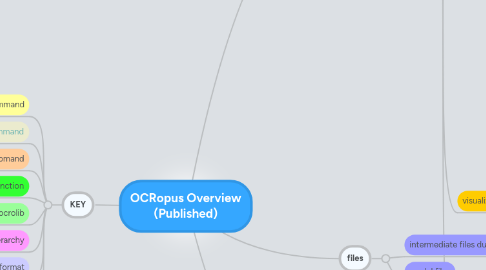
1. KEY
1.1. this is a command
1.2. this is an obsolete command
1.3. this is an unimplemented comand
1.4. this is a group of modules by function
1.5. this is a module in ocrolib
1.6. this is a file name in the directory hierarchy
1.7. this is a file format
1.8. click on the +/- signs to expand/collapse nodes
2. commands
2.1. recognition
2.1.1. Tools for recognizing text on collections of pages.
2.1.2. ocropus-nlbin
2.1.2.1. purpose
2.1.2.1.1. noise and border removal
2.1.2.1.2. page rotation correction
2.1.2.1.3. grayscale normalization
2.1.2.1.4. binarization
2.1.2.2. usage
2.1.2.2.1. ocropus-nlbin image1.png image2.png ...
2.1.2.2.2. inputs
2.1.2.2.3. outputs
2.1.2.3. notes
2.1.2.3.1. optimized for 300dpi book pages
2.1.2.3.2. other kinds of inputs may require parameter adjustments
2.1.2.4. parameters
2.1.2.4.1. TBD
2.1.3. ocropus-gpageseg
2.1.3.1. purpose
2.1.3.1.1. column finding
2.1.3.1.2. text line finding
2.1.3.2. usage
2.1.3.2.1. ocropus-gpageseg page1.png page2.png ...
2.1.3.2.2. ocropus-gpageseg 'book/????.png'
2.1.3.2.3. inputs
2.1.3.2.4. outputs
2.1.3.3. notes
2.1.3.3.1. optimized for 300 dpi book pages
2.1.3.3.2. other kinds of inputs may require parameter adjustment
2.1.3.3.3. this program is really more of a placeholder and will be replaced by trainable layout analysis
2.1.3.4. limitations
2.1.3.4.1. no text/image segmentation; pages with complex figures will result in a lot of "noise"
2.1.3.4.2. column finding performance is variable depending on the input documents
2.1.3.4.3. text lines printed in very large fonts will get erroneously split horizontally, leading to misrecognition
2.1.3.5. TODO
2.1.3.5.1. improve column finding
2.1.3.5.2. add image detection / removal
2.1.4. ocropus-rpred
2.1.4.1. purpose
2.1.4.1.1. apply an neural network recognizer
2.1.4.2. usage
2.1.4.2.1. ocropus-rpred line1.png line2.png ...
2.1.4.2.2. inputs
2.1.4.2.3. outputs
2.1.4.3. notes
2.1.4.3.1. recognizer is currently trained on UW3 and UNLV data; you can load other models with -m
2.1.4.3.2. also performs text line normalization
2.1.4.4. limitations
2.1.4.4.1. current models are trained on modern type fonts and flatbed scans (UW3, UNLV); performance will be worse on other kinds of inputs
2.1.4.5. parameters
2.1.4.5.1. -m model.pyrnn
2.1.4.5.2. TBD
2.1.5. ocropus-hocr
2.1.5.1. purpose
2.1.5.1.1. outputs hOCR encoded recognition output
2.1.5.2. usage
2.1.5.2.1. ocropus-hocr bookdirectory -o book.html
2.1.5.3. notes
2.1.5.3.1. outputs hOCR format, including bounding boxes for text lines and per-line baseline information
2.1.5.3.2. also outputs font size information
2.1.5.4. TODO
2.1.5.4.1. lots of bug fixing and testing using the new pipeline
2.1.5.4.2. optionally output word and character bounding boxes
2.1.5.4.3. output more font-related information
2.2. training tools
2.2.1. Tools for training OCRopus to recognize new scripts and languages.
2.2.2. ocropus-rtrain
2.2.2.1. purpose
2.2.2.1.1. train a neural network recognizer
2.2.2.2. usage
2.2.2.2.1. ocropus-rtrain -o newmodel.pyrnn line1.png line2.png ...
2.2.2.2.2. inputs
2.2.2.2.3. outputs
2.2.2.3. notes
2.2.2.3.1. transcriptions should be in Unicode
2.2.2.3.2. internally, text lines will be normalized prior to training/recognition; the normalization process may need modification for some scripts
2.2.2.4. parameters
2.2.2.4.1. -s 4
2.2.2.4.2. TBD
2.2.3. ocropus-gtedit html
2.2.3.1. purpose
2.2.3.1.1. line-wise correction of input text
2.2.3.2. usage
2.2.3.2.1. ocropus-gtedit html -o mypage.html *.png
2.2.3.2.2. inputs
2.2.3.2.3. outputs
2.2.4. ocropus-gtedit extract
2.2.4.1. purpose
2.2.4.1.1. extract ground truth from an edited HTML file created with ocropus-gtedit
2.2.4.2. usage
2.2.4.2.1. ocropus-gtedit extract -o mydir mypage.html
2.3. visualization tools
2.3.1. Tools that helps with debugging and identifying the sources of recognition errors.
2.3.2. ocropus-showline
2.3.2.1. purpose
2.3.2.1.1. show text line recognition results and details
2.3.3. ocropus-showpage
2.3.3.1. purpose
2.3.3.1.1. show page recognition results and details
2.3.3.2. usage
2.3.3.2.1. ocropus-showpage book/0001.bin.png
2.3.3.2.2. ocropus-showpage book/0001.bin.png -o debug.png
2.3.3.3. TODO
2.3.3.3.1. ocropus-showpage 'book/????.bin.png' --index index.html
2.3.4. ocropus-visualize-results
2.3.5. ocropus-submit
2.3.5.1. purpose
2.3.5.1.1. submit a bug report
2.4. character-level recognizer
2.4.1. These are the tools for an older, character level recognizer.
2.4.2. recognition
2.4.2.1. ocropus-lattices
2.4.2.1.1. purpose
2.4.2.1.2. usage
2.4.2.1.3. notes
2.4.2.1.4. limitations
2.4.2.1.5. parameters
2.4.2.2. ocropus-ngraphs
2.4.2.2.1. purpose
2.4.2.2.2. usage
2.4.2.2.3. notes
2.4.2.2.4. parameters
2.4.2.2.5. limitations
2.4.2.2.6. TODO
2.4.3. character training
2.4.3.1. ocropus-tsplit
2.4.3.1.1. purpose
2.4.3.2. ocropus-tleaves
2.4.3.2.1. purpose
2.4.3.3. ocropus-wtrain
2.4.3.3.1. purpose
2.4.3.3.2. TODO
2.4.3.4. ocropus-db
2.4.3.4.1. purpose
2.4.3.4.2. subcommands
2.4.3.5. ocropus-cedit
2.4.3.5.1. purpose
2.4.3.5.2. TODO
2.4.4. language models
2.4.4.1. ocropus-ngraphs --build
2.4.4.1.1. purpose
2.4.5. data generation
2.4.5.1. ocropus-align
2.4.5.1.1. purpose
2.4.5.1.2. TODO
2.4.5.2. ocropus-lattices --extract
2.4.5.2.1. purpose
2.4.5.2.2. usage
2.4.5.3. ocropus-linegen
2.4.5.3.1. purpose
2.4.5.3.2. TODO
2.4.5.4. ocropus-db tess2h5
2.4.5.4.1. see above
2.4.6. visualization
2.4.6.1. ocropus-ngraphs --print
2.4.6.1.1. usage
2.4.6.1.2. purpose
3. files
3.1. intermediate files during recognition
3.1.1. file formats
3.1.1.1. File formats are documented externally; please follow the links.
3.1.1.2. .pseg.png
3.1.1.2.1. http://goo.gl/9IfXM
3.1.1.3. .rseg.png
3.1.1.3.1. http://goo.gl/9IfXM
3.1.1.4. .cseg.png
3.1.1.4.1. http://goo.gl/9IfXM
3.1.1.5. .lattice
3.1.1.5.1. http://goo.gl/9IfXM
3.1.1.6. .aligned
3.1.1.6.1. http://goo.gl/9IfXM
3.1.1.7. hOCR output
3.1.1.7.1. http://goo.gl/zXNrC
3.1.2. directory layout
3.1.2.1. Although many OCRopus commands work on individual files, there is a standard directory layout that some tools expect. It consists of a top level directory containing files of the form 0001.extension for each page, and containing subdirectories of the form 0001, that in turn contain files for each line.
3.1.2.2. book
3.1.2.2.1. 0001.bin.png
3.1.2.2.2. 0001.nrm.png
3.1.2.2.3. 0001.pseg.png
3.1.2.2.4. 0001
3.2. model files
3.2.1. These are the models responsible for recognition.
3.2.2. .cmodel
3.2.2.1. A pickled python object representing a character classifier.
3.2.2.2. members
3.2.2.2.1. coutputs(image) -> [(cls,prob),...]
3.2.2.2.2. sizemode
3.2.2.3. comments
3.2.2.3.1. training with "ocropus-tsplit" and "ocropus-tleaves" or other kinds of character training tools
3.2.2.3.2. primarily responsible for recognition of characters
3.2.2.3.3. cmodels may expect different size normalization of their inputs
3.2.3. .lineest
3.2.3.1. A pickled Python object with two methods returning polynomial models of the baseline and xline.
3.2.3.2. members
3.2.3.2.1. lineFit(image) -> (bl_poly,xl_poly)
3.2.3.3. comments
3.2.3.3.1. training with "python -m ocrolib.lineest" command
3.2.3.3.2. if the script is similar to Latin script, probably doesn't need retraining
3.2.3.3.3. Line estimators are only needed for scripts in which relative size and position of characters makes a significant difference. For most scripts other than Latin scripts this isn't the case.
3.2.4. .ngraphs
3.2.4.1. A pickled Python object representing conditional probabilities.
3.2.4.2. members
3.2.4.2.1. all the members are private (the class is only used by ocropus-ngraphs)
3.2.4.3. comments
3.2.4.3.1. training with "ocropus-ngraphs" command
3.2.4.3.2. this is easy to train, and needs to be trained even for different corpora
3.2.4.3.3. recognition performance is quite sensitive to the parameters given to ocropus-ngraphs (e.g., language model weight etc.); this tricky tradeoff between language models and character recognition is true of all OCR engines
3.2.5. .wmodel
3.2.5.1. A pickled Python object estimating the probabilities that there is a space at a particular location.
3.2.5.2. members
3.2.5.2.1. setLine(image)
3.2.5.2.2. classifySpace(x) -> (no,yes)
3.2.5.3. comments
3.2.5.3.1. the training tools for this is currently broken; it will be replaced
4. library
4.1. utility
4.1.1. default
4.1.1.1. a few default paths
4.1.1.2. this is also read by setup.py
4.1.1.3. TODO
4.1.1.3.1. rename to defaults.py
4.1.2. toplevel
4.1.2.1. decorators etc. intended to be imported at toplevel (very few)
4.1.2.2. in particular, contains the @checks decorator, which checks arguments to Python functions
4.1.2.3. there is a large number of argument checks defined, relevant to image processing and OCR
4.1.3. common
4.1.3.1. reading and writing of images, segmentation files, etc.
4.1.3.2. dealing with file names and directories
4.1.3.3. some general simple image processing functions
4.1.3.4. some unicode-related utilities
4.1.3.5. warnings
4.1.3.6. checks
4.1.3.7. loading and instantiating components
4.1.3.8. TODO
4.1.3.8.1. move exceptions and annotations to toplevel.py
4.1.3.8.2. use warning, checks, etc. more consistently
4.1.3.8.3. use mkpython more consistently
4.1.3.8.4. remove old make_I... functions
4.1.3.8.5. move rect_union to sl
4.1.3.8.6. move drawing functions into separate module
4.1.3.8.7. make gt_explode/implode into Python codec
4.1.4. sl
4.1.4.1. utilities for dealing with Python tuples-of-slices as rectangles
4.1.5. native
4.1.5.1. a small library from embedding C code inside Python
4.2. image processing
4.2.1. morph
4.2.1.1. morphological operations to completely scipy.ndimage.morphology
4.2.2. improc
4.2.2.1. various image processing operations
4.2.3. lineproc
4.2.3.1. image processing specifically related to text lines
4.2.4. psegutils
4.2.4.1. image processing specifically related to page images
4.3. pattern recognition
4.3.1. patrec
4.3.1.1. various pattern recognition algorithms
4.3.2. mlinear
4.3.2.1. linear classifiers, logistic regression
4.3.3. mlp
4.3.3.1. MLPs and backpropagation
4.4. recognition
4.4.1. wmodel
4.4.1.1. white space models
4.4.2. lineseg
4.4.2.1. line segmentation by connected components
4.4.2.2. line segmentation by dynamic programming cuts
4.4.3. linerec
4.4.3.1. text line recognition
4.4.4. lineest
4.4.4.1. line geometry estimation
4.4.4.2. finds the baseline and xline of text lines
4.4.4.3. trained models of the form name.lineest
4.5. text
4.5.1. lang
4.5.1.1. language and script related data
4.5.1.2. TODO
4.5.1.2.1. merge with ligatures.py
4.5.2. ligatures
4.5.2.1. encoding and decoding of ligatures as integers, related functions
4.5.3. lattice
4.5.3.1. reads lattice files and augments them with some transitions; used for both alignment and language modeling
4.5.3.2. notes
4.5.3.2.1. you should usually use linerec.read_lattice and linerec.write_lattice
4.5.3.3. TODO
4.5.3.3.1. change the name to something less generic
4.5.4. ngraphs
4.5.5. hocr