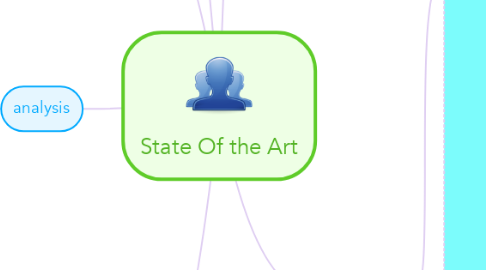
1. Other
1.1. robot acceptance
1.1.1. ivaldi2015towards
1.2. Cooperation Tasks
1.2.1. interaction primitives
1.2.1.1. denisalearning
1.2.1.2. ben2014interaction
1.2.2. evrard2009teaching
1.2.3. gribovskaya2011motion
1.3. master/ slave
1.3.1. Learning assistance by demo
1.3.2. transparency
1.3.3. adaptative prediction intention
1.3.4. reactive VS proactiv + DBN
1.4. walking
1.4.1. hu2016walking
2. Challenges
2.1. equilibrium
2.1.1. nori2015icub
2.1.2. romano2015whole
2.1.3. henzeapproach
2.1.4. nikolic2015task
2.2. Stiffness adaptation
2.2.1. berret2011stochastic
2.2.2. geravand2013human
2.2.3. permet de pas avoir à exercer de grosses forces
2.2.4. grip adaptation
2.3. Movement adaptation
2.4. Goal prediction
2.4.1. wang2012probabilistic
2.5. Multitask
2.5.1. hierarchical control/null space proj + passivity-based multi-contact balancing
2.5.2. task prio + compliance
3. humanoids robots
3.1. TORO
4. analysis
4.1. crossvalidation
5. Applications
5.1. To direct someone or to be directed
5.1.1. mansard2009versatile
5.1.2. Dongheui Lee
5.2. To lift someone or to be lifted
5.2.1. ikemoto2009physical
6. Tools
6.1. Machine Learning
6.1.1. assistive machines
6.1.2. Perception/action / apprentissage par imitation / LMS + SAW
6.1.2.1. Mon stage / mes cours
6.1.2.2. papiers de Gaussier
6.1.2.3. Grossenberg
6.1.3. Markov chains
6.1.4. Revoir RN type "ganglion de la base"
6.1.5. Gaussian mixture
6.1.5.1. rozo2014learning
6.1.6. kinesthesic learning
6.1.6.1. baxter
6.1.6.2. nikolic2015task
6.1.7. OIESGP
6.1.8. ProgrammingByDemo
6.1.8.1. Learning force and position constraints
6.1.8.1.1. rozo2014learning
6.1.9. learning assistance by demo
6.1.10. human motion predictors
6.1.10.1. geometric-based
6.1.10.1.1. BHMIP
6.1.10.1.2. Foka: Probabilistic Autonomous Robot Navigation in Dynamic Environments with Human Motion Prediction
6.1.10.2. place-dependent-based
6.1.10.2.1. Learning Motion Patterns of People for Compliant Robot Motion
6.1.10.2.2. vasquez: Growing Hidden Markov Models: An Incremental Tool for Learning and Predicting Human and Vehicle Motion
6.1.10.2.3. Chen: Adaptive human motion analysis and prediction
6.1.10.3. mixed approach
6.1.10.3.1. Ziebart: Planning-based prediction for pedestrians
6.1.10.3.2. Dee: Detecting inexplicable behaviour
6.1.11. ProMPs
6.1.12. DMP
6.1.12.1. ben2014interaction
6.1.13. HSMM
6.1.14. compliant movement primitives
6.1.14.1. denisalearning
6.1.15. Dnyamic Bayesian Network
6.1.15.1. baraglia2016
6.1.16. prediction of intent
6.1.17. BHMIP Bayesian H motion intenionality prediction
6.1.18. motion learning and adaptative mpedence
6.1.19. opti strategy for tuning the param of RBF networks model
6.1.20. facilitaing intention predicton for humans
6.2. Dynamics/kinematics
6.2.1. Forces computation
6.2.1.1. ivaldi2011computing
6.2.1.2. fumagalli2012force
6.2.1.3. romano2015whole
6.2.2. RNEA
6.2.2.1. Sciavicco : modeling
6.2.2.2. quoi d'autre ?
6.2.3. Enhanced Oriented Graph
6.2.3.1. 3 à lire
6.2.4. Classical Recursive Newton-Euler Algorithm
6.2.5. Zero-moment-point VS FRI
6.2.6. COM
6.3. Skin callibration
6.3.1. prete2011skin
6.4. iCub
6.4.1. natale2013icub