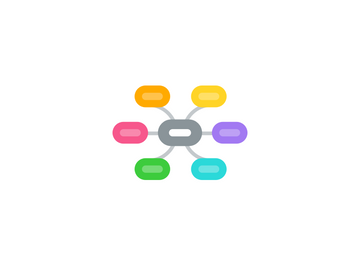
1. panel
1.1. Why Panel
1.1.1. Short time-series and need for MORE OBSERVATIONS (more degrees of freedom)
1.1.2. Investigate a WIDER RANGE OF QUESTIONS and tackle more complex problems than would be possible with pure time-series or pure cross section data alone
1.1.3. REDUCE MULTICOLLINEARITY, greater degrees of freedom and hence greater efficiency in the estimation
1.1.4. By structuring the model in an appropriate way we can REMOVE THE IMPACT of certain forms of OMITTED VARIABLE BIAS in regression results
1.2. the simple pooled model
1.2.1. assumptions
1.2.1.1. 1. All the usual OLS assumptions are not violated
1.2.1.2. 2. The intercept α is constant across all units j
1.2.1.3. 3. The impact of X1, X2... Xk on Y is constant across observations
1.2.1.4. 4. There is no interaction between Xs.
1.2.2. Autocorrelation in the residuals is possible
1.3. IF intercepts and slopes vary and we estimate a model with homogeneous intercepts or slopes we can get biased estimates.
1.3.1. approaches
1.3.1.1. Fixed effects model
1.3.1.1.1. The assumption is that slopes (partial impacts or betas) stay constant over time and across countries
1.3.1.1.2. methods
1.3.1.1.3. with Section Variant Intercepts
1.3.1.1.4. with Time Variant Intercepts
1.3.1.2. Random effects model
1.3.1.2.1. An alternative to fixed effects model, also known as the error components model (the error has two components)
1.3.1.2.2. As with fixed effects, the random effects model proposes different intercept terms of each cross-section unit and again these intercepts are constant over time.
1.3.1.2.3. But under the random effects model the intercepts for each cross section are assumed to arise from a common (and global) intercept α plus a random variable εj that varies cross sectionally but is constant over time.
1.3.1.2.4. Unlike the fixed effects model, there are no dummy variables to capture the heterogeneity in the cross-sectional dimension. Instead this occurs via the random ej term (which is constant through time).
1.3.1.2.5. For the estimation a generalised least squares (GLS) procedure is used since OLS will be inefficient due to cross-correlations between error terms for a given cross sectional unit at different points in time.
1.3.1.3. WHICH?
1.3.1.3.1. Hausman test