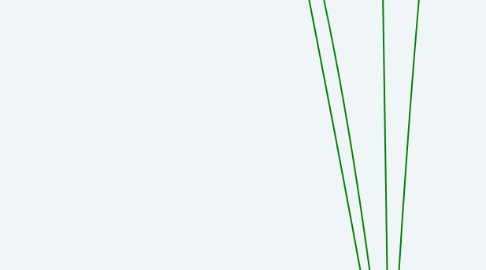
1. Determine the minimal sample size needed
1.1. Statistical test
1.2. Acceptable probability of type I error
1.3. Separate meaningful from trivial
1.4. Desired probability of rejecting the null hypothesis if population parameter is true
2. Sampling and Inferential Statistics
2.1. Sampling
2.1.1. Sample- a portion of a population (smaller)
2.1.2. Population- members of a well-defined class (larger)
2.1.3. Must be representative! If not, it is considered biased.
2.1.4. Steps
2.1.4.1. 1. Identify the target population
2.1.4.2. 2. Determine accessible population
2.1.4.3. 3. Select the sample
2.1.5. Probability Sampling
2.1.5.1. Everyone has an equal chance at being selected
2.1.5.1.1. Random Selection
2.1.5.2. Simple Random Sampling
2.1.5.2.1. 1. Define the population
2.1.5.2.2. 2. List all members
2.1.5.2.3. 3. Select the Sample
2.1.5.2.4. 4. Give each one an identification number
2.1.5.2.5. 5. Avoid all biases
2.1.5.3. Stratified Sampling
2.1.5.3.1. Study the differences in subgroups
2.1.5.3.2. Can be geographic
2.1.5.3.3. Strata that may differ in the characteristics being studied
2.1.5.3.4. May be geographic
2.1.5.3.5. Characteristics that may be of interest such as income, occupation, gender, age, education, etc.
2.1.6. Non-probability Sampling
2.1.6.1. Nonrandom procedures for selecting the members
2.1.6.2. Convenience Sampling
2.1.6.2.1. Using available cases for a study
2.1.6.2.2. Considered very weak
2.1.6.3. Purposive Sampling
2.1.6.3.1. AKA Judgment sampling
2.1.6.3.2. Sample elements are typical and representative
2.1.6.3.3. Can judgement be relied on?
2.1.6.4. Quota Sampling
2.1.6.4.1. Selecting typical cases from a diverse population
2.1.6.4.2. Based on known characteristics
2.1.7. Sizing
2.1.7.1. Size alone will not guarantee accuracy
2.1.7.2. Size is not as important as representativeness
2.1.8. Sampling Errors
2.1.8.1. Random samples can be expected to vary
2.1.8.2. Stems from observing only a sample instead of an entire population
2.1.8.3. Of the mean
2.1.8.3.1. Sampling errors is zero
2.1.8.3.2. Inverse function of sample size
2.1.8.3.3. Direct function of the standard deviation of the population
2.1.8.3.4. Distributed in a normal or near normal manner
2.2. Inferential Statistics
2.2.1. Making reasonable decisions with limited information
2.2.2. Null Hypothesis
2.2.2.1. No relationship between the variables
2.2.2.2. Nondirectional Test
2.2.2.2.1. Investigator is interested in either directio
2.2.2.2.2. Two-tailed
2.2.2.3. Directional test
2.2.2.3.1. Only one alternative to the null hypothesis
2.2.2.3.2. Parameter is greater or parameter is less
2.2.2.3.3. One-tailed
2.2.2.4. Power
2.2.2.4.1. ability to reject a null hypothesis when it is false
2.2.3. Type I Error
2.2.3.1. The rejection of a true null hypothesis
2.2.3.2. A "false alarm"
2.2.3.3. Think you have something, but really have nothing
2.2.3.4. Changes that are unwarranted
2.2.3.5. Considered more serious than a type II
2.2.3.6. Level of statistical significance
2.2.3.6.1. Null hypothesis is rejected
2.2.3.6.2. Investigator is willing to risk
2.2.3.7. All other variables that might affect the dependent variable are held constant
2.2.4. Type II Error
2.2.4.1. A "miss"
2.2.4.2. Investigator thinks there is nothing when there is actually something
2.2.4.3. Maintenance of status quo when a change is warranted
2.3. Determining the Sample Size
2.3.1. Specify the effect size
2.3.1.1. Difference between experimental and control groups divided by the standard deviation
2.3.1.2. The difference between the two groups divided by the estimated population standard deviation
2.3.1.3. Professionals in their field can specify an effect size
2.3.1.4. If effect size decreases, the number needed increases
2.3.2. Decide how precise you need to be
2.3.2.1. How large or small of an effect size you need
2.4. General Strategy of Statistical Tests
2.4.1. Compares what is observed with what we would expect to observe
2.4.2. Degrees of freedom
2.4.2.1. Number of observations free to vary around a constant parameter
2.4.3. Analysis of Variance
2.4.3.1. ANOVA
2.4.3.2. Analyze the data from a study with more than two groups
2.4.3.3. one-way ANOVA
2.4.3.3.1. One independent variable and one dependent variable
2.4.4. Multifactor Analysis of Variance
2.4.4.1. X influences Y in some circumstances, but not others
2.4.4.2. Is there an interaction?
2.4.4.3. Two independent variables are investigated= two way analysis of variance
2.4.5. Chi-Square Tests
2.4.5.1. Compare observed frequencies and expected frequencies
2.4.5.2. One-Variable
2.4.5.3. Two-Variable
2.4.5.3.1. Two independent variables
2.4.5.3.2. Multi-dimensional
2.4.5.4. Chi-square test of independence= Variables must be independent of the population
3. Experimental Research
3.1. Experiment characteristics
3.1.1. Independent variable is manipulated
3.1.2. The effect of manipulation of the IV on the DV is observed
3.2. Characteristics
3.2.1. Control
3.2.1.1. Arrange a situation in which the effect of a manipulated variable on a DV can be invetigated
3.2.2. Manipulation of the IV
3.2.2.1. Operation performed by experimenter to set up different treatment conditions
3.2.3. Observation
3.2.4. Measurement
3.3. Experimental Comparison
3.3.1. Begin with a hypothesis (prediction)
3.3.2. Must have experimental group and a control group
3.3.3. Comparison groups
3.3.3.1. comparing groups receiving different treatments
3.4. Experimental Design
3.4.1. Conceptual framework where experiment is conducted
3.4.2. Cause precedes effect in time
3.4.3. The cause occurs with the effect
3.4.4. alternative explanations can be ruled out
3.4.5. Differ in the number of IV that are manipulated
3.5. Validity
3.5.1. Are the relationship between two variables valid?
3.5.2. Internal Validity
3.5.2.1. Inferences about the changes in a DV and if it is changed by the IV
3.5.2.2. Threats
3.5.2.2.1. History
3.5.2.2.2. Maturation
3.5.2.2.3. Testing
3.5.2.2.4. Instrumentation
3.5.2.2.5. Statistical regression
3.5.2.2.6. Selection bias
3.5.2.2.7. Experimental mortality
3.5.2.2.8. Selection-maturation interaction
3.5.2.2.9. Experimenter effect
3.5.2.2.10. Subject effects
3.5.2.2.11. Diffusion
3.5.2.3. Random Assignment
3.5.2.3.1. The population has an equal chance of being assigned to the group
3.5.2.4. Randomized matching
3.5.2.4.1. Select pairs with identical or almost identical qualities and assign one member to group A and the other to group B
3.5.2.5. Homogeneous Selection
3.5.2.5.1. Selecting samples that are the same age, IQ score, etc.
3.5.2.6. Build variables into the design
3.5.2.7. Statistical control
3.5.2.7.1. ANCOVA
3.5.2.8. Use subjects as their own controls
3.5.2.8.1. assign the same subjects to all experimental conditions
3.5.2.9. Controlling situational differences
3.5.2.9.1. Hold them constant
3.5.2.9.2. Randomize them
3.5.2.9.3. Manipulate systematically and separately from the IV
3.5.2.9.4. Single-blind and double-blind experiment
3.5.2.10. Statistical Conclusion Validity
3.5.2.10.1. Use of statistics to infer whether a relationship between IV and DV is true cause-effect or solely chance
3.5.2.11. Construct Validity of Experiments
3.5.2.11.1. validity of inferences made about a construct based on...
3.5.2.11.2. Threats
3.5.2.11.3. Concerned with subjects chosen
3.5.2.11.4. 1. Start with clear people, setting, treatment, and outcome constructs
3.5.3. External Validity
3.5.3.1. Threats
3.5.3.1.1. Selection-treatment interaction
3.5.3.1.2. Setting-treatment interaction
3.5.3.1.3. Pretest-treatment interaction
3.5.3.1.4. Subject effects
3.5.3.1.5. Experimenter effects
3.5.3.2. Dealing with Threats
3.5.3.2.1. Randomly sample
3.5.3.2.2. Identify relevant characteristics and determine the impact of them
3.5.3.2.3. Do not use a pre-test
3.5.3.2.4. Arrange for a second control group to experience an existing interaction
3.5.3.2.5. Replicate the study in a new setting
4. Experimental Research Designs
4.1. X= independent variable
4.2. Y= dependent variable
4.3. S= subject or participant
4.4. E group= experimental group= given the IV treatment
4.5. C group= control group= different or no treatment
4.6. R= random assignment of subjects
4.7. Mr= subjects are matched and randomly assigned to comparison groups
4.8. Pre-experimental Designs
4.8.1. One-Group Pretest-Posttest Design
4.8.2. Static Group Comparison
4.8.3. Randomized subjects, Posttest only
4.8.4. Randomized matched subjects, posttest only
4.8.5. Randomized subjects, pre-test, posttest
4.8.6. Solomon three group design
4.8.7. Solomon four group design
4.9. Factorial designs
4.9.1. Simple factoral design
4.10. Quasi-experimental designs
4.10.1. Nonrandomized control group, pretest posttest
4.10.2. Counterbalanced
4.11. Time-Series Designs
4.11.1. One-group time-series
4.11.2. Control group time-series
4.12. Validity Problems
4.12.1. History
4.12.2. Maturation
4.12.3. Pretesting
4.12.4. Instrumentation
4.12.5. Statistical Regression
4.12.6. Differential Selection
4.12.7. Experimental mortality
4.12.8. Interaction of selection and other threats
4.12.9. Subject effects
4.12.10. Experimenter effect
4.12.11. Diffusion
4.13. ABAB Designs
4.13.1. A period of no treatment during which the behavior is measured
4.13.1.1. Such as the number of times a student leaves her seat
4.14. Multiple-baseline Design
4.14.1. Observations made on several participants, different target behaviors, different situations
4.14.2. Multiple-baseline across participants
4.14.2.1. Same intervention is applied to the same behavior to the same individual in the same settings
4.14.3. Multiple-baseline across behaviors
4.14.3.1. Applied to similar behaviors in the same setting
4.14.4. Multiple-baseline across settings
4.14.4.1. Same intervention is applied to the same behavior to the same individual in different settings
5. Descriptive Statistics
5.1. Analyze and interpret data
5.2. Communicate findings
5.3. Scales of Measurement
5.3.1. Nominal scale
5.3.1.1. Placing into categories
5.3.1.2. Numbers do not indicate value
5.3.2. Ordinal Scale
5.3.2.1. Ranks based on attributes
5.3.2.2. Numbers indicate order of the categories
5.3.3. Interval Scale
5.3.3.1. Places objects or events in order
5.3.3.2. Example is celsius and Fahrenheit thermometer
5.3.3.3. Marked in equal intervals
5.3.3.4. GPA is almost always interval data
5.3.4. Ratio Scale
5.3.4.1. Highest level of measurement scale
5.3.4.2. Has a true zero point and equal intervals
5.3.4.3. An example is a yardstick that contains inches and feet
5.4. Organizing Research Data
5.4.1. Frequency distributions
5.4.1.1. Highest to lowest
5.4.1.2. Systematic arrangement
5.4.2. Graphic Presentations
5.4.2.1. Histogram
5.4.2.1.1. Score points are horizontal, lowest on left, highest on right
5.4.2.1.2. Intervals are vertical
5.4.2.1.3. Dots above the center of each score
5.4.2.2. Frequency polygon
5.4.2.2.1. Connect adjacent dots, connect two ends to the base
5.4.2.2.2. Used to indicate data of a continuous nature
5.4.2.2.3. Resembles a line graph
5.5. Measures of Central Tendency
5.5.1. Mean
5.5.1.1. Most stable, precise, and frequently used
5.5.1.2. Sum of all scores divided by number of cases
5.5.1.3. The average
5.5.1.4. Side note: I'm getting flashbacks from middle school math classes! Lol!
5.5.2. Median
5.5.2.1. Ordinal
5.5.2.2. Halfway between lowest and highest points
5.5.2.3. Does not consider individual scores
5.5.2.4. Based on rank
5.5.3. Mode
5.5.3.1. Nominal
5.5.3.2. Occurs most frequently
5.5.3.3. I think mode is the easiest to understand
5.5.3.4. Inspection instead of computation
5.5.3.5. Least useful because it is unstable and there may be multiple modes
5.5.4. Comparison
5.5.4.1. Mean is the most precise
5.5.4.2. Mean is the most stable
5.5.4.3. Median is best indicator of typical performance
5.5.4.4. Mean is the best indicator of combined performance
5.5.5. Shapes of Distributions
5.5.5.1. Symmetrical when two halves are mirrored
5.5.5.1.1. Value of mean and median coincide
5.5.5.2. Skewed is pulled out to one end
5.5.5.2.1. Presence of an extreme score or outlier
5.5.5.2.2. Can be negative or positive
5.6. Measures of Variability
5.6.1. Range
5.6.1.1. Again, this reminds me of middle school math class. Lol.
5.6.1.2. Difference in the highest score and the lowest score
5.6.1.3. Unreliable because it's based only on two scores- highest and lowest
5.6.2. Variance and Standard Deviation
5.6.2.1. Most used
5.6.2.2. Based on deviation scores
5.6.2.3. Difference between raw score and mean
5.6.2.4. Interval/ratio statistics
5.6.2.5. This area really is overwhelming to me because I am not a numbers person.
5.6.2.6. Standard deviation
5.6.2.6.1. Same family as the mean
5.6.2.6.2. Educators prefer this index
5.6.2.6.3. Summarizes data in the same unit
5.6.2.6.4. Most frequently used
5.6.2.6.5. Spread, scatter, heterogeneity, dispersion, volatility
5.7. Measures of Relative Position
5.7.1. Indicate where a score falls in relation to the other scores
5.7.2. Z Score
5.7.2.1. Most widely used
5.7.2.2. Universal meaning
5.7.2.3. Mean is zero and standard deviation is one
5.7.2.4. Positive or negative differences between an individual score and the mean
5.7.3. T scores
5.7.3.1. Multiplying z scores by ten and adding fifty
5.7.3.2. Allows you to work with whole numbers and not decimals or negatives
5.7.4. A scores
5.7.4.1. Multiply z by desired standard deviation and add desired mean
5.7.5. Stanine Scores
5.7.5.1. 2Z+5
5.7.5.2. Avoid negatives and decimals
5.7.5.3. Often used in school systems for reporting standardized test scores
5.7.6. Percentile Rank
5.7.6.1. Percentage of scores that are equal to and fall below a certain point
5.7.6.2. Universal meaning and familiar concept
5.7.6.3. Cannot be added, subtracted, multiplied, or divided
5.8. The Normal Curve
5.8.1. When symmetrical, the mean, median, and mode are identical
5.8.2. Used to interpret standard scores
5.8.3. Bell-shaped
5.9. Correlation
5.9.1. Relationships between paired scores, can be positive or negative
5.9.2. Pearson r
5.9.2.1. No scatterplot
5.9.2.2. Direction and magnitude of relationship
5.9.2.3. Correlation does not indicate causation
5.9.3. Scatterplots
5.9.3.1. Dots represent individual's pairs of scores
5.9.3.2. Lower left to upper right=positive correlation
5.9.3.3. Upper left to lower right=negative correlation
5.9.3.4. Linear relationships
5.9.3.5. Scattered dots have a weak relationship
5.9.3.6. If the dots are curved, it is a curvilinear relationship
5.9.3.7. I think these are easier to understand than Pearson r.
5.10. Effect Size
5.10.1. Smith and Glass (1977)
5.10.2. Universal meaning to assess direction and strength
5.10.3. Determined the same way that z scores are
5.10.4. Independent of sample size
5.10.5. Cohen's d
5.10.5.1. Subtracting the mean of the control group from the mean of the experimental group and dividing the pooled standard deviation
5.11. Meta-analysis
5.11.1. Combines effect sizes with the independent and dependent variables
5.11.2. Provides an overall summary by calculating a weighted average
5.11.3. Gives a better relationship between among variables
5.11.4. Integrate findings of non-experimental studies