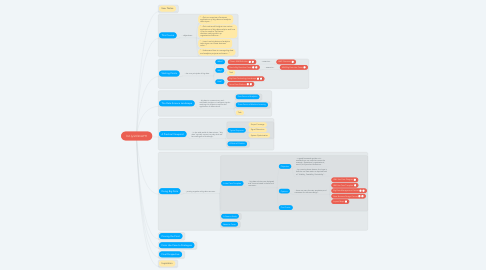
1. The Data Science Landscape
1.1. ...big data is a means to an end - actionable insights or intelligent signals - reaching this endpoint requires the application of data science...
1.1.1. Four Forms of Analytics
1.1.1.1. ...a data scientist uses data to "Describe", "Diagnose", "Predict", or "Prescribe"...
1.1.1.1.1. The Analytics Curve
1.1.2. Three Forms of Machine Learning
1.1.2.1. ...a machine learning specialist applies "Unsupervised", "Supervised", and "Reinforced" learning...
1.1.2.1.1. The Family Tree
1.1.2.1.2. Three Forms of Learning
1.1.2.1.3. ...plus one...
1.1.3. Task
1.1.3.1. Example: Personetics
1.1.3.2. Q: what forms of data science and machine learning ,might be used inside the Personetics solutions?
1.1.3.2.1. Team #1
1.1.3.2.2. Team #2
1.1.3.2.3. Team #3
1.1.3.2.4. Team #4
1.1.3.2.5. Team #5
2. A Practical Viewpoint
2.1. ...in the wide world of data science, "big data" typically comes into play when we face two types of challenge...
2.1.1. Typical Purposes
2.1.1.1. Scope Coverage
2.1.1.1.1. ..."big data" solutions are used to generate insight into systems that are massive in scope or complexity...
2.1.1.2. Signal Detection
2.1.1.2.1. ..."big data" solutions are used to craft predictive or classifier machine learning solutions in contexts where the "signal" is weak or its "mechanism" is complex...
2.1.1.3. System Optimisation
2.1.1.3.1. ..."big data" solutions are used to craft optimisation solutions in contexts where the system complexity is high...
2.1.2. A Note of Caution
2.1.2.1. ...it is not true that all "data science" efforts improve with volume of data...
2.1.2.1.1. Perspectives
3. Final Perspective
3.1. ...the data landscape is always moving...
3.1.1. Data Trends
4. From Use Case to Strategies
4.1. ...a use case does not make a strategy, some additional components are required to land "big data" within the organisation in a meaningful way...
4.1.1. Framing: McKinsey's 10 Flags
4.1.2. Product Management
4.1.2.1. Product Management Framework
4.1.3. Portfolio Management
4.1.3.1. McKinsey Prioritisation
4.1.4. Platforms and Integration
4.1.4.1. Perspective: Microservices
5. Inspiration
5.1. Personalisation
5.1.1. ...examples...
5.1.1.1. Service Personalisation
5.1.1.1.1. Example: Retail Omnichannel Experiences
5.1.1.2. Choice Recommendation
5.1.1.2.1. Example: Recommendation Engines
5.1.1.3. Behavioural Nudge
5.1.1.3.1. Example: Congestion Management
5.1.1.4. Data Driven Marketing
5.1.1.4.1. Example: Facebook Travel Ads
5.2. Operational Optimisation
5.2.1. ...examples...
5.2.1.1. Example: Logistics Optimisation
5.2.1.2. Example: Predicitve Maintenance
5.2.1.3. Example: Intelligence Process Automation
5.2.1.4. Example: Autonomous Service Bots
5.3. Economics and Finance
5.3.1. ...examples...
5.3.1.1. Credit and Premium Scoring
5.3.1.1.1. Example: Health Insurance Models
5.3.1.2. Dynamic Pricing
5.3.1.2.1. Example: Uber
5.3.1.2.2. Example: Dynamic B2B Pricing
5.3.1.3. Fraud Detection
5.3.1.3.1. Example: Internal Audit Analytics
5.3.1.4. Algorithmic Trading
5.3.1.4.1. Example: Algorithmic Trading
5.3.1.4.2. Perspective: Zoom Technologies Inc
5.3.1.5. Market Forecasting
5.3.1.5.1. Example: IMF Outlooks
5.4. Other
5.4.1. ...examples...
5.4.1.1. Sports
5.4.1.1.1. Example: FiveThirtyEight
5.4.1.2. Risk Sensing
5.4.1.2.1. Example: Risk Sensing
5.4.1.3. Environment
5.4.1.3.1. Example: Singapore MSS
5.4.1.4. Robotics
5.4.1.4.1. Example: Level 5 Autonomous Vehicles
5.5. General
5.5.1. Tableau
5.5.2. IBM
6. Proving the Point
6.1. ...we can look at the "playbooks" of "big data" leaders to consolidate our understanding...
6.1.1. Uber Michelangelo
6.1.1.1. "...Successfully scaling ML at a company like Uber requires getting much more than just the technology right—there are important considerations for organization and process design as well. In this section, we look at critical success factors across three pillars: organization, process, as well as technology..."
6.1.2. Task
6.1.2.1. Q. translate the Michelangelo story on to a PTW Canvas...
6.1.2.1.1. Team #1
6.1.2.1.2. Team #2
6.1.2.1.3. Team #3
6.1.2.1.4. Team #4
6.1.2.1.5. Team #5
7. Doing Big Data
7.1. ...putting together a big data use case...
7.1.1. A Use Case Template
7.1.1.1. ...big data solutions are designed and communicated in the form of use cases...
7.1.1.1.1. Objective
7.1.1.1.2. Options
7.1.1.1.3. Our Choice
7.1.2. A Case to Study
7.1.2.1. ...we can explore and understand the set of considerations underpinning "big data" solutions and applications by "decomposing" a solid example with lots going on behind the scenes...
7.1.2.1.1. Ogilvy and German Rail
7.1.3. Bases to Cover
7.1.3.1. 1. Aspirations
7.1.3.1.1. ...focus on...
7.1.3.1.2. Task
7.1.3.2. 2. Spaces
7.1.3.2.1. ...focus on...
7.1.3.2.2. Task
7.1.3.3. 3. Methods
7.1.3.3.1. ...focus on...
7.1.3.3.2. Task
7.1.3.4. 4. Capabilities
7.1.3.4.1. ...focus on...
7.1.3.4.2. Task
7.1.3.5. 5. Systems
7.1.3.5.1. ...focus on...
7.1.3.5.2. Task
8. Starting Points
8.1. ...the core principles of big data...
8.1.1. What?
8.1.1.1. Classic IBM Definition
8.1.1.1.1. ...extension...
8.1.2. Why?
8.1.2.1. Oracle Big Data Use Cases
8.1.2.1.1. ...extension...
8.1.2.2. Task
8.1.2.2.1. Q: what is the "purpose" and "value" of big data in the Oracle Paper?
8.1.3. How?
8.1.3.1. Big Data Technology Landscape
8.1.3.2. Azure Data Platform
8.1.3.2.1. ...distinctive elements...
9. This Course
9.1. ...objectives...
9.1.1. "...Gain an overview of business applications of big data and analytics techniques..."
9.1.2. "...Gain real-world insights into various applications of big data analytics and how it can be used to fuel better decision-making within an organisation/business..."
9.1.3. "...Learn how big data and analytics techniques can create business value..."
9.1.4. "...Understand how to manage big data and analytics projects and teams..."
10. User Notes
10.1. ...notes on tools used in this session...
10.1.1. Mindmeister
10.1.1.1. Copy Map
10.1.2. Jamboards
10.1.2.1. Copy Boards