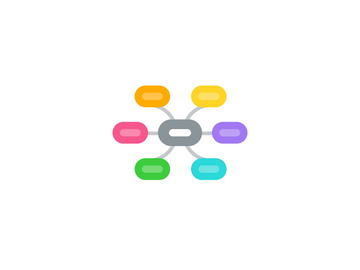
1. Big Data Landscape
1.1. Apps
1.1.1. Business Inteligence
1.1.1.1. IBM
1.1.1.2. Microsoft BI
1.1.1.3. Oracle Hyperion
1.1.1.4. SAP
1.1.2. Analytics and Visualization
1.1.2.1. 1010Data
1.1.2.2. Alpine
1.1.2.3. Opera
1.1.2.4. Palantir
1.1.2.5. SAS
1.1.2.6. Tableau
1.1.2.7. Teradata
1.1.3. Operational Intelligence
1.1.3.1. App Dynamics
1.1.3.2. Vitria
1.1.3.3. Splunk
1.1.3.4. Sumo Logic
1.2. Infrastructure
1.2.1. Analytics
1.2.1.1. Cloudera
1.2.1.2. Exasol
1.2.1.3. Hortonworks
1.2.1.4. Infobright
1.2.1.5. Kognitio
1.2.1.6. MapR
1.2.1.7. Netezza
1.2.1.8. Pivotal
1.2.1.9. Vertica
1.2.2. Structured DB
1.2.2.1. IBM DB2
1.2.2.2. SQL Server
1.2.2.3. MySQL
1.2.2.4. Oracle
1.2.2.5. Sybase
1.3. Technologies
1.3.1. Hbase
1.3.2. Cassandra
1.3.3. Hadoop
1.3.4. Hadoop Map Reduce
2. What is Big Data?
2.1. Definition
2.1.1. A collection of data sets so large and complex that it becomes difficult to process using on-hand database management tools or traditional data processing applications.
2.2. The True Value of Big Data
2.2.1. Big Data should be measured by the size of its impact, not by the amount of storage space or processing power that it consumes.
3. Your BigData Roadmap
3.1. 1. Identify the data sources you want to work
3.2. 2. Pull all the data together in one repository
3.3. 3. Have data in a workable state
3.4. 4. Query the data so you can get the information you need
3.5. 5. Display your data in visual form
3.6. Big Data Analytics
3.6.1. A. Getting What You Want
3.6.2. B. Interpreting What You Get
3.6.2.1. "No tool is more powerful for helping to interpret Big Data results than visualization"
4. Doing a Big Data Project
4.1. Setting up the project
4.1.1. 1. Define the outcome
4.1.2. 2. Identify the questions to answer
4.1.3. 3. Create policies around what data you use and how you use it
4.1.4. 4. Measure the value
4.1.5. 5. Identify the resources, including the people, data, hardware, and software, that you need to answer those questions
4.1.6. 6. Visualize the results