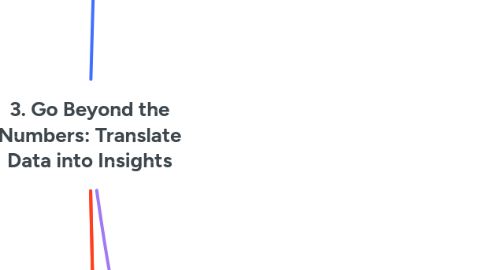
1. Find and share stories using data
1.1. Exploratory data analysis
1.1.1. The process of investigating, organizing, and analyzing datasets and summarizing their main characteristics, often employing data wrangling and visualization methods
1.1.2. Practices of EDA
1.1.2.1. Discovering
1.1.2.1.1. Data professionals familiarize themselves with the data so they can start conceptualzing how to use it
1.1.2.2. Structuring
1.1.2.2.1. The process of taking raw data and organizing or transforming it to be more easily visualized, explained, or modeled
1.1.2.3. Cleaning
1.1.2.3.1. The process of removing errors that may distort your data or make it less useful
1.1.2.4. Joining
1.1.2.4.1. The process of augmenting or adjusting data by adding values from other datasets
1.1.2.5. Validating
1.1.2.5.1. The process of verifying that the data is consistent and high quality
1.1.2.6. Presenting
1.1.2.6.1. Making your cleaned dataset or data visualizations available to others for analysis or further modeling
1.2. Data science and storytelling
1.2.1. "Storytelling is the way that your insights make it to other people and really make change"
1.2.2. "A really good way to tell a story with data is to think about categories of users, categories of devices, or categories of use cases"
1.3. Ethics of working with data
2. Explore raw data
2.1. Understanding raw data
2.1.1. Data sources
2.1.1.1. The location where data originates
2.1.1.2. Example
2.1.1.2.1. Report from a computer system
2.1.1.2.2. Selection from a large online database
2.1.1.2.3. Data table that has been manually entered
2.1.2. Data formats
2.1.2.1. Tabular files
2.1.2.2. XML files
2.1.2.3. CSV files
2.1.2.4. Excel files
2.1.2.5. DB files
2.1.2.6. JSON files
2.1.3. Data types
2.1.3.1. First-party data
2.1.3.1.1. Data that was gathered from inside your own organization
2.1.3.2. Second-party data
2.1.3.2.1. Data that was gathered outside your organization but directly from the original source
2.1.3.3. Third-party data
2.1.3.3.1. Data gathered outside your organization and aggregated
2.1.3.4. Other types of data
2.1.3.4.1. Geographic
2.1.3.4.2. Demographic
2.1.3.4.3. Numeric
2.1.3.4.4. Time-based
2.1.3.4.5. Financial
2.1.3.4.6. Qualitative
2.1.4. Python functions
2.1.4.1. df.head()
2.1.4.2. Info( )
2.1.4.2.1. Gives the total number and data types of individual entries. Keep in mind that data types are called Dtypes in pandas
2.1.5. Questions during the discovering process
2.1.5.1. How can I break this data into smaller groups so I can understand it better?
2.1.5.2. How can I prove my hypothesis?
2.1.5.3. In its current form, can this data give me the answers I need?
2.1.6. Organize or alter data
2.1.6.1. Regroup entries into months/years or age ranges
2.1.6.2. Group customer ages into age ranges
2.1.6.3. Combine or split data columns
2.1.6.4. Change date formats or time zones
2.1.7. Understand data format
2.1.7.1. Import statements
2.1.7.1.1. import matplotlib.pyplot as plt: This imports the "plotting" part of the matplotlib library, which is like a collection of tools for **drawing graphs. **
2.1.7.1.2. import pandas as pd: This imports the Pandas library, which is like a **super spreadsheet for organizing and analyzing data.**
2.1.7.1.3. import seaborn as sns: Seaborn helps us **make our graphs prettier and easier to understand. **
2.1.7.2. Key functions
2.1.7.2.1. **pd.to_datetime():** This function is like a language translator. It takes a column of dates written as text ("2023-10-26") and converts them into a format Python understands as dates, allowing for date-based calculations.
2.1.7.2.2. **strftime():** This is like a date formatter. It takes a date and lets you display it in different ways (e.g., "October 26, 2023" or "2023-10-26").
2.1.7.2.3. plt.bar(): This function is our bar chart builder. We give it data for the x-axis (e.g., weeks) and y-axis (e.g., number of lightning strikes), and it draws the chart.
2.1.7.2.4. plt.xlabel(), plt.ylabel(), plt.title(): These functions are like adding labels to our chart to make it clear what it represents.
2.1.7.2.5. plt.xticks(): This helps us format the labels on the x-axis, rotating them if they're too crowded.
2.1.7.2.6. plt.show(): This is the "reveal" button. It takes all our chart setup and displays the final result.
2.1.8. Create structure from raw data
2.1.8.1. Sorting
2.1.8.1.1. The process of arranging data into meaningful order for analysis
2.1.8.2. Extracting
2.1.8.2.1. The process of retrieving data from a dataset or source for further processing
2.1.8.3. Filtering
2.1.8.3.1. The process of selecting a smaller part of your dataset based on specified parameters and using it for viewing or analysis
2.1.8.4. Slicing
2.1.8.4.1. A method for breaking information down into smaller parts to facilitate efficient examination and analysis from different viewpoints
2.1.8.5. Grouping
2.1.8.5.1. Aggregating individual observations of a variable into groups
2.1.8.6. Merging
2.1.8.6.1. Method to combine two different data frames along a specified starting column
2.1.8.7. EDA structuring with Python
2.1.8.7.1. **A box plot** is a data visualization that depicts the locality, spread, and skew of groups of values within quartiles. Box plots provide information on the variability and dispersion of data by depicting how the values in the data are spread out.