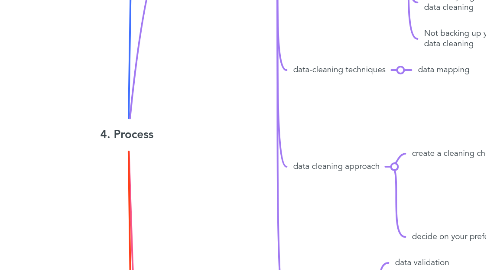
1. Data integrity
1.1. definition
1.1.1. data integrity
1.1.1.1. the accuracy, completeness, consistency, and trustworthiness of data throughout its lifecycle
1.1.2. data replication
1.1.2.1. the process of storing data in multiple locations
1.1.3. data transfer
1.1.3.1. the process of copying data from a storage device to memory, or from one computer to another
1.1.4. data manipulation
1.1.4.1. the process of changing data to make it more organized and easier to read
1.2. other threats to data integrity
1.2.1. human error
1.2.2. viruses
1.2.3. malware
1.2.4. hacking
1.2.5. system failures
1.3. data constraint
1.3.1. data type
1.3.2. data range
1.3.3. mandatory
1.3.4. unique
1.3.5. Regular expression (regex) patterns
1.3.6. Cross-field validation
1.3.7. Primary-key
1.3.8. Set-membership
1.3.9. Foreign-key
1.3.10. Accuracy
1.3.11. Completeness
1.3.12. Consistency
1.4. Types of insufficient data
1.4.1. data only from only one source
1.4.2. data that keeps updating
1.4.3. outdated data
1.4.4. geographically-limited
1.5. Ways to address insufficient data
1.5.1. identify trends with the available data
1.5.2. wait for more data if time allows
1.5.3. talk with stakeholders and adjust your objective
1.5.4. look for new dataset
1.6. population vs sample
1.6.1. sample size
1.6.1.1. a part of population that is representative of the population
1.6.1.1.1. pros
1.6.1.1.2. cons
1.6.2. random sampling
1.6.2.1. a way of selecting a sample from a population that every possible type of the sample has an equal chance of being chosen
1.6.3. proxy data
1.7. statistical power
1.7.1. statistically significant
1.7.1.1. the results of the test are real and not an error caused by random chance
1.7.2. statistical power at least 80% to consider your results statistically significant
1.8. confidence level
1.8.1. the probability that your sample size accurately reflects the greater population
1.8.2. most countries expect a 90% or 95% confidence level
1.9. margin of error
1.9.1. the maximum amount that the sample results are expected to differ from those of the actual population
1.10. schema
1.10.1. a way of describing how something is organized
2. Clean vs dirty data
2.1. definition
2.1.1. dirty data
2.1.1.1. data that is incomplete, incorrect or irrelevant to the proble you're trying to solve
2.1.2. clean data
2.1.3. data engineers
2.1.3.1. transform data into a useful format for analysis and give it a reliable infrastructure
2.1.4. data warehousing specialists
2.1.4.1. develop processes and procedures to effectively store and organize data
2.1.5. compatibility
2.1.5.1. how well two or more datasets are able to work together
2.2. types of dirty data
2.2.1. duplicate data
2.2.2. outdated data
2.2.3. incomplete data
2.2.4. incorrect/inaccurate data
2.2.5. inconsistent data
2.3. common mistakes to avoid
2.3.1. Not checking for spelling errors
2.3.2. Forgetting to document errors
2.3.3. Not checking for misfielded values
2.3.4. Overlooking missing values
2.3.5. Only looking at a subset of the data
2.3.6. Losing track of business objectives
2.3.7. Not fixing the source of the error
2.3.8. Not analyzing the system prior to data cleaning
2.3.9. Not backing up your data prior to data cleaning
2.4. data-cleaning techniques
2.4.1. data mapping
2.5. data cleaning approach
2.5.1. create a cleaning checklist
2.5.1.1. determine the size of the dataset
2.5.1.2. determine the number of categories or labels
2.5.1.3. identify missing data
2.5.1.4. indentify unformatted data
2.5.1.5. explore the different data types
2.5.2. decide on your preferred methods
2.6. data cleaning tools
2.6.1. data validation
2.6.2. conditional formatting
2.6.3. COUNTIF
2.6.4. sorting
2.6.5. filtering
3. Data cleaning with SQL
3.1. Relational databases
3.1.1. a database that contains a series of tables that can be connected to form relationships
3.2. Spreadsheets versus SQL
3.2.1. features of spreadsheets
3.2.1.1. Smaller data sets
3.2.1.2. Enter data manually
3.2.1.3. Create graphs and visualizations in the same program
3.2.1.4. Built-in spell check and other useful functions
3.2.1.5. Best when working solo on a project
3.2.2. features of SQL
3.2.2.1. Larger datasets
3.2.2.2. Access tables across a database
3.2.2.3. Prepare data for further analysis in another software
3.2.2.4. Fast and powerful functionality
3.2.2.5. Great for collaborative work and tracking queries run by all users
3.2.3. spreadsheets
3.2.3.1. Generated with a program
3.2.3.2. Access to the data you input
3.2.3.3. Stored locally
3.2.3.4. Small datasets (1 million rows)
3.2.4. SQL
3.2.4.1. A language used to interact with database programs
3.2.4.2. Can pull information from different sources in the database
3.2.4.3. Stored across a database
3.2.4.4. Larger datasets
3.2.4.5. Tracks changes across team
3.3. Data-cleaning functions
3.3.1. LENGTH
3.3.2. SUBSTR (column name, position of the first letter, how many letters you want to cut including the first letter)
3.3.3. TRIM
3.3.4. CAST ( )
3.3.4.1. convert anything from one data type to another
3.3.5. CONCAT ( )
3.3.5.1. add strings together to create new text strings that can be used as unique keys
3.3.6. COALESCE ( )
3.3.6.1. return non-null values in a list
4. Verify and report cleaning results
4.1. definition
4.1.1. changelog
4.1.1.1. a file containing a chronologically ordered list of modifications made to a project
4.2. see the big picture when verifying data-cleaning
4.2.1. 1. consider the business problem
4.2.2. 2. consider the goal
4.2.3. 3. consider the data
4.2.4. ask yourself "Do the numbers make sense?"
4.3. correct the most common problems
4.3.1. Sources of errors: Did you use the right tools and functions to find the source of the errors in your dataset?
4.3.2. Null data: Did you search for NULLs using conditional formatting and filters?
4.3.3. Misspelled words: Did you locate all misspellings?
4.3.4. Mistyped numbers: Did you double-check that your numeric data has been entered correctly?
4.3.5. Extra spaces and characters: Did you remove any extra spaces or characters using the TRIM function?
4.3.6. Duplicates: Did you remove duplicates in spreadsheets using the Remove Duplicates function or DISTINCT in SQL?
4.3.7. Mismatched data types: Did you check that numeric, date, and string data are typecast correctly
4.3.8. Messy (inconsistent) strings: Did you make sure that all of your strings are consistent and meaningful?
4.3.9. Messy (inconsistent) date formats: Did you format the dates consistently throughout your dataset?
4.3.10. Misleading variable labels (columns): Did you name your columns meaningfully?
4.3.11. Truncated data: Did you check for truncated or missing data that needs correction?
4.3.12. Business Logic: Did you check that the data makes sense given your knowledge of the business?
4.4. Documentation
4.4.1. the process of tracking changes, additions, deletions, and errors involved in your data-cleaning effort
4.4.1.1. recover data-cleaning errors
4.4.1.2. inform other users of changes
4.4.1.3. determine quality of data
4.5. Common data errors
4.5.1. human error in data entry
4.5.2. flawed processes
4.5.3. system issues