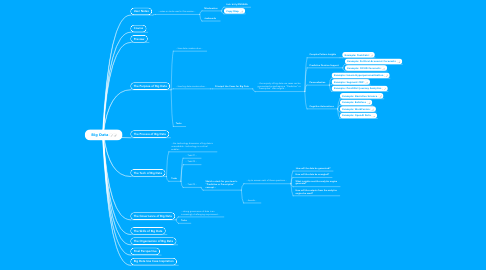
1. The Organisation of Big Data
1.1. ...when we move from one use case to an broader big data strategy, we must consider organisation...
1.1.1. Points of Failure
1.1.1.1. ...big data analytics initiatives routinely fail, why?...
1.1.1.1.1. Framing: McKinsey's 10 Flags
1.1.1.2. ...many of these failures derive from organisational, not technical issues...
1.1.1.2.1. Critical Mindsets
1.1.2. Crafting a CoE
1.1.2.1. ...a big data Centre of Excellence trading on an internal market...
1.1.2.1.1. Content
1.1.2.1.2. Positioning
1.1.2.1.3. ...we can look at the "playbooks" of "big data" leaders to consolidate our understanding...
1.2. Tasks
1.2.1. ...Task #1...
1.2.1.1. Translate your team's big data concept to a "Play to Win" canvas...
1.2.1.1.1. ...guide...
1.2.1.1.2. ...boards...
1.2.2. ...Task #2...
1.2.2.1. Make the case for your big data concept...
1.2.2.1.1. Case Making
1.2.2.1.2. ...boards...
1.2.3. ...Task #3...
1.2.3.1. Where will you take your concept next?
1.2.3.1.1. Portfolios
1.2.3.1.2. ...boards...
2. The Process of Big Data
2.1. ...process patterns are critical to unlocking the value of big data...
2.1.1. Principal Data Process Pattern
2.1.1.1. ...some process patterns are concerned with anchoring the data science to business needs...
2.1.1.1.1. CRISP-DM
2.1.2. Machine Learning Process Patterns
2.1.2.1. ...some process patterns are concerned with enabling the machine to learn...
2.1.2.1.1. Machine Learning Process Patterns
2.1.2.1.2. Supervised Learning Deep Dive
2.1.3. Hybrid Process Pattern
2.1.3.1. ...success requires fusions of CRISP-DM and Supervised Learning Pattern...
2.1.3.1.1. Example: Uber Michaelangelo
2.1.3.1.2. Illustration: Particle Data
2.2. Tasks
2.2.1. ...Task #1...
2.2.1.1. Example: German Rail
2.2.1.1.1. Consider how the image recognition element in this example was put together from a process perspective...
2.2.2. ...Task #2...
2.2.2.1. Develop your "Predictive or Prescriptive" concept - what is the process?
2.2.2.1.1. ...process details...
2.2.2.1.2. ...boards...
3. The Tech of Big Data
3.1. ...the technology dimension of big data is unavoidable - technology is a critical enabler...
3.1.1. Previous Starting Point
3.1.1.1. Big Data Technology Landscape
3.1.1.2. Azure Data Platform
3.1.1.2.1. ...three distinctive challenges...
3.1.2. Big Data Stacks
3.1.2.1. ...in big data, the technology stack typically takes the form of a pipeline architecture...
3.1.2.1.1. Pipeline Architecture Outline
3.2. Tasks
3.2.1. ...Task #1...
3.2.1.1. Observe tech in StackShare.io
3.2.2. ...Task #2...
3.2.2.1. Build a stack in Crowdcraft.co
3.2.2.1.1. Target Stack
3.2.2.1.2. ...steps...
3.2.3. ...Task #3...
3.2.3.1. Sketch a stack for your team's "Predictive or Prescriptive" concept...
3.2.3.1.1. ...try to answer each of these questions...
3.2.3.1.2. ...boards...
4. Final Perspective
4.1. ...the data landscape is always moving...
4.1.1. Data Trends
5. Big Data Use Case Inspiration
5.1. Personalisation
5.1.1. ...examples...
5.1.1.1. Service Personalisation
5.1.1.1.1. Example: Retail Omnichannel Experiences
5.1.1.2. Choice Recommendation
5.1.1.2.1. Example: Recommendation Engines
5.1.1.3. Behavioural Nudge
5.1.1.3.1. Example: Congestion Management
5.1.1.4. Data Driven Marketing
5.1.1.4.1. Example: Facebook Travel Ads
5.2. Operational Optimisation
5.2.1. ...examples...
5.2.1.1. Example: Logistics Optimisation
5.2.1.2. Example: Predicitve Maintenance
5.2.1.3. Example: Intelligence Process Automation
5.2.1.4. Example: Autonomous Service Bots
5.3. Economics and Finance
5.3.1. ...examples...
5.3.1.1. Credit and Premium Scoring
5.3.1.1.1. Example: Health Insurance Models
5.3.1.2. Dynamic Pricing
5.3.1.2.1. Example: Uber
5.3.1.2.2. Example: Dynamic B2B Pricing
5.3.1.3. Fraud Detection
5.3.1.3.1. Example: Internal Audit Analytics
5.3.1.4. Algorithmic Trading
5.3.1.4.1. Example: Algorithmic Trading
5.3.1.4.2. Perspective: Zoom Technologies Inc
5.3.1.5. Market Forecasting
5.3.1.5.1. Example: IMF Outlooks
5.4. Other
5.4.1. ...examples...
5.4.1.1. Sports
5.4.1.1.1. Example: FiveThirtyEight
5.4.1.2. Risk Sensing
5.4.1.2.1. Example: Risk Sensing
5.4.1.3. Environment
5.4.1.3.1. Example: Singapore MSS
5.4.1.4. Robotics
5.4.1.4.1. Example: Level 5 Autonomous Vehicles
5.5. General
5.5.1. Tableau
5.5.2. IBM
6. The Governance of Big Data
6.1. ...strong governance of data is an increasingly challenging requirement...
6.1.1. Big Data Governance
6.1.1.1. Frameworks
6.1.1.1.1. DAMA DMBOK
6.1.1.2. Challenges
6.1.1.2.1. Privacy, Access, and Use
6.1.1.2.2. Accuracy, Explainability, and Fairness
6.1.1.3. Tools
6.1.1.3.1. ...general...
6.1.1.3.2. ...privacy...
6.1.1.3.3. ...FEAT...
6.2. Tasks
6.2.1. ...Task #1...
6.2.1.1. Assess the "FEAT" exposure of your team's big data concept...
6.2.1.1.1. MAS FEAT
6.2.1.1.2. ...boards...
6.2.2. ...Task #2...
6.2.2.1. Assess the GDPR/PDPA exposure of your team's big data concept...
6.2.2.1.1. Exhibit: GDPR and PDPA
6.2.2.1.2. ...boards...
7. Preview
7.1. ...the core principles of big data...
7.1.1. The "What?"
7.1.1.1. ...technical perspective...
7.1.1.1.1. Classic IBM Definition
7.1.1.2. ...outcomes perspective...
7.1.1.2.1. Facebook and German Rail
7.1.2. The "Why?"
7.1.2.1. ...prediction and prescription use cases...
7.1.2.1.1. Use Case Template
7.1.3. The "How?"
7.1.3.1. Big Data Technology Landscape
7.1.3.2. Azure Data Platform
7.1.3.2.1. ...three distinctive challenges...
8. Course
8.1. ...objectives...
8.1.1. "...Gain an overview of business applications of big data and analytics techniques..."
8.1.2. "...Gain real-world insights into various applications of big data analytics and how it can be used to fuel better decision-making within an organisation/business..."
8.1.3. "...Learn how big data and analytics techniques can create business value..."
8.1.4. "...Understand how to manage big data and analytics projects and teams..."
9. User Notes
9.1. ...notes on tools used in this session...
9.1.1. Mindmeister
9.1.1.1. Link: bit.ly/SMUBAA
9.1.1.2. Copy Map
9.1.2. Jamboards
9.1.2.1. Example
9.1.2.2. Copy Boards
10. The Skills of Big Data
10.1. ...despite all the "tech", success and failure in big data still hinges on people...
10.1.1. Complex Skills Requirements
10.1.1.1. ...problematic unicorn models...
10.1.1.1.1. Example: Data Science Unicorns
10.1.1.2. ...emerging solutions...
10.1.1.2.1. Red, Blue, and Purple Profiles
10.1.1.2.2. Team Structures
10.2. Tasks
10.2.1. ...Task #1...
10.2.1.1. Sketch the skills profile for your team's "Predictive or Prescriptive" concept...
10.2.1.1.1. ...boards...
11. The Purpose of Big Data
11.1. ...how data creates value...
11.1.1. The Analytics Spectrum
11.1.1.1. ...data is used to "Describe", "Diagnose", "Predict", or "Prescribe"...
11.1.1.1.1. The Analytics Curve
11.1.1.2. ..."Prediction" and "Prescription" is the domain of "Machine Learning"...
11.1.1.2.1. Machine Learning Family Tree
11.1.1.2.2. Forms of Machine Learning
11.1.1.3. ..."Machine Learning" is the engine of "Artificial Intelligence"...
11.1.1.3.1. Artificial Intelligence
11.2. ...how big data creates value...
11.2.1. Principal Use Cases for Big Data
11.2.1.1. ...the majority of big data use cases can be summarised as packaging a "Predictive" or "Prescriptive" data engine...
11.2.1.1.1. Complex Pattern Insights
11.2.1.1.2. Predictive Decision Support
11.2.1.1.3. Personalisation
11.2.1.1.4. Cognitive Automations
11.3. Tasks
11.3.1. ...Task #1...
11.3.1.1. Identify an example of Machine Learning being used inside the Personetics solutions, how might it work?
11.3.1.1.1. Example: Personetics
11.3.1.1.2. ...boards...
11.3.2. ...Task #2...
11.3.2.1. Craft a "Predictive or Prescriptive" concept for an industry represented in your team - start by answering "What and Why"?
11.3.2.1.1. ...boards...