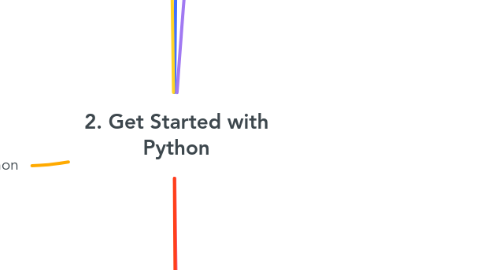
1. Hello, Python
1.1. programming languages
1.1.1. the words and symbols we use to write instructions for computers to follow
1.1.2. computer
1.1.2.1. computer hardware
1.1.2.2. transitor
1.1.2.2.1. controls the flow of electricity through a circuit
1.1.2.2.2. exists in two stages: on or off
1.1.3. library
1.1.4. Python versus other programming languages
1.1.4.1. Five considerations of programming languages
1.1.4.1.1. Speed
1.1.4.1.2. Approachability
1.1.4.1.3. Variables
1.1.4.1.4. Data science focus
1.1.4.1.5. Programming paradigm
1.1.4.2. Programming language comparisons
1.1.4.2.1. Speed
1.1.4.2.2. Approachability
1.1.4.2.3. Variable
1.1.4.2.4. Data science focus
1.1.4.2.5. Programming paradigm
1.2. Jupiter Notebooks
1.2.1. an open-source web application for creating and sharing documents containing live code, mathematical formulas, visualizations, and text
1.2.1.1. Why Jupyter Notebook
1.2.1.1.1. Modular/interactive computing
1.2.1.1.2. Integration of code and documentation
1.2.1.1.3. Support for multiple languages
1.2.1.1.4. Data exploration and analysis
1.2.1.1.5. Cloud-based services
1.2.1.1.6. Libraries and extensions
1.2.2. Cells
1.2.2.1. the modular code input/output fields into which Jupiter Notebooks are partitioned
1.3. Object-oriented programming
1.3.1. A programming system that is based around objects, which can contain both data and code that manipulates that data
1.3.2. Object
1.3.2.1. An instance of a class; a fundamental building block of Python
1.3.3. Class
1.3.3.1. A class is an object's data type that bundles data and functionality together
1.3.3.1.1. core Python classes
1.3.4. Method
1.3.4.1. A function that belongs to a class and typically performs an action or operation
1.3.5. Dot Notation
1.3.5.1. How to access the methods and attributes that belong to an instance of a class
1.3.6. Attribute
1.3.6.1. A value associated with an object or class which is referenced by name using dot notation
1.3.7. distinction between Attribute and Method
1.3.7.1. Attributes
1.3.7.1.1. characteristics of the object
1.3.7.2. Methods
1.3.7.2.1. actions or operations
1.4. Variables and data types
1.4.1. Variable
1.4.1.1. a container with a label on it, a container is a separate thing from whatever it contains
1.4.1.1.1. variable name
1.4.2. Data type
1.4.2.1. An attribute that describes a piece of data based on its values, its programming language, or the operations it can perform
1.4.3. Variable algorithm questions
1.4.3.1. What's the variable's name?
1.4.3.2. What's the variable's type?
1.4.3.3. What's the variable's starting value?
1.4.4. Assignment
1.4.4.1. The process of storing a value in a variable
1.4.5. Expression
1.4.5.1. A combination of numbers, symbols, or other variables that produce a result when evaluated
1.4.6. Dynamic typing
1.4.6.1. Variables can point to objects of any data type
1.4.7. Operator
1.4.7.1. Symbols that perform operations on objects and values
1.4.8. Function
1.4.8.1. A group of related statements to perform a task and return a value
1.4.9. Conditional statements
1.4.9.1. Sections of code that direct program execution based on specified conditions
1.4.10. difference between syntax and semantics in Python
1.4.10.1. syntax is about writing code correctly
1.4.10.2. semantics is about making sure your code does what you intend it to do
1.5. Data types
1.5.1. String
1.5.1.1. A sequence of characters and punctuation that contains textual information
1.5.2. Integer
1.5.2.1. A data type used to represent whole numbers without fractions
1.5.3. Float
1.5.3.1. A data type that represent numbers that contain decimals
1.5.4. Boolean
1.5.4.1. A data type that has only two possible values, usually true or false
1.5.5. Immutable data type
1.5.5.1. A data type in which the values can never be altered or updated
1.5.6. Convert data in Python
1.5.6.1. Implicit conversion
1.5.6.1.1. automatically converts one data type to another without user involvement
1.5.6.2. Explicit conversion
1.5.6.2.1. Users convert the data type of an object to a required data type
2. Functions and conditional statements
2.1. Functions
2.1.1. Tips for beginners
2.1.1.1. Start practicing to truly understand coding
2.1.1.2. Learn to debug
2.1.1.3. Start compartmentalizing to accelerate your learning journey
2.1.2. Definition
2.1.2.1. A body of reusable code for performing specific processes or tasks
2.1.2.2. def
2.1.2.2.1. a keyword that defines a function at the start of the function block
2.1.2.3. return
2.1.2.3.1. a reserved keyword in Python that makes a function produce new results, which are saved for later use
2.1.2.4. return vs. print
2.1.2.4.1. return
2.1.2.4.2. print
2.1.2.5. functions vs. methods
2.1.2.5.1. methods
2.1.2.5.2. functions
2.1.2.5.3. **Methods** **are functions with objects **(classes), while functions stand alone and can be applied more broadly.
2.1.3. Function syntax
2.1.3.1. Begin with the def keyword followed by the function’s name, then put its parameters/arguments in parentheses, ending with a colon.
2.1.3.2. For important functions or functions whose purposes or operations are not very obvious, include a docstring. Write the docstring between three opening and closing quotation marks.
2.1.3.3. Write the body of the function.
2.1.3.4. Finally, use a return statement to return a value or a print statement to print something to the console and complete the function.
2.2. Write clean code
2.2.1. Reusability
2.2.1.1. Defining code once and using it many times without having to rewrite it
2.2.2. Modularity
2.2.2.1. The ability to write code in separate components that work together and that can be reused for other programs
2.2.3. Refactoring
2.2.3.1. The process of restructuring code while maintaining its original functionality
2.2.4. Self-documenting code
2.2.4.1. Code written in a way that is readable and makes its purpose clear
2.3. Commenting
2.3.1. Algorithm
2.3.1.1. A set of instructions for solving a problem or accomplishing a task
2.3.2. Docstring
2.3.2.1. A string at the beginning of a function's body, that summarizes the function's behavior, and explains its arguments and return values
2.4. Operators
2.4.1. Comparators
2.4.1.1. Operators that compare two values and produce Boolean values (True/False)
2.4.2. Logical operators
2.4.2.1. Operators that connect multiple statements together and perform more complex comparisons
2.4.2.1.1. and
2.4.2.1.2. or
2.4.2.1.3. not
2.4.3. Arithmetic operators
2.4.3.1. Modulo
2.4.3.1.1. An operator that returns the remainder when one number is divided by another
2.5. Conditional statements
2.5.1. Branching
2.5.1.1. The ability of a program to alter its execution sequence
2.5.2. if
2.5.2.1. A reserved keyword that sets up a condition in Python
2.5.3. else
2.5.3.1. A reserved keyword that executes when preceding conditions evaluate as False
2.5.4. elif
2.5.4.1. A reserved keyword that executes subsequent conditions when the previous conditions are not True
3. Loops and strings
3.1. Loops
3.1.1. Loop
3.1.1.1. A block of code used to carry out iterations
3.1.1.1.1. while loop
3.1.1.1.2. for loop
3.1.1.1.3. difference between for loop and while loop
3.1.1.1.4. break
3.1.1.1.5. continue
3.1.2. Iteration
3.1.2.1. The repeated execution of a set of statements, where on iteration is the single execution of a block of code
3.1.3. Iterable
3.1.3.1. An object that's looped, or iterated, over
3.2. Strings
3.2.1. Concatenate
3.2.1.1. To link or join together
3.2.2. Escape character
3.2.2.1. A character that changes the typical behavior of the characters that follow it
3.2.2.1.1. backslash ( \ )
3.2.2.1.2. backslash-N ( \n )
3.2.3. String slicing
3.2.3.1. Indexing
3.2.3.1.1. A way to refer to the individual items within an iterable by their relative position
3.2.3.1.2. Can be used on: strings, lists, tuples, most other iterable data types
3.2.3.1.3. index( ) : a string method that outputs the index number of a character in a string
3.2.3.2. String slice
3.2.3.2.1. A portion of a string, also known as a substring, that can contain more than one character
3.2.3.3. To check whether or not a substring is contained in a string, use the keyword "in"
3.2.3.4. In Python, the first element of any sequence has **an index of zero**
3.2.4. Format strings
3.2.4.1. format ( )
3.2.4.1.1. Formats and inserts specific substrings into a designated places
3.2.4.1.2. The format() function can also insert values into braces using explicitly assigned keyword names, which allow you to mix up the order of the function’s arguments without changing the order of their insertion into the final string
3.2.4.2. Literal string interpolation (f-strings)
3.2.4.2.1. when using Python version 3.6+ is literal string interpolation, also known as f-strings. F-strings further minimize the syntax required to embed expressions into strings. They’re called f-strings because the expressions always begin with f (or F—they’re the same).
3.2.4.3. Float formatting options
3.2.4.3.1. The float variable is what’s being formatted
3.2.4.3.2. A colon **(:)** separates what’s being formatted from the syntax used to format it
3.2.4.3.3. **. number** indicates the desired precision
3.2.4.3.4. A letter indicates the presentation type
3.2.4.4. String methods
3.2.4.4.1. str.count(sub[, start[, end]])
3.2.4.4.2. str.find(sub)
3.2.4.4.3. str.join()
3.2.4.4.4. str.partition(sep)
3.2.4.4.5. str.replace(old, new[, count])
3.2.4.4.6. str.split([sep])
3.2.4.5. format() method vs. f-strings
3.2.4.5.1. format() method
3.2.4.5.2. f-strings
3.2.4.6. Regular expressions
3.2.4.6.1. Defining the Pattern
3.2.4.6.2. Using the re Module
3.3. You're supposed to develop the skills to be able to approach a problem in an analytical mindset
4. Case study
4.1. Automatidata: Inspect and analyze data
4.1.1. Understand the situation
4.1.2. Understand the data
4.1.3. Understand the variables
5. Write clean code
6. Data structures in Python
6.1. Lists and tuples
6.1.1. List
6.1.1.1. A data structure that helps store and manipulate an ordered collection of items
6.1.1.1.1. Lists are **mutable,** which means that you can change their contents after they are created
6.1.1.1.2. Lists can be combined using the addition operator (+) and the multiplication operator (*)
6.1.1.2. Sequence
6.1.1.2.1. A positionally ordered collection of items
6.1.1.3. Mutability
6.1.1.3.1. The ability to change the internal state of a data structure
6.1.1.4. Immutability
6.1.1.4.1. A data structure or element's values can never be altered or updated
6.1.1.5. Method
6.1.1.5.1. **append ( )** : adds an element to the end of a list
6.1.1.5.2. **insert ( ) **: function that takes an index as the first parameter and an element as the second parameter, then inserts the element into a list at the given index
6.1.1.5.3. **remove ( ) **: removes an element from a list
6.1.1.5.4. **pop ( )** : extracts an element from a list by removing it at a given index. If no index is specified, pop() removes and returns the last item in the list
6.1.1.5.5. **index ( ) **: Return the index of the first occurrence of an item in the list
6.1.1.5.6. **count ( ) :** Return the number of times an item occurs in the list
6.1.1.5.7. **sort ( ) :** Return the number of times an item occurs in the list
6.1.2. Tuple
6.1.2.1. Tuples are expressed with parentheses or the tuple ( ) function
6.1.2.2. tuple ( )
6.1.2.2.1. Function that transforms input into tuples
6.1.2.3. unpacking a tuple
6.1.2.3.1. Use a for Loop to Loop over the list
6.1.3. Compare lists, strings, and tuples
6.1.3.1. Syntax/instantiation
6.1.3.1.1. **Strings:** Declared using single quotes ('...'), double quotes ("..."), or triple quotes ('''...''' or """...""")
6.1.3.1.2. **Lists:** Defined using square brackets ([...]) and elements are separated by commas.
6.1.3.1.3. **Tuples:** Created using parentheses ((...)) with elements separated by commas.
6.1.3.2. Mutability
6.1.3.2.1. **Strings:** Immutable
6.1.3.2.2. **Lists:** Mutable
6.1.3.2.3. **Tuples:** Immutable
6.1.3.3. Use cases
6.1.3.3.1. **Strings:** Primarily used for working with text data
6.1.3.3.2. **Lists:** Ideal for storing collections of items that you need to modify, iterate over, or search
6.1.3.3.3. **Tuples:** Suitable for representing fixed collections of items, often used for returning multiple values from a function or as dictionary keys.
6.1.3.4. Content
6.1.3.4.1. **Strings:** Contain any character—letters, numbers, punctuation marks, spaces
6.1.3.4.2. **Lists:** Contain any data type, and in any combination
6.1.3.4.3. **Tuples:** Contain any data type, and in any combination
6.1.4. When choose tuples instead of lists
6.1.4.1. Need a fixed collection of items
6.1.4.2. Order matters, and the elements should always stay together
6.1.4.3. Ensure data integrity and prevent accidental modifications
6.1.5. Initialization
6.1.5.1. **Initializing an empty list** gives the program a place to store values as they are calculated in the loop. Without it, you’d have no container for the results, which would cause an error or prevent the accumulation of values.
6.1.6. Functions
6.1.6.1. zip ( )
6.1.6.1.1. works with as many iterable objects as you need.
6.1.6.1.2. If the input iterables have different lengths, the resulting iterator will be the same length as the shortest input.
6.1.6.2. unzipping
6.1.6.2.1. unzip an object with the * operator
6.1.6.3. enumerate ( )
6.1.6.3.1. Adds a counter to an iterable: It takes an iterable object (like a list, tuple, or string) and returns an iterator that produces pairs of (index, element) for each item in the iterable.
6.1.6.3.2. **Default Starting Index:** The default starting index is 0, but you can customize it by passing a second argument to enumerate(). For example, enumerate(fruits, 1) would start the index at 1.
6.1.6.3.3. **Returns an Iterator:** Remember that enumerate() returns an iterator, so you'll typically use it within a loop or convert it to a list/tuple if you need to store the results.
6.1.7. List comprehensions
6.1.7.1. Benefits
6.1.7.1.1. **Concise:** It allows you to write code that is more compact and readable, especially for simple list manipulations.
6.1.7.1.2. **Efficient:** List comprehensions are often faster than traditional for loops, especially for larger datasets
6.1.7.2. **Basic structure:** new_list = [expression for item in iterable if condition]
6.1.7.2.1. **expression** refers to an operation or what you want to do with each element in the iterable sequence.
6.1.7.2.2. **element** is the variable name that you assign to represent each item in the iterable sequence.
6.1.7.2.3. **iterable** is the iterable sequence.
6.1.7.2.4. **condition** is any expression that evaluates to True or False. This element is optional and is used to filter elements of the iterable sequence.
6.2. Dictionaries and sets
6.2.1. Dictionary
6.2.1.1. A mutable data structure that consists of a collection of key-value pairs
6.2.1.1.1. Create a dictionary
6.2.1.2. Immutable keys
6.2.1.2.1. Integers
6.2.1.2.2. Floats
6.2.1.2.3. Tuples
6.2.1.2.4. Strings
6.2.1.3. Mutable data types cannot be used as keys
6.2.1.3.1. Lists
6.2.1.3.2. Sets
6.2.1.3.3. Other dictionaries
6.2.1.4. Item methods
6.2.1.4.1. keys ( )
6.2.1.4.2. values ( )
6.2.1.4.3. items ( )
6.2.2. Sets
6.2.2.1. A data structure in Python that contains only unordered, non-interchangeable elements
6.2.2.2. Imagine you have a bag of marbles. You can have marbles of different colors, but you can't have the same color marble twice. That's what a set in Python is like! It's a way to store unique items. You can add or remove marbles from the bag, but each marble inside will be one of a kind.
6.2.2.3. Functions
6.2.2.3.1. Create sets
6.2.2.3.2. intersection ( )
6.2.2.3.3. difference ( )
6.2.2.3.4. symmetric_difference ( )
6.2.2.3.5. union ( )
6.3. Arrays and vectors with NumPy
6.3.1. Library (or package)
6.3.1.1. Broadly refers to a reusable collection of code
6.3.1.1.1. matplotlib
6.3.1.1.2. Seaborn
6.3.2. Python libraries
6.3.2.1. NumPy
6.3.2.1.1. An essential library that contains multidimensional array and matrix data structures and functions to manipulate them
6.3.2.2. pandas
6.3.2.2.1. A powerful library built on top of NumPy that's used to manipulate and analyze tabular data
6.3.3. Module
6.3.3.1. A simple Python file containing a collection of functions and **global variables**
6.3.3.1.1. Global variables: variables that can be accessed from anywhere in a program or script
6.3.3.2. Commonly used Python modules
6.3.3.2.1. Math
6.3.3.2.2. Random
6.3.3.2.3. Datetime
6.3.4. Import statement
6.3.4.1. Uses the import keyword to load an external library, package, module, or function into your computing environment
6.3.5. N-dimentional array (ndarray)
6.3.5.1. The core data object of NumPy
6.3.5.2. contain data of the same type
6.3.5.3. attribute
6.3.5.3.1. dtype
6.3.5.3.2. shape
6.3.5.3.3. ndim
6.3.5.4. method
6.3.5.4.1. NumPy method used to change the shape of an array
6.4. Dataframes with pandas
6.4.1. Primary data structures
6.4.1.1. Series
6.4.1.1.1. A one-dimensional, labeled array
6.4.1.2. DataFrame
6.4.1.2.1. A two-dimensional, labeled data structure with rows and columns
6.4.2. Attributes and methods
6.4.2.1. Attributes
6.4.2.1.1. An attribute is a value associated with an object or class that is referenced by name using dotted expressions
6.4.2.2. Methods
6.4.2.2.1. A method is a function that is defined inside a class body and typically performs an action
6.4.3. Boolean masking
6.4.3.1. A filtering technique that overlays a Boolean grid onto a dataframe in order to select only the values in the dataframe that align with the True values of the grid
6.4.3.1.1. Logical operators
6.4.4. Grouping and aggregation
6.4.4.1. groupby ( )
6.4.4.1.1. A pandas DataFrame method that groups rows of the dataframe together based on their values at one or more columns, which allows further analysis of the groups
6.4.4.2. agg ( )
6.4.4.2.1. Short for "aggregate.". A pandas groupby method that allows you to apply multiple calculations to groups of data
6.4.5. Pandas functions
6.4.5.1. concat ( )
6.4.5.1.1. A pandas function that combines data either by adding it horizontally as new columns for existing rows, or vertically as new rows for existing columns
6.4.5.2. merge ( )
6.4.5.2.1. A pandas function that joins two dataframes together; it only combines data by extending along axis one horizontally
6.4.6. NaN
6.4.6.1. How null values are represented in pandas, which stands for "not a number"
6.4.7. iloc [ ]
6.4.7.1. A way to indicate in pandas that you want to select by integer-location-based position
6.4.8. loc [ ]
6.4.8.1. Used to select pandas rows and columns by name
6.5. Data structures
6.5.1. Collections of data values or objects that contain different data types