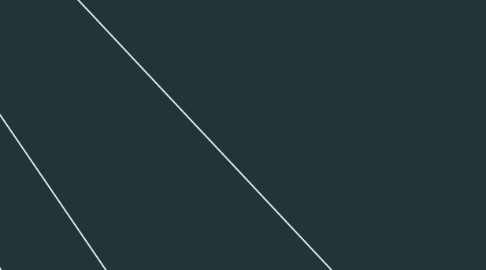
1. **Step 7** Evaluate and tune ML model(s)
1.1. Use generalization techniques to reduce overfitting.
1.2. Improve the structure of your datasets.
1.3. Consider the optimal complexity of your models
1.4. Set realistic performance requirements.
1.5. Use models in combination
1.6. Minimize loss/cost function (error)
1.6.1. Optimize model evaluation metrics and model parameters
1.7. Hyperparameter optimization
1.7.1. Grid search
1.7.2. Randomized search
1.7.3. Bayesian optimization
1.7.4. Genetic algorithms
2. **Step 8** Operationalize ML model(s)
2.1. ML model deployment
2.1.1. Training modes (can be applied to all deployment methods below)
2.1.1.1. Online
2.1.1.2. Offline
2.1.2. Deployment methods
2.1.2.1. Batch deployment
2.1.2.2. Real-time serving
2.1.2.2.1. API
2.1.2.2.2. Synchronous
2.1.2.2.3. Example online store recommendations
2.1.2.3. Streaming
2.1.2.3.1. Asyncrhonous
2.1.2.3.2. Message broker queue (middleware)
2.1.2.3.3. Example bacnk fraud detection reports
2.1.3. Deep Learning model deployment
2.1.3.1. Advanced AI accelerators and infrastructure
2.1.3.2. Distributed AI (DAI)
2.1.3.2.1. Learning agents
2.1.4. Infrastructure requirements
2.1.5. Stakeholder requirements
2.1.6. Deployment endpoints
2.1.6.1. API URI
2.1.6.2. Load balancing
2.1.6.3. Choice of best backend ML model, based on number of parameters in API request
2.1.7. Cloud services
2.1.7.1. Managed
2.1.7.1.1. Amazon SageMaker
2.1.7.1.2. Azure Machine Learning
2.1.7.1.3. Google Vertex AI
2.1.7.2. Unmanaged
2.1.8. Containers
2.1.8.1. Docker
2.2. Pipeline automation with MLOps
2.2.1. MLOps maturity levels
2.2.1.1. MLOps 0 (manual)
2.2.1.2. MLOps 1 (pipelines)
2.2.1.3. MLOps 2 (CI/CD/CT for pipelines)
2.2.2. scikit-learn pipeline module
2.2.3. Automation tasks via scripts and application software
2.2.3.1. Data collection
2.2.3.2. Data preparation
2.2.3.3. Model training
2.2.4. Pipeline triggers
2.2.4.1. On-demand
2.2.4.2. On a schedule
2.2.4.3. On availability of new data
2.2.4.4. On model drift or performance hit
2.2.5. CI/CD
2.2.5.1. Code repos
2.2.5.2. Unit tests
2.2.5.3. Multiple environments
2.2.5.3.1. Dev/Test
2.2.5.3.2. Staging/UAT
2.2.5.3.3. Prod
2.2.6. ML libraries and frameworks
2.2.6.1. Pytorch
2.2.6.2. Tensorflow
2.2.6.3. scikit-learn
2.2.6.4. Flask
2.2.6.5. Django
2.3. Integrate with existing ML systems
2.3.1. ML systems
2.3.2. Model APIs
2.3.2.1. API types
2.3.2.1.1. REST
2.3.3. ML system documentation
2.3.4. Design patterns
2.3.5. Ethical considerations for ML model operationalization
3. **Step 6** Train and test ML model(s)
3.1. Dataset splitting/sampling methods for cross-validation
3.1.1. Holdout
3.1.2. LOOCV
3.1.3. k-hold cross validation
3.1.4. Stratified cross validation
3.1.5. Training, validation and test data folds (subsets)
3.1.5.1. train_test_split() function
3.1.5.1.1. X_train
3.1.5.1.2. X_test
3.1.5.1.3. Y_train
3.1.5.1.4. Y_test
3.2. Regularization
3.2.1. Reduce overfitting
3.3. Select the right algorithm for the job (see previous step)
3.4. Avoid unnecessary complexity.
3.5. Model evaluation metrics
3.5.1. Learning curves
3.5.1.1. Understand if more training data will improve model or not (and until which point)
3.6. Prioritize model generalization
3.6.1. Check bias/variance
3.6.2. Check underfitting/overfitting
3.6.2.1. Sweet spot (good fit)
3.7. Minimize cost/error/loss function
3.8. Parameters
3.8.1. Identify model hyperparameters (external)
3.8.1.1. hyperparameter optimization
3.8.2. Model parameters (internal)
3.9. AutoML systems
3.9.1. https://cloud.google.com/automl
3.9.2. https://azure.microsoft.com/en-us/products/machine-learning/automatedml/
3.9.3. https://h2o.ai/platform/ai-cloud/make/h2o-driverless-ai/
4. **Step 5** Implement ML algorithm(s)
4.1. Regression (supervised)
4.1.1. Linear regression
4.1.1.1. Regularized linear regression
4.1.1.1.1. Iterative linear regression
4.1.2. Univariate forecasting
4.1.2.1. ARIMA
4.1.2.1.1. statsmodel.tsa.arima.model.ARIMA(y_train, order= (p, d, q), seasonal_order= (p, d, q, 12))
4.1.3. Multivariate forecasting
4.1.3.1. VAR
4.1.3.1.1. statsmodels.tsa.vector_ar.var_model.VAR(train_endo, train_exo)
4.1.4. Regression model evaluation (performance) metrics
4.1.4.1. Mean Squared Error (MSE)
4.1.4.2. Root Mean Squared Error (RMSE)
4.1.4.3. Mean Absolute Error (MAE)
4.1.4.4. R2 Coefficient of determination
4.1.5. Decision trees and random forests (regressors)
4.1.5.1. Decision trees
4.1.5.1.1. Classification and Regression Trees (CART) algorithm
4.1.5.1.2. C4.5
4.1.5.1.3. Pruning technique
4.1.5.1.4. Decision tree regressor contructor class example
4.1.5.2. Random forests (ensemble multiple decision trees)
4.1.5.2.1. Bagging (data sampling technique)
4.1.5.2.2. Out-of-bag error for ensemble learning (similar to cross-validation k-fold error estimation for non-ensemble learning)
4.1.5.2.3. sklearn.tree.RandomForestRegressor(n_estimators = 100, max_depth = 5)
4.1.5.3. Gradient boosting (ensemble algorithm with decision trees) - Alternative to random forests.
4.1.5.3.1. XGBoost library
4.1.6. SVM (regressors)
4.1.6.1. Hyperplane
4.1.6.2. Support Vector
4.1.6.3. Support-vector margin
4.1.6.4. Decision boundary
4.1.6.5. Hard-margin and soft-margin classification
4.1.6.6. Linear regression
4.1.6.7. Non-linear regression
4.1.6.7.1. kernel methods
4.2. Classification (supervised)
4.2.1. Logistic regression classification
4.2.1.1. Regularization and iteration
4.2.1.1.1. sklearn.linear_model.LogisticRegression(penalty = 'l2', C = 0.05, solver = 'sag')
4.2.2. K-NN classification
4.2.2.1. sklearn.neighbors.KNeighborsClassifier(n_neighbors = k)
4.2.3. Multi-label and multi-class classification
4.2.3.1. sklearn.linear_model.LogisticRegression(multi_class = 'multinomial’)
4.2.4. Classification model evaluation (performance) metrics
4.2.4.1. Confusion Matrices
4.2.4.2. Accuracy
4.2.4.3. Precision
4.2.4.4. Recall
4.2.4.5. F1 score
4.2.4.6. Specificity
4.2.4.7. ROC/AUC/PRC curves and thresholds
4.2.5. Decision trees (classifiers)
4.2.5.1. sklearn.tree.DecisionTreeClassifier(criterion = 'gini', max_depth = 5)
4.2.6. Random forests (classifiers)
4.2.6.1. sklearn.tree.RandomForestClassifier(n_estimators = 100, criterion = 'gini', max_depth = 5)
4.2.7. SVM (classifiers)
4.2.7.1. ε hyperparameter
4.2.7.2. Linear classification
4.2.7.3. Non-linear classification
4.2.7.3.1. Feature engineering to achieve linearization
4.2.7.3.2. The kernel trick and kernel methods
4.3. Clustering (unsupervised)
4.3.1. k-means clustering
4.3.1.1. k determination
4.3.1.1.1. sklearn.cluster.KMeans(n_clusters = k)
4.3.1.2. elbow point
4.3.1.2.1. yellowbrick.cluster.KElbowVisualizer(model, k = (1, 10))
4.3.1.3. WCSS/BCSS
4.3.1.4. Silhouette analysis
4.3.1.4.1. yellowbrick.cluster.SilhouetteVisualizer(model)
4.3.1.5. Dunn index and Davies-Bouldin index
4.3.2. hierarchical clustering
4.3.2.1. HAC (hierachical anglomerative clustering)
4.3.2.1.1. sklearn.cluster.AgglomerativeClustering(n_clusters = 3, linkage ='ward')
4.3.2.2. HDC (hierarchical divisive clustering)
4.3.2.3. cluster number determination
4.3.2.4. silhouette analysis
4.3.2.5. Dunn index
4.3.2.6. Dendrograms
4.4. Deep learning (ANN)
4.4.1. Regression
4.4.1.1. Forecasting
4.4.2. Classification
4.4.3. Clustering
4.4.4. Reinforcement learning
4.4.5. Feedforward neural networks (FNN) = single-direction
4.4.6. Multi-layer perceptrons (MLP) (FNN)
4.4.6.1. Perceptron algorithm
4.4.6.1.1. Binary classification
4.4.6.1.2. w vector of weights
4.4.6.1.3. b bias term (always 1)
4.4.6.1.4. Single-layer Single-label perceptrons (= 1x threshold logic unit (TLU), i.e. output neuron)
4.4.6.1.5. Single-layer Multi-label perceptrons (more than 1 TLU)
4.4.6.1.6. Multi-layer perceptrons
4.4.7. CNN (FNN)
4.4.7.1. Computer vision tasks
4.4.7.1.1. keras.models.Sequential()
4.4.7.2. Convolutional layers
4.4.7.2.1. CNN filters
4.4.7.3. Padding and stride
4.4.7.4. Pooling layers
4.4.7.5. CNN architecture
4.4.7.5.1. Input layer
4.4.7.6. Generative Adversarial Networks (GAN)
4.4.7.6.1. Generator neural network (inverse CNN)
4.4.7.6.2. Discriminator neural network (CNN)
4.4.7.6.3. GAN architecture
4.4.7.6.4. Mostly trained on image data
4.4.8. RNN (NOT FNN)
4.4.8.1. NLP tasks
4.4.8.1.1. keras.models.Sequential()
4.4.8.2. Multiple recurrent neurons in each RNN layer
4.4.8.3. Multiple RNN layers in time sequence (unrolling)
4.4.8.3.1. matrix of weighted input values (Wx)
4.4.8.3.2. matrix of weighted output values (Wy)
4.4.8.3.3. Activation function per RNN layer
4.4.8.4. Memory cells
4.4.8.4.1. Basic memory cells
4.4.8.4.2. Long short-term memory (LSTM) cells resolve shortcomings of basic memory cells
4.4.8.4.3. Gated Recurrent Unit (GRU) Cell = simplified LSTM cell
4.4.8.5. Training RNN
4.4.8.5.1. Backpropagation through time (BPTT)
4.5. LLM and generative AI
4.5.1. Transformer models
4.6. Ensemble learning
4.6.1. Ensemble multiple algorithms
4.6.2. Ensemble same algorithm on different data subset
4.6.3. Random forests
4.6.4. Gradient boosting (XGBoost library)
4.7. Semi-supervised learning
4.7.1. Self-supervised learning
5. **Step 9** Maintain ML model(s)
5.1. Security
5.1.1. RBAC
5.1.2. Hashing
5.1.3. Adversarial machine learning (defense methods)
5.1.3.1. Supply-chain attacks
5.1.3.2. ML model poisoning and evasion
5.1.3.3. MITRE Atlas matrix
5.1.4. Pipeline platform security
5.1.4.1. Data encryption
5.1.4.2. User access control
5.1.4.3. Intrusion detection / prevention
5.1.4.4. Security zoning
5.1.4.5. Penetration testing
5.1.4.6. Vulnerability scanning
5.1.4.7. Platform updates/patching
5.1.5. Pipeline job/task security
5.1.6. Access control
5.1.6.1. DAC
5.1.6.2. MAC
5.1.6.3. RBAC
5.1.6.4. Rules-based access control
5.1.6.5. Adaptive access control
5.1.6.6. User role management
5.1.6.6.1. Personas
5.1.6.7. User actions (permissions) management
5.2. Pipeline monitoring
5.2.1. Model/concept/data drifts
5.2.2. Logging
5.2.2.1. Logging events
5.2.2.2. Logging format
5.2.3. Continuous testing (part of Continuous Integration (CI))
5.2.4. Model/content/data drift
5.2.4.1. Model re-training (changing data only)
5.2.4.1.1. Pipeline triggers
5.2.4.1.2. Automatic re-training
5.3. Checkpoints and rollbacks
5.3.1. Disaster recovery
6. **Step 1 ** Define the business problem and desired outcome
6.1. Interviews with all stakeholders
6.2. Can the problem be solved manually or with conventional non-ML algorithms?
6.2.1. Time
6.2.2. Budget
6.2.3. Resources
6.2.4. Performance
6.3. Define problem inputs (independent parameters, input features) and desired outputs (output features)
6.4. Describe the problem in plain language
6.5. Define the type of problem
6.5.1. Regression
6.5.2. Classification
6.5.3. Clustering
6.5.4. Deep learning (ANN)
6.5.5. Combination of problems and algorithms
6.6. Project management
6.6.1. Time plan
6.6.2. Budget
6.6.3. Human resources and skills
6.6.4. Stakeholders and communication plan
6.6.5. PoC design
6.6.6. Ethical risks
7. **Step 2** Formulate the ML problem
7.1. Frame the business issue as an ML problem
7.2. Research datasets, data sourcesand algorithms Compare problem with other known problems
7.2.1. Data/ML communities
7.2.1.1. kaggle.com/
7.2.1.2. https://github.com/awesomedata/awesome-public-datasets
7.2.1.3. huggingface.co/
7.2.1.4. analyticsvidhya.com
7.2.1.5. mlcontests.com
7.2.1.6. unic.ac.cy/iff/research/forecasting/?ref=mlcontests
7.2.1.7. drivendata.org/
7.2.1.8. aicrowd.com/
7.2.1.9. codalab.org
7.2.1.10. alibabacloud.com/en/developer/ai-forward
7.2.1.11. signate.jp
7.2.1.12. eval.ai/
7.2.1.13. thinkonward.com/app/dashboard
7.2.1.14. data.gov.gr/
7.2.1.15. data.gov.cy/
7.2.2. Data sources
7.2.2.1. Data wareshouses
7.2.2.2. Data lakes
7.2.2.3. Data marts
7.2.2.4. Data hubs
7.2.3. Design of Experiment (DoE)
7.2.4. Probabilities of success
7.3. Suitability of learning modes
7.3.1. Reinforcement learning
7.3.2. Supervised learning
7.3.3. Unsupervised learning
7.3.4. Semi-supervised learning
7.4. Research suitability of common AI solutions
7.4.1. Prediction
7.4.2. Recommendation
7.4.3. Diagnosis
7.4.4. Natural language processing (NLP)
7.4.5. Computer vision
7.4.6. Robotics
7.5. Address responsible AI and ethical risks
8. **Step 3** Setup the ML infrastructure
8.1. On-premise, cloud or hybrid?
8.1.1. Physical computers
8.1.2. Virtual machines
8.1.3. Virtual Containers
8.1.4. Managed vs unmanaged cloud services
8.2. Development stack
8.2.1. Python
8.2.1.1. Python ML libraries, packages and modules
8.2.1.1.1. SciKit-Learn
8.2.1.1.2. scipy
8.2.1.1.3. numpy
8.2.1.1.4. keras
8.2.1.1.5. librosa
8.2.1.1.6. skimage
8.2.1.1.7. FFmpeg
8.2.1.1.8. spaCy
8.2.1.1.9. Pandas
8.2.1.1.10. Matplotlib
8.2.1.1.11. statsmodels
8.2.1.1.12. yellowbrick
8.2.2. R
8.2.3. Java/Javascript
8.2.4. Julia
8.3. ML notebook workspace
8.3.1. Jupyter
8.3.2. Kaggle notebooks
8.3.3. Google Colab
8.4. AI operations tools and platforms **(see step 8 ) **
8.5. AI maintenance tools and platforms **(see step 9)**
8.6. AI governance and observability tools
8.7. Setup AutoML for non-ML professionals
9. **Step 4** Work with data in ML workspace(s) - ETL
9.1. Collect and extract data sources and create datasets
9.1.1. Rows aka records aka Data examples
9.1.2. Columns aka fields aka Features
9.1.3. Values
9.1.4. Target variable/feature (label)
9.2. Create the ML notebook
9.2.1. Create Markdown cells
9.2.2. Create Python code cells
9.3. Load datasets and use dataframe objects to manipulate data
9.3.1. pandas.read_csv
9.3.2. pandas.read_excel
9.3.3. pandas.read_pickle
9.3.4. Relational DB/SQL
9.3.5. Other formats available
9.4. Data preparation/transformation/munging/wrangling
9.4.1. Handle corrupt/unusable data
9.4.2. Data quality issues
9.4.2.1. Irrelevant features
9.4.2.2. Non-representative data
9.4.2.3. Imbalanced data
9.4.2.4. Errors, outliers, and noise
9.4.3. Data quantity issues
9.4.3.1. Rule of thumb: At least 10 times as many records as features.
9.4.4. Correct data formats
9.4.5. Convert dates
9.4.6. Deduplicate data
9.4.7. Handle missing values
9.4.7.1. Fill with zeroes
9.4.7.2. Delete examples with missing data
9.4.7.3. Data imputation with statistical methods (e.g. mean value)
9.4.8. Examine descriptive statistics and data visualization
9.4.8.1. Count
9.4.8.2. Min/Max
9.4.8.3. Percentiles
9.4.8.4. Mean values
9.4.8.5. Standard deviation (STD)
9.4.8.6. Skewness plots
9.4.8.7. Gaussian (normalized) vs other data distributions
9.5. Feature engineering (aka data pre-processing)
9.5.1. Scale features
9.5.2. Encode features
9.5.2.1. Label
9.5.2.2. One-hot
9.5.2.3. Binary
9.5.2.4. Other methods
9.5.3. Discretize features
9.5.3.1. Binning
9.5.4. Split features
9.5.5. Reduce feature dimensionality
9.5.5.1. PCA algorithm
9.5.5.2. Random forests
9.5.5.3. Other algorithms
9.5.6. Normalization (for non-gaussianor other distribution)
9.5.6.1. sklearn.preprocessing.MinMaxScaler()
9.5.6.2. Same scale for all data ([0,1] or [-1, 1])
9.5.7. Standardization (for gaussian distribution of data)
9.5.7.1. sklearn.preprocessing.StandardScaler()
9.5.7.2. Standard score aka z-score
9.6. Transform unstructured data
9.6.1. Text
9.6.1.1. NLP data cleaning
9.6.1.1.1. Remove stop words
9.6.1.1.2. Case folding
9.6.1.1.3. Lower case
9.6.1.1.4. Punctuation
9.6.1.1.5. Word stemming
9.6.1.2. Bag of words
9.6.1.2.1. Word embedding methods
9.6.1.3. Tokenization
9.6.1.4. Stemming and Lemmatization
9.6.1.5. Python spaCY library
9.6.2. Images
9.6.2.1. Grayscale
9.6.2.2. Scaling
9.6.2.3. Re-shaping (Cropping, Contracting)
9.6.2.4. Perturbation (flip, rotate, offset, add noise)
9.6.2.5. Normalization and standardization (distribution values instead of absolute values)
9.6.2.6. Python skimage and matplotlib libraries
9.6.3. Video
9.6.3.1. Extract images from video (frame rate)
9.6.3.1.1. Python FFmpy library to extract frames
9.6.3.2. Apply image preprocessing techniques on extracted images
9.6.4. Audio
9.6.4.1. STFT (Fourier transformation)
9.6.4.2. Reduce sampling rate
9.6.4.3. Reduce bit depth/bit rate
9.6.4.4. Convert stereo to mono
9.6.4.5. Normalize/standardize values
9.6.4.6. Add or remove silence
9.6.4.7. Python librosa library