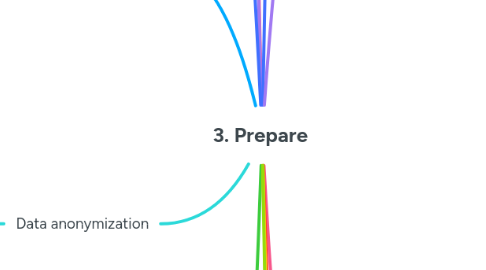
1. Unbiased and objective data
1.1. Data bias
1.1.1. type
1.1.1.1. Observer bias (experimenter bias/ research bias)
1.1.1.2. Interpretation bias
1.1.1.3. Confirmation bias
2. Idetify good data sources
2.1. ROCCC
2.1.1. Reliable
2.1.2. Original
2.1.3. Comprehensive
2.1.4. Current
2.1.5. Cited
3. Data ethics
3.1. Ethics
3.1.1. Well-founded standards of right and wrong that prescribe what humans ought to do, usually in terms of rights, obligations, benefits to society, fairness, or specific virtues
3.2. Data ethics
3.2.1. Well-founded standards of right and wrong that dictate how data is collected, shared, and used
3.2.1.1. aspects
3.2.1.1.1. ownership
3.2.1.1.2. transaction transparency
3.2.1.1.3. consent
3.2.1.1.4. currency
3.2.1.1.5. privacy
3.2.1.1.6. openness
3.3. Third-party data
3.3.1. is collected by an entity that doesn’t have a direct relationship with the data
3.4. Personal identifiable information (PII)
3.4.1. data that is reasonably likely to identify a person and make information known about them. It is important to keep this data safe
3.5. resources for open data
3.5.1. U.S. government data site U.S. Census Bureau Open Data Network Google Cloud Public Datasets Dataset Search
4. At the end of a day, data are people
5. Data anonymization
5.1. the process of protecting people's private or sensitive data by eliminating that kind of information
5.1.1. involves blanking, hashing, or masking personal information, often by using fixed-length codes to represent data columns, or hiding data with altered values.
5.2. list of data is often anomynized
5.2.1. Telephone numbers Names License plates and license numbers Social security numbers IP addresses Medical records Email addresses Photographs Account numbers
6. Databases
6.1. Metadata
6.1.1. data about data
6.1.2. is used in database management to help data analysts interpret the contents of the data within the database
6.1.3. 3 common types
6.1.3.1. descriptive
6.1.3.1.1. describes a piece of data and can be used to identify it at a later point in time
6.1.3.2. structural
6.1.3.2.1. indicates how a piece of data is organized and whether it is part of one, or more than one, data collection
6.1.3.3. administrative
6.1.3.3.1. indicates the technical source of a digital asset
6.1.4. benefits
6.1.4.1. reliability
6.1.4.2. consistency
6.1.5. metadata repositories
6.1.5.1. describe where the metadata came from and store that data in an accessible form with a common structure
6.1.5.2. can be kept in a physical location or a virtual environment—like data that exists in the cloud
6.1.6. is stored in a single, central location, and gives the company standardized information about all of its data
6.1.6.1. include information about where each system is located and where the datasets are located within those systems
6.1.6.2. decribes how all of the data is connected between the various systems
6.1.7. data governance
6.1.7.1. a process to ensure the formal management of a company's data assests
6.2. Relational database
6.2.1. a database that contains a series of related tables that can be connected via their relationships
6.2.1.1. primary key
6.2.1.1.1. an identifier that references a column in which each value is unique
6.2.1.2. foreign key
6.2.1.2.1. a field within a table that is a primary key in another table
7. Data collection
7.1. how data will be collected
7.1.1. interviews
7.1.2. observations
7.1.3. forms
7.1.4. questionnaires
7.1.5. surveys
7.1.6. cookies
7.2. choose data sources
7.2.1. first-party data
7.2.1.1. data collected by an individual or group using their own resources
7.2.2. second-party data
7.2.2.1. data collected by a group directly from its audience and then sold
7.2.3. third-party data
7.2.3.1. data collected from outside sources who did not collect it directly
7.3. decide what data to use
7.4. how much data to collect
7.4.1. population
7.4.1.1. all possible data values in a certain dataset
7.4.2. sample
7.4.2.1. a part of a population that is representative of the population
7.5. select the right data type
7.6. determine the time frame
7.7. data collection considerations
7.7.1. 1. Select the right data type
7.7.1.1. 2. Determine the timeframe
7.7.1.1.1. collect new data?
7.7.1.1.2. use existing data?
8. Data formats
8.1. continuous versus discrete data
8.1.1. continous data
8.1.1.1. data that is measured and can have almost any numeric value
8.1.1.1.1. temperature
8.1.1.1.2. height of kids in third grade classes
8.1.2. discrete data
8.1.2.1. data that is counted and has a limited number of values
8.1.2.1.1. tickets sold in the current month
8.1.2.1.2. maximum capacity allowed in a room
8.1.2.1.3. number of people who visit a hospital on a daily basis
8.2. nominal versus ordinal data
8.2.1. nominal data
8.2.1.1. a type of qualitative data that is categorized without a set order
8.2.1.1.1. new job applicant, existing applicant, internal applicant
8.2.1.1.2. first time customer, returning customer, regular customer
8.2.2. ordinal data
8.2.2.1. a type of qualitative data with a set order or scale
8.2.2.1.1. movie ratings
8.2.2.1.2. ranked-choice voting selections
8.2.2.1.3. satisfaction level measured in a survey (satisfied, neutral, dissatisfied)
8.3. internal versus external data
8.3.1. internal data
8.3.1.1. data that lives within a company's own systems
8.3.1.1.1. wages of employees across different business units track by HR
8.3.1.1.2. sales data by store location
8.3.1.1.3. product inventory levels across distribution centers
8.3.2. external data
8.3.2.1. data that lives and is generated outside of an organization
8.3.2.1.1. national average wages for the various positions throughout your organization
8.3.2.1.2. credit reports for customers of an auto dealership
8.4. qualitative versus quantitative
8.4.1. qualitative data
8.4.1.1. a subjective and explanatory measure of a quality or characteristic
8.4.1.1.1. favorite exercise
8.4.1.1.2. brand with best customer service
8.4.1.1.3. fashion preferences of young adults
8.4.2. quantitative data
8.4.2.1. a specific and objective measure, such as a number, quantity, or range
8.4.2.1.1. percentage of board certified doctors who are women
8.4.2.1.2. population size of elephants in Africa
8.5. structured versus unstructured data
8.5.1. structured data
8.5.1.1. data organized in a certain format such as rows and columns
8.5.1.1.1. expense reports
8.5.1.1.2. tax returns
8.5.2. unstructured data
8.5.2.1. data that is not organized in any easily identifiable manner
8.5.2.1.1. social media posts
8.5.2.1.2. emails
8.5.2.1.3. videos
8.6. primary versus secondary data
8.6.1. primary data
8.6.1.1. collected by a researcher from first-hand sources
8.6.1.1.1. data from an interview you conducted - data from a survey returned from 20 participants
8.6.1.1.2. data from questionnaires you got back from a group of workers
8.6.2. secondary data
8.6.2.1. gathered by other people or from other research
8.6.2.1.1. demographic data collected by a university
8.6.2.1.2. data you bought from a local data analytics firm's customer profiles
9. Data modeling
9.1. definition
9.1.1. a process of creating diagrams that visually represent how data is organized and structured
9.2. levels
9.2.1. conceptual - business concepts
9.2.1.1. gives a high-level view of the data structure, such as how data interacts across an organization
9.2.1.2. doesn't contain technical details
9.2.2. logical - data entities
9.2.2.1. focuses on the technical details of a database such as relationships, attributes, and entities
9.2.3. physical - physical tables
9.2.3.1. depicts how a database operates
9.3. techniques
9.3.1. Entity Relationship Diagram (ERD)
9.3.1.1. a visual way to understand the relationship between entities in the data model
9.3.2. Unified Modeling Language (UML)
9.3.2.1. very detailed diagrams that describe the structure of a system by showing the system's entities, attributes, operations, and their relationships
10. Data type
10.1. spreadsheets
10.1.1. number
10.1.2. text or string
10.1.2.1. a sequence of characters and punctuation that contains textual information
10.1.3. boolean
10.1.3.1. a data type with two possible values, TRUE or FALSE
10.2. wide and long data
10.2.1. wide data
10.2.1.1. data in which every data subject has a single row with multiple columns to hold the values of various attributes of the subject
10.2.2. long data
10.2.2.1. data in which each row is one time point per subject, so each subject will have data in multiple rows
11. Data transformation
11.1. goals
11.1.1. data organization
11.1.2. data compatibility
11.1.3. data migration
11.1.4. data merging
11.1.5. data enhancement
11.1.6. data comparison
12. Data interoperability
12.1. the ability of data systems and services to openly connect and share data
13. Organize and protect data
13.1. best practices when organizing data
13.1.1. naming conventions
13.1.1.1. consistent guidelines that describe the content, date, or version of a file in its name
13.1.1.1.1. SalesReport_2023_11_25_v02
13.1.1.2. use logical and descriptive names for your files to make them easier to find and use
13.1.2. foldering
13.1.2.1. organize your files into folders
13.1.3. archiving older files
13.1.4. align your naming and storage practices with your team
13.1.5. develop metadata practices
13.2. data security
13.2.1. protecting data from unauthorized access or corruption by adopting safety measures
13.2.1.1. Encryption
13.2.1.1.1. uses a unique algorithm to alter data and make it unusable by users and applications that don’t know the algorithm.
13.2.1.1.2. This algorithm is saved as a “key” which can be used to reverse the encryption; so if you have the key, you can still use the data in its original form.
13.2.1.2. Tokenization