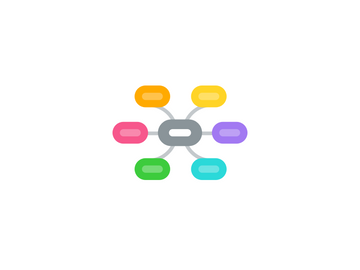
1. HETEROSCEDASTICITY
1.1. var(ut) =/ Ò2
1.2. Often arises in cross section studies but the variance may vary in accordance with time, qualitative characteristics, one or some of the explanatory variables
1.3. CONSEQUENCES
1.3.1. OLS estimates of coefficients are STILL CONSISTENT AND UNBIASED
1.3.2. no longer EFFICIENT
1.3.2.1. they do not have minimum variance
1.3.2.2. Standard errors and coefficient tests are incorrect
1.3.2.2.1. Standard procedure of statistical inference such as tests of significance are invalid
1.3.2.2.2. ???Example: if the variance of the errors is positively related to the square of an explanatory variable the OLS standard error will be too low.
1.3.2.2.3. The problem with heteroscedasticity is that it leads to incorrect standard errors, while the point estimates of the coefficients are unbiased.
1.4. DETECT
1.4.1. If a1 = a2 = … = am = 0 then &2t = a0 a constant and there is no evidence of heteroscedasticity
1.4.2. White's General Test-F version
1.4.2.1. Run an F-test
1.4.2.1.1. Unrestricted: Auxiliary regression
1.4.2.1.2. Restricted: Auxiliary run on a constant only
1.4.2.1.3. Joint Null: α2=0 and α3=0 and α4=0 and α5=0 and α6=0
1.4.3. Alternatively run LM (Lagrange-multiplier) test
1.4.3.1. TR2 ~ X2(m)
1.4.3.1.1. m is the number of regressors in auxiliary regression excluding the constant term
1.4.3.1.2. Joint Null: α2=0 and α3=0 and α4=0 and α5=0 and α6=0
1.4.3.1.3. Reject null (of no heteroscedasticity) if computed test statistic higher than critical χ2 at the chosen level of significance
1.5. Solutions
1.5.1. estimation in the presence of heteroscedasticity
1.5.1.1. Transform the data
1.5.1.1.1. Vt is homoscescedastic
1.5.1.2. The problem with heteroscedasticity is that it leads to incorrect standard errors, while the point estimates of the coefficients are unbiased.
1.5.1.2.1. White’s method of correcting the standard errors uses the residuals to obtain consistent estimates
1.5.2. Transform the data into logs to "pull in " extreme observations
1.5.3. Use heteroscedasticity-consistent standard error estimates that are common in econometric software packages.
2. GENERAL
2.1. TESTING THE RESTRICTED REGRESSION
2.1.1. F- test
2.1.1.1. test statistic = (RRSS-URSS) / URSS * (T-k)/m
2.1.1.2. where URSS = RSS from unrestricted regression RRSS = RSS from restricted regression m = number of restrictions T = number of observations k = number of regressors in unrestricted regression including constant (or total number of parameters to be estimated in unrestricted regression).
2.1.1.3. The value of the degrees of freedom parameters are m and (T-k) respectively (the order of the d.f. parameters is important).
2.1.1.3.1. column m, row (T-k).
2.1.1.3.2. not symmetrical
2.1.1.3.3. reject the null if the test statistic > critical F-value
2.1.2. it could be tested in the framework above for the F-test. Note that the two tests always give the same result since the t-distribution is just a special case of the F-distribution.
2.2. overall significance of the regression equation
2.2.1. F-test
2.2.1.1. Fk-1,n-k = R2/(K-1) / (1-R2)/(n-k)
3. E(UT)=/ 0
3.1. If a constant is included in the equation this assumption will never be violated
3.2. R2 can be negative without an intercept
4. Autocorrelation and Dynamic Models
4.1. definition
4.1.1. arises when the error terms in an equation are not independent but self, or Autocorrelation/Serial Correlation
4.1.1.1. E(ui , uj) =/ 0, for some i, j (i=/j)
4.1.1.1.1. 所有自己以外的,残余关系
4.1.2. Most often arises with time series data
4.1.2.1. In time series the effects of omitted variables etc tend to move cyclically and this will show up as correlated errors
4.1.3. why does serial correlation occur?
4.1.3.1. 1. Inertia
4.1.3.2. 2. Specification bias: omitted variables
4.1.3.3. 3. Specification bias: functional form
4.1.3.4. 4. Lags
4.1.3.5. 5. Manipulation of data
4.1.3.5.1. interpolation
4.1.3.5.2. data smoothness
4.1.4. AR(1)
4.1.4.1. where the errors in the current period are positively related to those in the PREVIOUS FIRST period. This is referred to as positive first order autoregressive error term or AR(1) for short
4.1.5. AR(1) can be negative and there is AR(2) or more
4.2. Detection of Autocorrelation
4.2.1. Residual plot against time
4.2.1.1. If serial correlation is a problem positive and negative values will tend to occur in groups
4.2.2. tests
4.2.2.1. for first order autocorrelation •Autocorrelogram, Durbin Watson Statistic
4.2.2.2. for higher order autocorrelation •Box Pierce, Ljung-Box and LM Tests
4.2.3. Durbin-Watson Statistic
4.2.3.1. d=~2(1-r1)
4.2.3.2. When there is no correlation between successive values of the residuals r1 = 0 (or ρ1=0) and d = 2
4.2.3.2.1. •If there is close to perfect positive correlation r1 -> 1 and d -> 0. •If there is close to perfect negative correlation r1 -> -1 and d -> 4.
4.2.3.3. H0: p = 0 and H1: p =/ 0.
4.2.3.3.1. •Reject H0 if d < dL (positive autocorrelation of the first order) •Reject H0 if d >4-dL (negative autocorrelation of the first order) •Do not reject H0 if dU<d<4-dU •Inconclusive: dL<d<dU or 4-dU<d<4-dL.
4.2.4. Breusch-Godfrey LM test
4.2.4.1. Regress the residuals (obtained from OLS estimates of the original model) on all explanatory variables in the model plus successive values of lagged residuals up to lag r.
4.2.4.2. This means that only (T-r) observations are available at the second stage.
4.2.4.3. X2 version
4.2.4.3.1. (T-r) R2 ~ X2(r)
4.2.4.3.2. r = lag periods, T is number of time observation
4.2.4.3.3. For r = 1, LM is an alternative to the Durbin-Watson test
4.2.4.4. F version
4.2.4.4.1. Unrestricted: Auxiliary regression with lags
4.2.4.4.2. Restricted: original regression
4.2.4.4.3. Joint Null: ρ1=0 and ρ2=0 ... ρr=0
4.3. Consequences of Ignoring Serial Correlation
4.3.1. still unbiased but they inefficient (standard errors are wrong, hence wrong inference)
4.3.1.1. to whether a variable is an important determinant of variations in y.
4.3.2. R2 could be inflated.
4.4. solutions
4.4.1. Think of omitted variables
4.4.1.1. missed
4.4.2. The dynamic structure in y has not been captured -- respecify the model, consider adding lags
4.4.2.1. lag
4.4.3. Wrong functional form
4.4.3.1. square or others
4.4.4. Autocorrelation and heteroscedasticity consistent estimators (eg Newey West)
4.5. LAGGED DEPENDENT VIABLES
4.5.1. E(yt,ut)=/ 0
4.5.1.1. Contemporaneous correlation between an explanatory variable (lagged Y) and an error term.
4.5.2. consequences
4.5.2.1. OLS estimates are biased and inconsistent
4.5.3. tests
4.5.3.1. The Durbin-Watson is NO longer valid
4.5.3.2. Durbin's h-test for first order correlation
4.5.3.2.1. method 1
4.5.3.3. LM test
4.5.3.3.1. method 2
5. Multicollinearity, Normality, Functional Form, Structural breaks, Information Criteria
5.1. multicollinearity
5.1.1. definition
5.1.1.1. Exact (perfect) multicollinearity
5.1.1.1.1. An exact linear relationship amongst the explanatory variables
5.1.1.1.2. Consequences: estimation is impossible
5.1.1.2. Multicollinearity (near perfect)
5.1.1.2.1. A high, but not perfect correlation among (some of) the explanatory variables
5.1.1.2.2. Estimation is possible
5.1.2. consequences of Multicollinearity
5.1.2.1. Small t-statistics
5.1.2.2. High R2
5.1.2.3. Significant F-statistic for zero slopes
5.1.2.4. Inference is unreliable
5.1.3. tests
5.1.3.1. cor(xi, xj)>0.8 i=/j
5.1.3.2. xi=0.6xj
5.1.4. Solutions to Multicollinearity
5.1.4.1. More data, although in practice this might mean waiting several years!
5.1.4.2. Re-arrange variables in the model
5.1.4.3. Use a priori information (empirical or theoretical) to drop one of the variables
5.1.4.4. Transform highly correlated variables into a ratio and include only the ratio
5.1.4.5. Use panel data
5.2. Normality
5.2.1. tests
5.2.1.1. Jarque-Berra test
5.2.1.1.1. An asymptotic, or large-sample test
5.2.1.1.2. JB= n*( S2/6 + (k-3)^2/24 )
5.2.1.1.3. Null hypothesis: the residuals are normally distributed
5.2.1.1.4. Follows chi-sqare distribution with 2 degrees of freedom
5.3. Functional Form
5.3.1. Assumption of linear functional form in CLRM
5.3.2. RESET (Regression Specification Error Test) to test for linearity by Ramsay
5.3.2.1. A general specification test
5.3.2.2. Add powers of the predicted values of Y from the initial regression
5.3.2.2.1. The squared fitted values capture complex dynamics among the original explanatory variables
5.3.2.3. F version
5.3.2.3.1. =RRSS-URSS/RRSS * T-k/m
5.3.2.3.2. TR2~X2(p-1)
5.3.2.4. a variant of F-test
5.3.2.4.1. (R2new-R2old)/number of new regressors / (1-R2new)/(n-number of parameters in new model)
5.3.2.5. mis-specified if significant
5.3.3. Solutions if Wrong Functional Form
5.3.3.1. A sample problem: outliers in small samples
5.3.3.1.1. increase sample size
5.3.3.2. Some models can still be estimated: transform into log
5.4. Structural Stability / Parameter Stability Test
5.4.1. Chow test
5.4.1.1. Split the sample into sub-periods and estimate the model for each of the subparts
5.4.1.2. F-distribution
5.4.1.2.1. RSS-(RSS1+RSS2) / (RSS1+RSS2) * N-2K / K
5.4.1.3. not stable if significant
5.4.2. Predictive Failure Test
5.4.2.1. RSS-RSS1/RSS1 * T1-k/T2
5.4.2.2. fail to predict if significant
5.5. Information Criteria
5.5.1. Metrics to assist in model selection in particular when several lags are included
5.5.2. embody two factors: the residual sum of squares and a penalty for the loss of degrees of freedom from adding and estimating additional coefficients.
5.5.3. The aim is to minimise the value of the information criteria