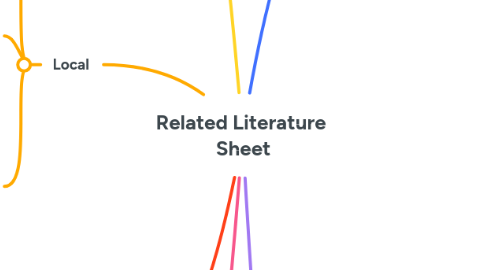
1. Primary
1.1. About VFS Background
1.1.1. **Chen, He, Wang, Li, Guan, and Hao‘** A Study on the Arable Land Demand for Food Security in China [1] **K. Benke and B. Tomkins,** ‘Future food-production systems: vertical farming and controlled-environment agriculture’[2]
1.1.1.1. **Arable Land Limitations**
1.1.1.1.1. "By 2050, arable land will be a third of what was available in 1970"
1.1.2. **Statista,** Forecast for vertical farming market global 2032,” .[14]
1.1.2.1. **Increasing Value for VFS**
1.1.2.1.1. "35 Billion on 2032"
1.1.3. **F. Sandison, J. Yeluripati, and D. Stewart,** “Does green vertical farming offer a sustainable alternative to conventional methods of production?: A case study from Scotland,”
1.1.3.1. **VFS Systems Around the Globe**
1.1.3.1.1. Singapore - SkyGreens Japan - Nuvege Chicago - The Plant
1.2. VFS Automation and Digitalization
1.2.1. **M. S. N. Kabir et al.,** “Technological Trends and Engineering Issues on Vertical Farms: A Review
1.2.1.1. **Trends in Adapting Sensors, Automation, etc.**
1.2.2. **N. S. Abu et al.,** “Internet of Things Applications in Precision Agriculture: A Review,”
1.2.2.1. **Integration of IoT**
1.2.2.1.1. Projections suggest that by 2050, IoT can potentially increase agricultural output by over 70% by implementing existing techniques
2. Local
2.1. **M. P. Pascual, G. A. Lorenzo, and A. G. Gabriel,** ‘Vertical Farming Using Hydroponic System: Toward a Sustainable Onion Production in Nueva Ecija, Philippines [4]
2.1.1. Nueva Ecija
2.2. **S. Tagle, H. Benoza, R. Pena, and A. Oblea,** “Green Technologies Social Impact Development of an Indoor Hydroponic Tower for Urban Farming, [5]
2.2.1. DLSU
2.3. **Gokongwei College of Engineering, De La Salle University,** Manila, Philippines et al., ‘A Comparative Analysis of Machine Learning Algorithms Modeled from Machine Vision-Based Lettuce Growth Stage Classification in Smart Aquaponics’ [11]
2.3.1. DLSU
3. Bases for Objectives
3.1. **G. Rajendiran, J. Rethnaraj** Optimizing Lettuce Crop Yield Prediction in an Indoor Aeroponic Vertical Farming System Using IoT-Integrated Machine Learning Regression Models [123]
3.1.1. **MAPE and R-squared**
3.1.1.1. MAPE of 15.5 and R-squared of 0.574 are the parameters of linear regression. Must be equal to or greater than to be viable
3.2. **C. Sace and E. Navitidad** Economic Analysis of an Urban Vertical Garden for Hydroponic Production of Lettuce (Lactuca sativa)* [93]
3.2.1. **Yield and Economic Parameters**
3.2.1.1. Must be equal to or greater than their BCR to compete with VFS without ML and IoT
4. Patents
4.1. US11825784B1
4.1.1. AI-enabled IoT-fog system in hydroponic automation for heterogeneous plants. This invention features a hydroponic system automation method using the Nutrient Film Technique (NFT) and a Deep Water Culture (DWC).
4.1.1.1. GAPS
4.1.1.1.1. Lacks scalability and modularity, single-unit, uses simple NFT, focuses on fog computing rather than the farming system, does not offer predictive analysis
4.2. US2022295691A1
4.2.1. which presents an AI-based system for managing nutrient concentration in water-based solutions. This system includes hardware processors and a memory that houses several modules
4.2.1.1. GAPS
4.2.1.1.1. Lacks scalability and modularity, single-unit, uses simple NFT, focuses on fog computing rather than the farming system, does not offer predictive analysis, uses a weaker algorithm (Perceptrons)
4.3. WO2021097368A1
4.3.1. introduces an apparatus for autonomous indoor farming. The apparatus comprises a matrix of enclosed growing containers, each housing a movable cart for plants. An articulated robot, capable of moving along the matrix, can insert or extract the carts from the containers.
4.3.1.1. GAPS
4.3.1.1.1. Lacks scalability and modularity, single-unit, consumes large spaces with wide scale DWC, focuses full automation with industrial robots, does not offer predictive analysis, cost
5. Parameters and Crop Growth
5.1. Crop Growth
5.1.1. **M. Majid, J. N. Khan, Q. M. Ahmad Shah, K. Z. Masoodi, B. Afroza, and S. Parvaze,** “Evaluation of hydroponic systems for the cultivation of Lettuce (Lactuca sativa L., var. Longifolia) and comparison with protected soil-based cultivation
5.1.1.1. deep water culture plants consistently achieved the highest yields, recording significantly greater yields of 1.636 and 1.645 kilograms per plant
5.1.1.1.1. WHY DEEP WATER CULTURE (DWC)
5.1.2. R. Wildeman, “Vertical farming: a future perspective or a mere conceptual idea
5.1.2.1. VFS significantly outproduce traditional farming, achieving over 80 times the yield due to multiple harvests per year and higher plant density.
5.1.2.1.1. WHY VFS
5.2. Nutrient Content of Hydroponics Crops
5.2.1. **M. A. O. K. Albadwawi, Z. F. R. Ahmed, S. S. Kurup, M. A. Alyafei, and A. Jaleel,** “A Comparative Evaluation of Aquaponic and Soil Systems on Yield and Antioxidant Levels in Basil, an Important Food Plant in Lamiaceae,”
5.2.1.1. the aquaponic crops exhibited higher levels of key macronutrients, including nitrogen phosphorus, and magnesium, while, potassium, calcium, and sulfur levels were similar between the two systems.
5.2.1.1.1. WHAT IS THE ADVANTAGE OF HYDROPONICS VS SOIL
5.3. Water Consumption
5.3.1. **L. Barbosa et al.,** “Comparison of Land, Water, and Energy Requirements of Lettuce Grown Using Hydroponic vs. Conventional Agricultural Methods
5.3.1.1. Hydroponics required 13 times less water than soil cultivation
5.3.1.1.1. WHAT IS THE ADVANTAGE OF HYDROPONICS VS SOIL
5.3.2. S. T. Magwaza, L. S. Magwaza, A. O. Odindo, and A. Mditshwa, “Hydroponic technology as decentralised system for domestic wastewater treatment and vegetable production in urban agriculture
5.3.2.1. A technology utilized in vertical farming was conferred that necessitates 70% less water. The water that is required can also be recycled
5.4. **Light Intensity**
5.4.1. **L. Tuomisto,** “Vertical Farming and Cultured Meat: Immature Technologies for Urgent Problems
5.4.1.1. The findings indicate that LED lights, which emit a reddish-purple color due to a proportion of three parts red light to one-part blue light, can promote plant growth.
5.4.2. **Ben-Asher, A. Garcia Y Garcia, I. Flitcroft, and G. Hoogenboom, **“Effect of atmospheric water vapor on photosynthesis, transpiration and canopy conductance: A case study in corn
5.4.2.1. The advised light intensity for vegetable crops falls within the range of 150 to 200 µmol m−2 s−1
5.4.2.1.1. WHAT IS THE REQUIREMENT FOR LIGHT IN ANY CROP
5.5. Ambient Temperature
5.5.1. **N. G. BRUSATORE, R. Adolf, R. G. Fraser, R. R. Adolf.** “Vertical growing tower for automated horticulture and agriculture **W. Went**, “The Effect of Temperature on Plant Growth
5.5.1.1. internal temperature ideally between 20-30 degrees Celsius. It indicates that the best temperatures for plant growth are generally quite high, typically at or above 25 degrees Celsius
5.5.1.1.1. WHAT IS THE IDEAL TEMPERATURE FOR CROP GROWTH
5.6. Humidity
5.6.1. **Brechner, M., Both, A.J., CEA Staff.** Hydroponic Lettuce Handbook: Cornell Controlled Environment Agriculture
5.6.1.1. for optimal growth of lettuce and other leafy greens, the RH should ideally be maintained between 50% and 70%
5.6.1.1.1. WHAT IS THE IDEAL RELATIVE HUMIDITY FOR CROPS
5.7. **pH Level**
5.7.1. **Y. D. Chuah, J. V. Lee, S. S. Tan, and C. K. Ng,** “Implementation of smart monitoring system in vertical farming
5.7.1.1. Most vegetables that are commonly served in restaurants need a pH level in the middle of 5.5 and 6.5 for favorable growth
5.7.1.1.1. WHAT IS THE IDEAL PH LEVEL OF WATER FOR CROPS
5.8. Nutrient Solution
5.8.1. H. Hosseini, V. Mozafari, H. R. Roosta, H. Shirani, P. C. H. Van De Vlasakker, and M. Farhangi, “Nutrient Use in Vertical Farming: Optimal Electrical Conductivity of Nutrient Solution for Growth of Lettuce and Basil in Hydroponic Cultivation,
5.8.1.1. For lettuce, the optimal EC level is 0.9 dS m-1
5.8.1.1.1. Out of Scope
5.9. Water Level
5.9.1. **M. Tavan, B. Wee, G. Brodie, S. Fuentes, A. Pang, and D. Gupta,** “Optimizing Sensor-Based Irrigation Management in a Soilless Vertical Farm for Growing Microgreens,
5.9.1.1. Yields did not differ significantly
6. Machine Learming
6.1. Random Forest
6.1.1. **M. A. Rahman, N. R. Chakraborty, A. Sufiun, S. K. Banshal, and F. R. Tajnin,** “An AIoT-based hydroponic system for crop recommendation and nutrient parameter monitorization
6.1.1.1. Random Forest demonstrated superior performance across key metrics, achieving an accuracy of 0.975, an F1 score of 0.98, and high precision and recall scores (0.98 and 0.97, respectively), outperforming algorithms like Decision Tree, K-Nearest Neighbors (KNN), and SVM
6.2. Multiple Linear
6.2.1. **A. Fuentes,** Hands-on predictive analytics with Python: master the complete predictive analytics process, from problem definition to model deployment
6.2.1.1. Multiple Linear Regression provides direct insights into how each environmental factor (e.g., pH, light, nutrient levels) influences crop yield. If the relationship between factors and crop yield is approximately linear, MLR can yield reasonable predictions with minimal computational resources
6.3. Single Linear
6.3.1. **A. Fuentes,** Hands-on predictive analytics with Python: master the complete predictive analytics process, from problem definition to model deployment
6.3.1.1. Single Linear Regression (SLR) is a fundamental regression technique that models the relationship between a single independent variable x and a dependent variable Y. This model is often used to predict outcomes based on one key predictor, making it simpler and more interpretable than multiple regression models.