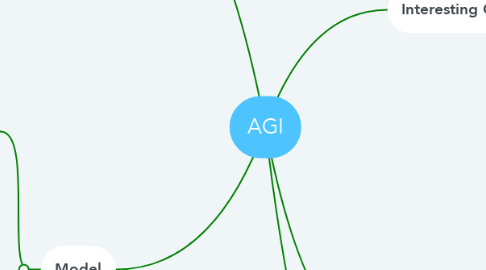
1. Env
1.1. Evaluation
1.1.1. Unity-perception
1.1.1.1. Part-whole recognition
1.1.1.2. Occlusion
1.1.1.2.1. Stella Yu
1.1.2. Innate Physics
1.1.2.1. Flying toycar
1.1.2.2. Disappearing toy car
1.1.3. social aspect
1.1.3.1. Ethics in early child
1.1.3.1.1. attending to the toy that is doing good behavior
2. Model
2.1. Intrinsic motivation
2.1.1. Novelty-based
2.1.1.1. Uncertainty-based
2.1.1.2. Information-gain
2.1.1.2.1. Eq3.2
2.1.1.2.2. Eq3.3
2.2. Top-down feedback
2.2.1. HTM
2.2.2. Capsule Network
2.3. Regularization
2.3.1. L1 regularization lasso
2.3.2. Dropout
2.3.3. Spike coding
2.3.4. Sex
2.4. Sparse coding
2.4.1. Sparsey
2.4.2. HTM
2.5. prediction
2.5.1. Predictive Learning
2.5.1.1. https://royalsocietypublishing.org/doi/pdf/10.1098/rstb.2018.0030
3. Interesting Groups
3.1. Project AGI
3.2. Models
3.2.1. Numenta
3.2.2. Laurent U Perrinet
3.2.3. Yukie Nagai
3.2.4. Juyang Weng
3.2.5. Pierre-Yves Oudeyer
3.2.6. Neurithmetic
3.2.7. Vicarious
3.2.8. Yoshua Bengio
3.2.8.1. Deep InfoMax
3.2.8.2. Disentangle causal mechnisms
3.3. Environments
3.3.1. Grounded Language
3.3.1.1. Cynthia Matsuszek
3.3.2. Chen Yu
3.3.3. Linda Smith
3.4. Program Manager
3.4.1. Ted Senator
3.4.1.1. Lifelong Learning Machines (L2M)
3.4.1.2. Science of Artificial Intelligence and Learning for Open-World Novelty (SAIL-ON)
4. Sparse coding
4.1. An adaptive homeostatic algorithm for the unsupervised learning of visual features
4.2. A blog article about sparse coding
5. Publication
5.1. Conference
5.1.1. ICDL-Epirob
5.1.2. AGI
5.1.3. International Symposium on AI and Brain Science
5.2. Newsletter
5.2.1. CDS newletter