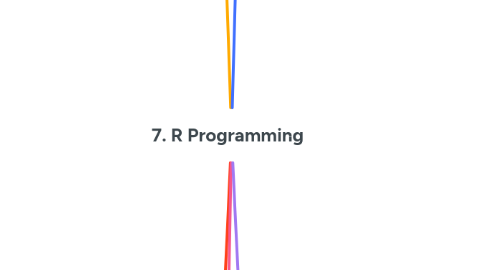
1. Working with data in R
1.1. Data frame
1.1.1. a collection of columns
1.1.1.1. columns should be named
1.1.1.2. data stored can be many different types, like numeric, factor, or character
1.1.1.3. each data frame should contain the same number of data items
1.2. Tibbles
1.2.1. are like streamlined data frames
1.2.1.1. never change the data types of the inputs
1.2.1.2. never change the names of your variables
1.2.1.3. never create row names
1.2.1.4. make printing easier
1.2.2. tibbles versus data
1.2.2.1. A data frame
1.2.2.1.1. a collection of columns, like a spreadsheet or a SQL table
1.2.2.1.2. Overall, you can make more changes to data frames, but **tibbles are easier to use**
1.2.2.2. Tibbles
1.2.2.2.1. streamlined data frames that are automatically set to pull up only the first 10 rows of a dataset, and only as many columns as can fit on the screen
1.2.2.2.2. Unlike data frames, **tibbles never change the names of your variables,** or the data types of your inputs
1.2.2.2.3. The tibble package is part of the core tidyverse
1.3. Tidy data
1.3.1. a way of standardizing the organization of data within R
1.3.2. standards
1.3.2.1. Variables are organized into columns
1.3.2.2. Observations are organized into rows
1.3.2.3. Each value must have its own cell
1.4. Cleaning data
1.4.1. File-naming conventions
1.4.1.1. Do
1.4.1.1.1. - Keep your filenames to a reasonable length - Use underscores and hyphens for readability - Start or end your filename with a letter or number - Use a standard date format when applicable; example: YYYY-MM-DD - Use filenames for related files that work well with default ordering
1.4.1.2. Don't
1.4.1.2.1. - Use unnecessary additional characters in filenames - Use spaces or “illegal” characters; examples: &, %, #, <, or > - Start or end your filename with a symbol - Use incomplete or inconsistent date formats; example: M-D-YY - Use filenames for related files that do not work well with default ordering
1.4.2. Using R functions
1.4.2.1. Clean
1.4.2.1.1. select()
1.4.2.1.2. skim_without_charts()
1.4.2.1.3. clean_names()
1.4.2.1.4. rename()
1.4.2.1.5. glimpse()
1.4.2.1.6. rename_with()
1.4.2.2. Organize
1.4.2.2.1. group_by()
1.4.2.2.2. drop_na()
1.4.2.2.3. max()
1.4.2.2.4. filter()
1.4.2.2.5. mean()
1.4.2.2.6. summarize()
1.4.2.2.7. arrange()
1.4.2.3. Transform
1.4.2.3.1. separate()
1.4.2.3.2. mutate()
1.4.2.3.3. unite()
1.5. R operators
1.5.1. Arithmetic
1.5.1.1. %% modulus
1.5.1.2. %/% integer division
1.5.2. Relational
1.5.3. Logical
1.5.4. Assignment
1.6. Anscombe's quartet
1.6.1. four datasets that have nearly identical summary statistics
1.7. bias function
1.7.1. finds the average amount that the actual outcome is greater than the predicted outcome
2. More about visualizations, aesthetics, and annotations
2.1. Create data viz in R
2.1.1. Functions
2.1.1.1. ggplot2
2.1.1.1.1. most popular and easy to use
2.1.1.1.2. benefits
2.1.1.1.3. core concepts
2.1.1.1.4. cheatsheet
2.1.1.1.5. steps
2.1.1.1.6. common coding errors in ggplot2
2.1.1.2. Plotly
2.1.1.3. Lattice
2.1.1.4. RGL
2.1.1.5. Dygraphs
2.1.1.6. Leaflet
2.1.1.7. Highcharter
2.1.1.8. Patchwork
2.1.1.9. gganimate
2.1.1.10. ggridges
2.2. Explore aesthetics in analysis
2.2.1. aesthetics for points
2.2.1.1. X
2.2.1.2. Y
2.2.1.3. Color
2.2.1.4. Shape
2.2.1.5. Size
2.2.1.6. Alpha
2.2.2. aesthetics in ggplot2
2.2.2.1. color
2.2.2.2. size
2.2.2.3. shape
2.2.3. geoms
2.2.3.1. geom functions
2.2.3.1.1. geom_point
2.2.3.1.2. geom_bar
2.2.3.1.3. geom_line
2.2.4. facet functions
2.2.4.1. facet_wrap()
2.2.4.2. facet_grid()
2.2.5. tilde (~)
2.2.5.1. Tilde operator is used to define the relationship between dependent variable and independent variables in a statistical model formula.
2.2.5.2. The variable on the left-hand side of tilde operator is the dependent variable and the variable(s) on the right-hand side of tilde operator is/are called the independent variable(s).
2.3. Annotate and save visualizations
2.3.1. annotate
2.3.1.1. to add notes to a document or diagram to explain or comment upon it
2.3.1.1.1. titles
2.3.1.1.2. subtitles
2.3.2. saving your viz
3. Documentation and reports
3.1. Develop documentation and reports in Rstudio
3.1.1. R Markdown
3.1.1.1. a file format for making dynamic documents with R
3.1.2. Jupyter notebooks
3.1.2.1. documents that contain computer code and rich text elements – such as comments, links, or descriptions of your analysis and results
3.2. Create R Markdown documents
3.2.1. YAML (yet another markup language)
3.2.1.1. a language for data that translates it so it's readable
3.3. Understand code chunks and exports
3.3.1. Code chunk
3.3.1.1. code added in an .Rmd file
3.3.2. Delimiter
3.3.2.1. a character that indicates the beginning or end of a data item
3.3.3. Code chunk delimiter
3.3.3.1. '''{r} and '''
4. Programming and data analytics
4.1. The R-versus-Python debate
4.1.1. Common features
4.1.1.1. R
4.1.1.1.1. - Open-source - Data stored in data frames - Formulas and functions readily available - Community for code development and support
4.1.1.2. Python
4.1.1.2.1. - Open-source - Data stored in data frames - Formulas and functions readily available - Community for code development and support
4.1.2. Unique advantages
4.1.2.1. R
4.1.2.1.1. - Data manipulation, data visualization, and statistics packages - "Scalpel" approach to data: find packages to do what you want with the data
4.1.2.2. Python
4.1.2.2.1. - Easy syntax for machine learning needs - Integrates with cloud platforms like Google Cloud, Amazon Web Services, and Azure
4.1.3. Unique challenges
4.1.3.1. R
4.1.3.1.1. - Inconsistent naming conventions make it harder for beginners to select the right functions - Methods for handling variables may be a little complex for beginners to understand
4.1.3.2. Python
4.1.3.2.1. - Many more decisions for beginners to make about data input/output, structure, variables, packages, and objects - "Swiss army knife" approach to data: figure out a way to do what you want with the data
4.2. Programming languages
4.2.1. Programming languages
4.2.1.1. the words and symbols we use to write instructions for computers to follow
4.2.1.1.1. benefits of using any programming language to work with your data
4.2.2. Coding
4.2.2.1. writing instructions to the computer in the syntax of a specific programming language
4.2.3. Ways to learn about programming
4.2.3.1. Data analyst
4.2.3.1.1. R
4.2.3.1.2. Python
4.2.3.1.3. Kaggle
4.2.3.2. Web designer
4.2.3.2.1. HTML5
4.2.3.2.2. CSS
4.2.3.3. Mobile application developer
4.2.3.3.1. Swift
4.2.3.3.2. Java
4.2.3.3.3. C#
4.2.3.4. Web application developer
4.2.3.4.1. Java
4.2.3.4.2. Python
4.2.3.4.3. Ruby
4.2.3.4.4. PHP
4.2.3.5. Game developer
4.2.3.5.1. C#
4.2.3.5.2. C++
4.2.4. Introduction to R
4.2.4.1. a programming language frequently used for statistical analysis, visualization and other data analysis
4.2.4.1.1. Accessible
4.2.4.1.2. Data-centric
4.2.4.1.3. Open source
4.2.4.1.4. Community
4.2.4.2. 3 scenarios
4.2.4.2.1. Reproducing your analysis
4.2.4.2.2. Processing lots of data
4.2.4.2.3. Creating data viz
4.2.5. Introduction to RStudio
4.2.5.1. RStudio's an IDE or integrated development environment
4.2.5.1.1. RStudio brings together all the tools you might want to use in a single place
5. Programming using RStudio
5.1. Understand basic programming concepts
5.1.1. The basic concepts of R
5.1.1.1. functions
5.1.1.1.1. a body of reusable code used to perform specific tasks in R
5.1.1.1.2. argument
5.1.1.2. comments (#)
5.1.1.3. variables
5.1.1.3.1. a representation of a value in R that can be stored for use later during programming
5.1.1.3.2. a variable name
5.1.1.4. data types
5.1.1.4.1. An attribute that describes a piece of data based on its values, its programming language, or the operations it can perform
5.1.1.5. vectors
5.1.1.5.1. a group of data elements of the same type stored in a sequence in R
5.1.1.5.2. two key properties
5.1.1.6. pipes
5.1.1.6.1. a tool in R for expressing a sequence of multiple operations, represented with "%>%"
5.1.2. Dates and times in R
5.1.2.1. lubridate package
5.1.2.2. tidyverse package
5.1.3. Other common data structures
5.1.3.1. data frames
5.1.3.1.1. a collection of columns containing data, similar to a spreadsheet or SQL table
5.1.3.1.2. things to keep in mind when working with data frames
5.1.3.2. matrices
5.1.3.2.1. two-dimensional collection of data elements
5.2. Explore coding in R
5.2.1. Operator
5.2.1.1. a symbol that names the type of operation or calculation to be performed in a formula
5.2.1.2. Assigment operators
5.2.1.2.1. used to assign values to variables and vectors
5.2.1.3. Arithmetic operators
5.2.1.3.1. used to complete math calculations
5.2.1.4. Logical operators
5.2.1.4.1. return a logical data type such as TRUE or FALSE
5.2.1.5. AND operator “&”
5.2.1.6. OR operator “|”
5.2.1.7. NOT operator “!”
5.3. Learning about R packages
5.3.1. Packages
5.3.1.1. units of reproducible R code
5.3.1.2. include
5.3.1.2.1. reusable R functions
5.3.1.2.2. documentation about the functions
5.3.1.2.3. sample datasets
5.3.1.2.4. tests for checking your code
5.3.1.3. Base R
5.3.1.4. CRAN (comprehensive R archive network)
5.3.1.4.1. an online archive with R packages, source code, manuals, and documentation
5.4. Explore the tidyverse
5.4.1. Tidyverse
5.4.1.1. a collection of packages in R with a common design philosophy for data manipulation, exploration, and visualization
5.4.1.2. 8 core tidyverse packages
5.4.1.2.1. ggplot2
5.4.1.2.2. tidyr
5.4.1.2.3. readr
5.4.1.2.4. dplyr
5.4.1.2.5. tibble
5.4.1.2.6. purrr
5.4.1.2.7. stringr
5.4.1.2.8. forcats
5.4.2. Use pipes to nest code
5.4.2.1. nested
5.4.2.1.1. describes code that performs a particular function and is contained within code that performs a broader function
5.4.2.2. nested function
5.4.2.2.1. a function that is completely contained within another function
5.4.2.3. when using pipes
5.4.2.3.1. add the pipe operator at the end of each line of the piped operation, except the last one
5.4.2.3.2. check your code after you've programming your pipe
5.4.2.3.3. revisit piped operations to check for parts of your code to fix
5.4.3. Coding tips
5.4.3.1. An important aspect of any type of script or when you are coding, is to structure it for overall readability
5.4.3.2. An important aspect for readability and overall understanding of your code is using comments
5.4.3.3. Documentation will explain in depth exactly what your code is doing, why it was built, what is the purpose for it and any limitations
5.4.3.4. Building it for scalability as well as making it dynamic