the PCM offers potential for bridging the qualitative/quantitative divide that permeates much human science research.
7.9. Extended Understanding
7.9.1. Category Functioning
7.9.2. Point-Measure Correlations
7.9.3. Fit Statistics
7.9.4. Dimensionality: Primary Components Factor Analysis of the Rasch Residuals
7.10. Summary Extended Understanding
7.10.1. Category Characteristic Curves provide a rather simple way of checking whether the categories used in any Partial Credit Model analysis actually function as expected.
7.10.2. A simple Rasch measurement principle for ordered categories requires that the higher category should have the higher average measure of persons responding in that category.
7.10.3. Then, the ordering of response categoryes form lowest to highest should be matched by the ordering of the average of the person measures for those categories.
7.10.4. Safer conclusions can be drawn from larger samples; adopting a minimum count of, say, 10 per category would be better for resolving response category issues.
7.11. Questions
7.11.1. pg 159. "They are partial marks, not part marks"
7.11.2. pg 164 "A simple Rasch measurement principle for ordered categories requires that the higher category should have the higher average measure of persons responding in that category."
7.11.3. pg 164 "Then, the ordering of response categoryes form lowest to highest should be matched by the ordering of the average of the person measures for those categories."
7.11.4. pg 164 "Safer conclusions can be drawn from larger samples; adopting a minimum count of, say, 10 per category would be better for resolving response category issues."
7.11.5. pg 141 What is Substantive theory?
8. 8. Measuring facets beyond ability and difficulty
8.1. Introduction
8.1.1. Human abilities are regarded as unidimensional
8.1.2. Rasch approach is simple, not simplistic
8.1.3. Wright (1998)
8.1.3.1. "I don't want to know which questions you answered. I want to know how much ... you know. I need to leap from what I know (observed raw score) and don't want -- to what I want (ability estimate) but can't know. That's called inference"
8.1.4. So far, two facets
8.1.4.1. Level of ability or attitude expressed by the person
8.1.4.2. Level of difficulty or endorsability of the item, stem, or prompt.
8.2. A basic Introduction to the Many-Facets Rasch Model
8.2.1. Figure Logit Scales
8.2.1.1. Figure Logit Scales
8.3. Why Not Use Interrater Reliability?
8.3.1. Table 8.1 Rating for Eight Cadidates by Judge Easy and Judge Tough
8.3.1.1. Table 8.1 Rating for Eight Cadidates by Judge Easy and Judge Tough
8.4. Relations Among the Rasch Familly of Models
8.5. Data Specifications of the Many-Facets Rasch Model
8.6. Rating Cretivity of Junior Scientists
8.6.1. Table 8.2 Ratings of Seven Junior Scientists on Five Creativity Traits by Three Senior Scientists
8.6.1.1. Table 8.2 Ratings of Seven Junior Scientists on Five Creativity Traits by Three Senior Scientists
8.6.2. Data
8.6.2.1. Data first part
8.6.2.2. Data second part
8.6.3. Figure 8.1 Pathway of the many-facets Rasch analysis of scientists' ratings
8.6.3.1. Figure 8.1 Pathway of the many-facets Rasch analysis of scientists' ratings
8.6.4. Figure 8.2 Wright map for the many-facets Rasch analysis of scientists' ratings
8.6.4.1. Figure 8.2 Wright map for the many-facets Rasch analysis of scientists' ratings
8.7. Many-Facets Analysis of Eighth-Grade Writing
8.7.1. Figure 8.3 Calibrations of rater severity, writing task, and writing domain difficulties
8.7.1.1. Figure 8.3 Calibrations of rater severity, writing task, and writing domain difficulties
8.7.2. Table 8.3 Observed and Expected Ratings for Selected Students
8.7.2.1. Table 8.3 Observed and Expected Ratings for Selected Students
8.8. Summary
8.9. Extended Understanding -- Chapter 8
8.9.1. Invariance of Rated Creativity Scores
8.9.1.1. Figure 8.4 Judge B's candidate meausres plotted againts those of Judges A and C together. And approximate expectation line is drawn in. Points are identified by junior scientist code
8.9.1.1.1. Figure 8.4 Judge B's candidate meausres plotted againts those of Judges A and C together. And approximate expectation line is drawn in. Points are identified by junior scientist code
8.9.2. Rasch Measurement of Facets Beyond Rater Effects
8.9.3. Summary
8.9.4. Suggested Reading