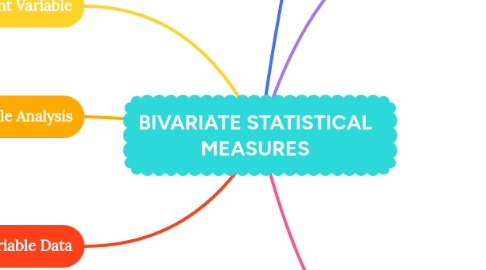
1. Bivariant Variable
1.1. Comes from the simultaneous observation of two variables (X, Y), in a sample of N individuals
2. Bivariable Analysis
2.1. We descibe and evaluate tha relationship between two variables or the behavior of one variable as a function of another
3. Bibariable Data
3.1. Are expressed through pairs of values
3.1.1. that is
3.1.1.1. Ordered pairs of type (a, b), when these types of values are obteined we refer to a two-dimensional distribution of the information we are analyzing
3.1.1.1.1. Each of the two variables can be qualitative or quantitative
4. Types of Correlations
4.1. Constant correlations
4.1.1. Contains a target element but no source element
4.2. Discovered correlations
4.2.1. It is a correlation that is propesed by executing the discovery function
4.3. Invalid correlations
4.3.1. The result of renaming the source or target models used in the correlation model
5. Regression analysis
5.1. is
5.1.1. An analysis technique that calculates the estimated relationship between a dependent variable and one or more explanatory variables
5.1.2. Possible to model the relationship between the chosen variables, as well as predict values based on the model
6. Regression
6.1. It is a statistical process that allows to analyze the relationship that exists between two or more variables, being one if them dependent to the rest of variables that we are using in our mathematical calculation
6.2. To apply an analysis of this type, we must use two types of variables
6.2.1. Dependent variables
6.2.1.1. Are those that we seek to study by means of statistical regression to understand how id adapts by modifying the independent variables
6.2.2. Independent variables
6.2.2.1. Are the factors that we consider to influence and directly affect the dependent variables under stufy
6.3. Types
6.3.1. Simple linear regression model
6.3.1.1. This is to study the effect of an independent variable on a single dependent variable of the first
6.3.2. Multiple linear regression model
6.3.2.1. It is a model that simply has more than one independent variable
6.3.3. Non-linear regression model
6.3.3.1. Allows us to obtain an approximation of the values of the dependet variable in a non-linear environment