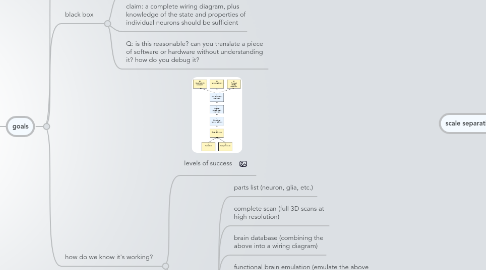
1. sources
1.1. Sanders and Bostrom: Brain Emulation Roadmap (2008)
1.2. (Review Article)
2. conclusions
2.1. whole brain scanning seems feasible
2.2. whole brain emulation is becoming feasible over the next few decades, barring unexpected upsets in the improvement of computer performance
3. feasiblity
3.1. do we know enough?
3.1.1. brain emulation won't work if we don't even know about some important circuit elements
3.1.2. scanning issues (if you don't scan it, you lose it)
3.1.2.1. do we know all the neurotransmitters?
3.1.2.2. do we know all the channels? about 71 channel subunits from H. sapiens, combining in complicated ways
3.1.2.3. is the dynamic state of the brain important?
3.1.3. simulation issues (fixable later)
3.1.3.1. are synapses everything? non-synaptic communication: diffusable messengers, glial cells, ephaptic effects
3.1.3.2. how important are neurogenesis and synaptogenesis?
3.2. scanning
3.2.1. (separate slides describing the technologies)
3.2.2. generally, a resolution of 5nm x 5nm x 50nm, achievable with electron microscopes
3.2.3. automatic slicing and microscopy, achievable with current technologies
3.2.4. similar to the human genome project in size and scope
3.3. do we have the computational resources?
3.3.1. depends on the scale of the simulation
3.3.2. estimates of brain computational power
3.3.2.1. memory
3.3.2.1.1. 10^16 bits (10^10 neurons, 1000 synapses, 34bit ID, 8 bit representation of state; Leitl 1995)
3.3.2.1.2. 10^20 bits (microtubule memory; Tuszynski, 2006)
3.3.2.1.3. 10^28 bits (10^11 neurons, 10^4 compartments, 4 dynamic variables and 10 parameters; Malickas, 1996)
3.3.2.1.4. compare
3.3.2.2. CPU
3.3.2.2.1. 10^14 ops/s (10^10 neurons, 1000 synapses, 10 Hz; Freitas, 1996)
3.3.2.2.2. 10^17 ops/s (10^11 neurons, 10^4 synapses, 100 Hz, 5 bits/signal; Bostrom, 1998)
3.3.2.2.3. 10^14 ops/ (retina scale up; Merkle, 1989)
3.3.2.2.4. 10^18 ops/s (10^11 neurons, 10^4 compartments, Hodkin-Huxley, 1200 FLOPS)
3.3.2.2.5. compare
3.3.3. more data
3.3.3.1. current simulations
3.3.3.1.1. (lots more)
3.3.3.2. storage
3.3.3.2.1. memory
3.3.3.2.2. access times
3.3.3.2.3. disk storage
3.3.3.3. processing
3.3.3.3.1. MIPS over time (10x = 7.1 years)
3.3.3.3.2. MIPS/# over time
3.3.3.3.3. computer performance per dollar over time
3.3.3.3.4. top 500
4. roadmap
4.1. research cycle
4.1.1. research usually consists of many steps and incremental improvements
4.1.2. it's useful to think about how to organize this cycle so that you achieve continuous improvement (think about it in your own work too)
4.1.3. the research cycle
4.2. overlap with other fields
4.2.1. which aspects of brain emulation research are reusable in other fields?
4.2.1.1. very large scale simulation
4.2.1.2. environment simulation
4.2.1.3. supercomputing
4.2.1.4. fault tolerance
4.2.1.5. virtual cell, brain, and body models for medical research
4.2.2. who might be motivated to fund some of this work?
4.2.3. what other applications provide economies of scale?
4.2.4. are there related consumer goods that might provide economies of scale? (gaming, household robotics, etc.)
4.3. model system
4.3.1. it's a bad idea to start with solving the most complex problem right away; pick a model system instead
4.3.2. C. elegans
4.3.3. 302 neurons
4.3.4. eutelic system
4.3.5. simple behavior
4.3.6. well studied
4.3.7. easy genetics
4.3.8. doesn't bite
4.4. impact
4.4.1. economic impact is important both for consequences of the research and funding
4.4.2. What would happen if you could "buy a mind", with or without attached arm?
4.4.2.1. $100m
4.4.2.2. $1m
4.4.2.3. $100k
4.4.2.4. $1000
4.4.3. Who is interested in funding it?
5. subproblems
5.1. scanning
5.1.1. preparation
5.1.2. physical handling
5.1.3. imaging
5.1.3.1. resolution
5.1.3.2. volume
5.1.3.3. functional information
5.2. translation
5.2.1. image processing
5.2.1.1. geometric adjustment
5.2.1.2. data interpolation
5.2.1.3. noise removal
5.2.1.4. tracing
5.2.2. scan interpretation
5.2.2.1. parameter estimation
5.2.2.2. connectivity identification
5.2.2.3. synapse identification
5.2.2.4. cell type identification
5.2.2.5. databasing
5.3. simulation
5.3.1. environment simulation
5.3.2. body simulation
5.3.3. distributed computation
5.3.4. fault tolerance
5.3.5. providing adequate resources
5.4. (based on Sanders and Bostrom, 2008; based on workshop participants)
6. hypotheses
6.1. philosophical
6.1.1. physicalism - the mind is a purely physical phenomenon
6.1.2. Turing equivalence - there is no hypercomputation required in the brain
6.2. technical
6.2.1. non-organicism - you don't need organic neurons to create a mind
6.2.2. scale separation - there is some scale below which the details of brain function can be abstracted as aggregate properties
6.2.3. scannability - all relevant properties can actually be scanned
6.3. biological
6.3.1. brain-centeredness - all you really need for a mind is a brain (plus some I/O)
6.4. These are important questions in physics, biology, and neuroscience.
6.5. The way we test hypotheses is by experiment, and for many of these hypotheses, emulation is the obvious experiment to do.
7. scale separation
7.1. the nervous system operates at many different scales
7.2. how do we simulate this? down to the atom? molecule? neuron?
7.3. can we describe the system at different scales? can we abstract functionality at high resolution?
7.4. we need at least one cut-off where we stop emulating greater details
7.5. observation: microstimulation (individual neurons) can produce macroscopic changes in behavior
7.6. but: in software engineering, we have many scales at which we compose software systems, yet changing a single bit somewhere can bring the whole system crashing down
7.7. detailed levels of emulation
7.8. estimates
8. goals
8.1. emulation vs simulation
8.1.1. simulation = a computational model of some aspect of a system (not complete)
8.1.2. emulation = a full computational model of an entire system; all relevant properties are modeled
8.1.3. brain emulator = software running on non-neural hardware emulating the brain
8.1.4. mind emulator = brain emulator that succeeds at creating a mind
8.2. black box
8.2.1. claim: we do not need to understand the system in order to emulate it
8.2.2. claim: a complete wiring diagram, plus knowledge of the state and properties of individual neurons should be sufficient
8.2.3. Q: is this reasonable? can you translate a piece of software or hardware without understanding it? how do you debug it?
8.3. how do we know it's working?
8.3.1. levels of success
8.3.2. levels
8.3.2.1. parts list (neuron, glia, etc.)
8.3.2.2. complete scan (full 3D scans at high resolution)
8.3.2.3. brain database (combining the above into a wiring diagram)
8.3.2.4. functional brain emulation (emulate the above generically, and produce some general properties of a brain)
8.3.2.5. species-generic brain emulation (produce the full range of normally observed behaviors)
8.3.2.6. social role-fit emulation (the simulation is accepted by others in at least some social roles; e.g. game avatar)
8.3.2.7. mind emulation (the emulation experiences mental states like humans)
8.3.2.8. personal identity emulation (the emulation describes itself as a continuation of the original mind)