Copy of Machine Learning Algorithms Grouped by Similarity
par Orazio A
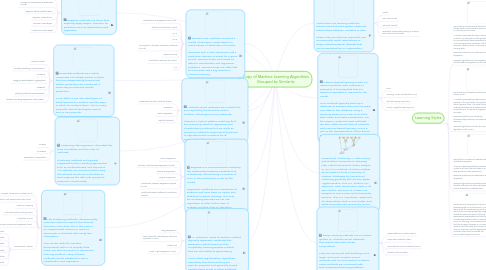
1. Bayesian methods are those that explicitly apply Bayes’ Theorem for problems such as classification and regression.
1.1. Naive Bayes
1.2. Average One Dependence Estimators (AODE)
1.3. Bayesian Belief Network (BBN)
1.4. Bayesian Network (BN)
1.5. Gaussian Naive Bayes
1.6. Multinomial Naive Bayes
2. Decision tree methods construct a model of decisions made based on actual values of attributes in the data. Decisions fork in tree structures until a prediction decision is made for a given record. Decision trees are trained on data for classification and regression problems. Decision trees are often fast and accurate and a big favorite in machine learning.
2.1. Classification & Regression Tree CART
2.2. Iterative Dichotomiser 3 (ID3)
2.3. C 4.5
2.4. C 5.0
2.5. Chi Squared Automatic Interaction Detection (CHAID)
2.6. Decision Stump
2.7. Conditional Decision Tree (CDT)
2.8. M 5
3. Ensemble methods are models composed of multiple weaker models that are independently trained and whose predictions are combined in some way to make the overall prediction. Much effort is put into what types of weak learners to combine and the ways in which to combine them. This is a very powerful class of techniques and as such is very popular.
3.1. Random Forest
3.2. Gradient Boosting Machines (GBM)
3.3. Boosting
3.4. Bagging (Bootstrapped Aggregation)
3.5. AdaBoost
3.6. Blending (Stacked Generalization)
3.7. Gradient Boosting Regression Trees (GBRT)
4. Artificial Neural Networks are models that are inspired by the structure and/or function of biological neural networks. They are a class of pattern matching that are commonly used for regression and classification problems but are really an enormous subfield comprised of hundreds of algorithms and variations for all manner of problem types.
4.1. Radial Basis Function Network (RBFN)
4.2. Perception
4.3. Back Propagation
4.4. Hopfield Network
5. Clustering, like regression, describes the class of problem and the class of methods. Clustering methods are typically organized by the modeling approaches such as centroid-based and hierarchal. All methods are concerned with using the inherent structures in the data to best organize the data into groups of maximum commonality.
5.1. k-means
5.2. k-medians
5.3. Expectation Maximization
6. Regression is concerned with modeling the relationship between variables that is iteratively refined using a measure of error in the predictions made by the model. Regression methods are a workhorse of statistics and have been co-opted into statistical machine learning. This may be confusing because we can use regression to refer to the class of problem and the class of algorithm. Really, regression is a process.
6.1. Linear Regression
6.2. Ordinary Least Squared Regression (OLSR)
6.3. Step-wise Regression
6.4. Logistic Regression
6.5. Multivariate Adaptive Regression Splines (MARS)
6.6. Locally Estimated Scatterplot Smoothing (LOESS)
7. Like clustering methods, dimensionality reduction seek and exploit the inherent structure in the data, but in this case in an unsupervised manner or order to summarize or describe data using less information. This can be useful to visualize dimensional data or to simplify data which can then be used in a supervised learning method. Many of these methods can be adapted for use in classification and regression.
7.1. Principal Component Analysis (PCA)
7.2. Partial Least Square Reduction (PLSR)
7.3. Sammon Mapping
7.4. Multi Dimensional Scaling (MDS)
7.5. Projection Pursuit
7.6. Principal Component Regression (PCR)
7.7. Discriminant Analysis
7.7.1. Linear
7.7.2. Regularized
7.7.3. Quadratic
7.7.4. Flexible
7.7.5. Mixture
7.7.6. Partial Least Squared