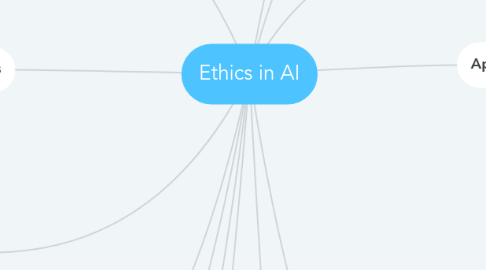
1. Societal impacts
1.1. Labour market
1.1.1. Working conditions
1.2. Income inequality
1.3. Reliance on technology
1.3.1. Inability to disconnect
1.3.2. Sacrificing privacy for connection
1.3.2.1. Inability to understand how own personal data is used
1.3.3. People's work and lives are retrofitted to serve machines. Ref: Prof Stephanie Dick
2. Other terms
2.1. Ethically aware algorithims
2.2. Human-centred machine learning
2.3. Inclusive machine learning and AI
2.4. Mindful AI
3. Human judgement in the data science progress
3.1. People choose where the data comes from, and why they think the selected examples are representative.
3.2. People define what determines success, and further, what evaluation metrics to use in measuring whether or not the model is working as intended.
3.3. People are affected by the results.
4. Biases
4.1. Cognitive
4.1.1. Social
4.2. Statistical
4.3. Algorithmic
5. When decisions are made by machines
5.1. Designing for transparency
5.2. Designing for a system of augmentation rather than replacement of decisions
5.3. Explainability of decisions is an important principles here
5.3.1. What kind of explanations are important?
5.3.1.1. Counterfactuals as explanations. Ref: Alan Turing Institute Brent Mittelstadt and Prof Sandra Watcher
6. Safety of AI algorithms
6.1. DeepFakes and disinformation
7. Explainability
7.1. Black box explanations
7.1.1. Explanations based on counterfactuals: Watcher et all 2019
7.1.1.1. Advantages: Accessible to the lay person and they can challenge the decision based on explanation
7.1.1.2. Limitation: Lacks interpretability - not enough for full transparency
8. Accuracy
8.1. Ethical ramifications
8.1.1. Precision versus recall trade-off - false positives and false negatives
9. What are others thinking/doing?
9.1. Google's AI principles
9.2. Vodafone
9.3. Facebook
9.4. MIT
9.5. DeepMind's ethical principles
10. Types of licences
10.1. Unrestrictive
10.2. Copyleft
11. Approaches to principles
11.1. Applying systems thinking
11.2. Do no harm or only do good?
11.3. Belmont human subject research principles
11.4. Thinking about regulation of algorithms
12. How machines and human interact
12.1. AI machines learn from humans in the case of reinforcement learning and deep learning - imitation learning
12.1.1. Deep learning is essentially an autonomous, self-teaching system in which you use existing data to train algorithms to find patterns and then use that to make predictions about new data.
12.1.2. Reinforcement learning is an autonomous, self-teaching system that essentially learns by trial and error. It performs actions with the aim of maximizing rewards, or in other words, it is learning by doing in order to achieve the best outcomes.
12.1.3. This is where we should focus our attention towards the dangers of society biases creeping into the algorithms
12.2. AI machines can also learn efficiently without learning from the behaviour of humans, but in the case of reinforcement learning they learn based on trial and error within a system of rules. Ref: Thore Graepal (DeepMind)
12.2.1. This seems to be an area of less ethical concern in terms of unintended consequences
12.3. Humans can learn from machines. You can use AI Models to examine complex human systems, e.g. the property market - and then can more easily identify points of sensitive intervention. Ref: Eric Beinhocker
12.4. Anthropormorphisms and Robomorphism
12.4.1. Robomorphism: We adapting ourselves to machines. We have to change our behaviour to fit with the technology. Retrofitting humans to technology, e.g. CVs, passport machines. Ref: Beth Singler.
12.5. Affective computing:
12.5.1. Affective robots and machines can teach people with autism how to read facial emotions. Ref: Maja Pantic, Imperial College London.
12.5.2. Affective machines can can read facial emotions can detect depression. Ref: Maja Pantic, Imperial College London
12.6. 'We need to nurture algorithms in the small way we nurture children'
12.7. Automation: What human abilities should be automated and which should not?
12.7.1. The way we automate and partition cognitive tasks is part of a long history of valuing and de-valuing forms of labour.
12.7.1.1. What groups are suffering as a result of the re-distribution of labour? What parts of labour being disciplined to become closer to machines? Ref: Stephanie Dick Penn
12.8. It's a symbiotic relationship
13. Types of AI
13.1. Machine learning
13.1.1. Supervised learning
13.1.2. Unsupervised learning
13.1.2.1. Deep learning
13.1.2.1.1. neural network
13.1.3. Reinforcement learning
13.1.3.1. Multi-Agent learning
13.2. Natural language processing
13.2.1. Word embeddings
13.2.1.1. Ethical issue: Man is to Computer Programmer as Woman is to Homemaker? Debiasing Word Embeddings