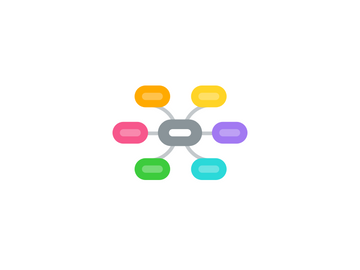
1. Statistical significance testing (basics)
1.1. p value
1.1.1. tutorials
1.1.1.1. one of the best videos explaining p value
1.1.1.2. .
1.1.2. .
1.1.3. .
1.2. confidence intervals
1.2.1. critical value: the region between critical values is called the confidence interval
1.2.2. critical region: Set of all possible test statistic values that would cause us to reject the null hypothesis
1.2.3. .
1.2.4. .
1.2.5. very useful videos
1.2.5.1. .
1.2.5.2. .
1.3. statistical significance
1.3.1. What Does Statistically Significant Mean?
1.4. type1 error and type 2 error
1.4.1. very useful video about type 1 and 2 error
1.4.2. interactive tutorial
1.4.3. .
1.5. hypothesis
1.5.1. alternative and null hypothesis
1.5.2. hypothetical-deductive model
1.5.3. http://www.socialresearchmethods.net/kb/hypothes.php
1.5.4. inferential statistics
1.6. one tail or tow tails test?
1.7. examples of when statistical significance is applied?
1.7.1. t-test
1.7.1.1. very useful in explaining how to interprete t-test
1.7.1.2. .
1.7.1.3. how to read the t-test stata output
1.7.1.4. .
1.7.2. ANOVA
1.7.2.1. one-way ANOVA
1.7.2.2. F ratio
1.7.2.3. Least significant difference
1.7.2.4. useful presentation explaining t-test and ANOVA
1.7.3. chi-square
1.7.3.1. useful tutorials
1.7.3.1.1. 1
1.7.3.1.2. 2
1.7.3.1.3. 3
1.7.3.1.4. 4
1.8. .
1.8.1. .
2. the goal of statistical analysis
2.1. the goals- according to EDPSY502
2.1.1. compare means/medians
2.1.1.1. investigating statistical differences is different that investigating statistical relationships
2.1.2. correlate
2.1.3. analysis of contingency (association)
2.2. relationships
2.2.1. scatter plot
2.2.1.1. algebra review of lines
2.2.1.1.1. slop--- intercept form of a line: y=mx+b
2.2.1.2. when we look at a scatter plot we are interested in
2.2.1.2.1. direction + or -
2.2.1.2.2. strength: the tighter the plots the stronger the relationship
2.2.1.2.3. shape: linear or curvy or.....
2.2.1.2.4. is there a relationship? for example: is the slop near zero?
2.2.2. linear relationships
2.2.2.1. bivariate relationships: relationship between two variables
2.2.2.1.1. simple linear regression
2.2.2.1.2. covariance
2.2.2.1.3. correlation
2.2.3. non-linear relationships
2.2.3.1. quadratic
2.2.3.2. exponential
2.2.3.3. polynominal
3. Factor analysis
3.1. reliability and item analysis
3.1.1. scale reliability is based on the correlations between the individual items or measurements that make up the scale, relative to the variances of the items.
3.1.2. .
3.2. what is it? and what's its purpose?
3.2.1. .
3.2.2. .
3.2.3. .
3.2.3.1. so in short: factor analysis is a data reduction method
3.3. types of factor analysis
3.3.1. exploratory factor analysis
3.3.1.1. Principle component analysis is also called R-mode
3.3.1.1.1. .
3.3.1.2. principal component is tecnically not a factor analysis technique. It's a data reduction technique. however, it gives the same results as factor analysis
3.3.2. confirmatory factor analysis
3.4. conceptual model
3.4.1. .
3.4.2. .
3.5. assumptions
3.5.1. .
3.5.2. .
3.5.3. .
3.5.4. .
3.5.5. .
3.5.5.1. .
3.5.5.2. .
3.5.5.2.1. .
3.5.5.2.2. .
3.5.6. .
3.5.7. FA is a model that requires participation of large samples because to make the correlation clearer (reduce the amount of noise), we need large n of cases.
3.6. communalities
3.6.1. .
3.6.1.1. .
3.7. explained variance
3.7.1. .
3.7.1.1. .
3.8. Eigen Values
3.8.1. .
3.8.2. .
3.9. scree plot
3.9.1. .
3.9.2. .
3.9.3. .
3.10. factor selection
3.10.1. .
3.10.2. .
3.10.3. .
3.10.4. .
3.10.5. .
3.11. factor loading
3.11.1. .
3.11.1.1. rotation tells you the proportion of an item correlated to the factor. therefore, an item may contribute to different factors with different proportions
3.11.2. .
3.11.3. .
3.12. factor rotation
3.12.1. .
3.12.2. .
3.12.3. .
3.12.4. .
3.12.5. .
3.12.6. .
3.13. interpretability
3.13.1. .
3.13.2. .
3.14. factor analysis in practice
3.14.1. .
3.14.2. .
3.15. videos
3.15.1. the concept of Factor Analysis
3.15.1.1. this channel has videos of SEM boot camp
3.15.2. Factor Analysis Visualized
3.15.3. Variables and Factor Analysis
3.15.4. A Beginner’s Guide to Factor Analysis: Focusing on Exploratory Factor Analysis
3.15.5. factor analysis- wikipedia
4. HLM
5. statistical reference websites
5.1. Introductory statistics This blog is a place for me to talk about various topics relevant for students taking introductory statistics at community colleges and universities.
5.2. stat news
5.3. math is fun
5.4. Dr. Alexander Wiseman Youtube Channel
5.5. Laerd Statistics
5.6. Minitab Support
5.7. Jim Grange Youtuve Channel
5.8. Math Guy Zero Youtube Channel
5.9. Stats Make Me Cry
5.10. SPSS tutorials by MyCalStateLA Youtube Channel
5.11. Institute of Digital research and Education- UCLA
5.12. Brandon Foltz Youtube Channel
5.13. Star Trek
5.14. NurseKillam Youtube Channel
5.15. Social Research Methods Knowledge Base
5.16. Stata software tutorials
5.17. Statistics How to
5.18. J David Eisenberg Youtube Channel
5.19. graph pad statistics guide
5.20. statistics learning center
6. comparison tests
6.1. test depend on the type of data
6.2. the test type depends on the number of samples
6.3. parametric and non-parametric tests
6.3.1. t-test
6.3.1.1. when to use t-test or z-test
6.3.1.1.1. .
6.3.1.1.2. .
6.3.1.1.3. .
6.3.1.1.4. .
6.3.1.2. very good explanation of what a t-score man
6.3.2. ANOVA family
6.3.2.1. one way ANOVA, 2 ways/factors, 3 ways/factor ANOVA (factorial ANOVA)
6.3.2.1.1. links
6.3.2.1.2. F distribution/ F test
6.3.2.1.3. use of Welch when violation of homogeneity (when Levenne test is significant)
6.3.2.1.4. use Brown-Forsythe when violation of normality
6.3.2.1.5. effect size why?
6.3.2.1.6. 2 ways ANOVA
6.3.2.1.7. ANCOVA
6.3.2.2. difference between ANOVA, MANOVA, ANCOVA, MANCOVA
6.3.2.3. ANOVA with repeated measures
6.3.2.3.1. use repeated measure when you want to test a score at 3 different times or more
6.3.2.4. MANOVA
6.3.2.4.1. useful video on how to report MANOVA results
6.3.2.4.2. .
6.3.2.5. ANCOVA
6.3.2.6. MANCOVA
6.3.3. post hoc test
6.3.3.1. .
6.3.4. select the appropriate test
6.3.4.1. .
6.3.4.2. .
6.3.4.3. .
6.3.4.4. .
6.3.4.5. very useful link. A tree of how to choose the appropriate test and how to conduct that test in STATA
6.3.4.6. what statistical analysis should I use
6.3.5. regression
6.3.5.1. model building and validation
6.3.5.1.1. it means validating prediction and generalizing accuracy
6.3.5.1.2. links
6.3.5.1.3. model building means choosing predictors
6.3.5.1.4. curve fit
6.3.5.1.5. To Explain or to Predict?
6.3.5.1.6. How High Should R-squared Be in Regression Analysis?
6.3.5.1.7. fitting the model OR model validation
6.3.5.2. dummy variables
6.3.5.2.1. very useful link of how to use dummy variables in regression
6.3.5.2.2. regression with dummy variables in SPSS
6.3.5.3. multiple regression
6.3.5.3.1. tutorial of Alex Wiseman
6.3.5.3.2. how to interpret the results of "regression"
6.3.5.3.3. difference between simple linear regression and multiple regression
6.3.5.3.4. preparations before conducting multiple regression
6.3.5.3.5. correlation coefficient: We need to standardize the covariance in order to allow us to better interpret and use it in forecasting, and the result is the correlation calculation.
6.3.5.3.6. basic explanation of regression
6.3.5.4. logistic regression
6.3.5.4.1. examples of logistic (logit) regression and how to interpreter its output in STATA
6.3.5.4.2. HOW DO I INTERPRET ODDS RATIOS IN LOGISTIC REGRESSION? | STATA FAQ
6.3.5.4.3. LOGISTIC REGRESSION ANALYSIS | STATA ANNOTATED OUTPUT
6.3.6. non-parametric
6.3.6.1. very useful link
6.3.6.2. what statistical analysis should I use
6.3.7. chi square test
6.3.7.1. chi square test of independence-- alternative name: test of association
6.3.7.1.1. contingency tables (cross tabs)
6.3.7.1.2. the difference between contingency table and chi square test: the tables provide a foundation for statistical inference, where statistical tests question the relationship between the variables on the basis of the data observed.
6.3.7.2. chi square: goodness tofit
6.3.7.3. correlation coeeficients for data with different levels of measurement
6.3.7.4. bivariate relationships
6.3.7.4.1. notes on bivariate relationships
6.3.7.5. Chi-square test vs. Logistic Regression: Is a fancier test better?
6.3.7.6. types of chi square tests
6.3.7.6.1. Categorical data
6.3.7.6.2. choosing the right test
6.3.8. how to choose a correlation coefficient?
6.3.8.1. .
6.3.9. effect size
6.3.9.1. .
6.3.9.2. .
6.3.9.3. standardized and non-standardized effect size
6.3.9.4. types of effect size
6.3.9.5. why effect size?
6.3.9.5.1. because ANOVA only tells that there is only one group is different and you need to know the size of the difference
6.3.9.5.2. Eta squared (interpreted the same as R squared)
6.3.9.5.3. does the effect size imply causality? I found this article helpful: To Explain or to Predict?
6.4. omnibus test
6.5. multivariate analysis
6.5.1. tutorial course
6.5.2. radar chart
6.5.3. cluster analysis
6.5.3.1. research design using cluster analysis
6.5.3.2. search keywords: cluster analysis research design steps
6.5.3.3. video tutorial
7. research design related topics
7.1. sampling
7.1.1. .
7.1.2. .
7.1.3. very useful slide show about sampling techniques
7.2. experimental design
7.2.1. three basic principles: control- randomization- repetition
7.2.2. experimental design statistics
7.2.2.1. confidence intervals
7.2.2.2. statistical significance tests
7.2.2.3. p values
7.3. statistical decision making
7.3.1. power
7.3.1.1. 0.80 is standard for power analysis
7.3.2. effect size
7.3.3. significance level
7.3.3.1. alpha value: decided before research
7.3.3.2. p value: decided after the research
7.3.4. sample size
7.4. controlling variables
7.4.1. What are control variables and how do I use them in regression analysis?
8. descriptive statistics
8.1. distribution
8.1.1. shape of the distribution OR types of univariate distribution
8.1.1.1. normal and skewed distribution
8.1.1.2. kurtosis
8.1.1.2.1. .
8.1.1.2.2. how to interprete it?-- z-score of 1.96
8.1.1.2.3. if the distribution is normal, the kurtosis and skewness should be zero
8.1.1.3. the mode influence the peak of the distribution
8.1.1.4. skewness
8.1.1.5. test of normality
8.1.1.5.1. stat (of skewness or kurtosis)- standard error. and then compare the outcome with 1.96 (why 1.96? because we measure on 95% level of confidence
8.1.1.5.2. useful presentation of how to test normality
8.1.1.6. http://www.mathsisfun.com/data/standard-normal-distribution.html
8.1.1.7. http://www.mathsisfun.com/data/standard-normal-distribution-table.html
8.1.2. .
8.2. measures of dispersion/ variability
8.2.1. variance
8.2.1.1. sum of squares. why we square the deviations? because their sum is 0 but if we squared them then we can see the variance
8.2.1.2. degrees of freedom
8.2.1.3. The variance of Dichotomous variables (like gender): this type doesn't have a mean. It's called "proportion"
8.2.1.4. What I know is that we cannot interpret variance because it has no sense. For example you can have a variance of 0.5%^2 (percentage squared), so to correct this lack of sense we calculate standard deviation which gives 7.1% in this example. This last value can be compared with other distribution variances. I think talking about variance and standard deviation, from a qualitative perspective, is the same since both are dispersion metrics and their objectives are the same (to quantify dispersion, so risk).
8.2.2. standard deviation
8.2.3. range
8.2.4. interquartile range
8.2.5. Wiseman tutorial of measures of variation
8.2.6. percentile rank
8.3. measures of central tendency
8.3.1. mean, median, mode
8.3.1.1. when can you use the median? when you want to know the point that divide the sample in halves
8.3.2. weighted means
8.3.3. outliers
8.3.4. standard errors of the mean
8.3.4.1. standard error of the estimate (the mean is one type of estimates)
8.3.4.1.1. .
8.3.4.2. standard error of the mean is the standard deviation of the mean of the means
8.3.5. confidence interval
8.3.6. central limit theoreme
8.3.6.1. if we took a large number of samples, then the sampling distribution will be normally distributed
8.4. summary statistics/ index creation
8.4.1. indexes can be created by Primary component analysis or factor analysis
9. concepts of inferential stat
9.1. sample or population
9.2. statistical significance
9.2.1. p<.05 means that the 5% of the results are the outliers
9.2.2. .
9.3. power
9.4. effect size
9.5. error type
9.6. sampling error
9.7. z-score
9.7.1. very useful webpage about how to calculate propability percentage of population between 0 and a z score
9.8. why we need standardized scaling?
9.9. weighing the sample
9.10. EDPSY 550: class subject: rater reliability classic errors in score assignment to constructed response measures: 1- central tendency 2- guessing 3- severity
10. analyzing survailance data/ large data sets
10.1. this file is very useful
10.2. limitations of survalance data
10.2.1. under-reporting
10.2.2. missing data
10.3. population descriptive analysis
10.3.1. stratified sub-groups
10.3.2. standard error of the mean
10.3.3. application of weights
10.4. compute and interprete measures of association
10.5. confidence intervals and/or statistical significance testing
10.5.1. t-test for continious data and chi-square for non-continuous data
10.6. measure the effect of an exposure on risk is distorted because of a potential confounder
10.7. main steps in analyzing large datasets
10.7.1. Conduct basic descriptive analysis
10.7.2. Compute and interpret measures of association
10.7.3. Conduct confidence intervals and/or statistical significance testing
10.7.4. Assess for effect measure modifcation
10.7.5. Assess the effect of potential confounders
10.8. Namey, E., Guest, G., Thairu, L., & Johnson, L. (2008). Data reduction techniques for large qualitative data sets. Handbook for team-based qualitative research, 137-161.
10.9. pisa data analysis
10.9.1. PISATOOLS: Stata module to facilitate analysis of the data from the PISA OECD study
10.9.2. What is done and can be done in Stata-- the STATA journal
10.9.3. weights and replicates
10.9.3.1. Stata Library: Replicate Weights
10.9.3.2. REPEST: Stata module to run estimations with weighted replicate samples and plausible values
10.9.3.3. search keywords: stata syntax weights replicates pisa
10.9.3.4. PISATOOLS: Stata module to facilitate analysis of the data from the PISA OECD study
10.9.3.5. from what I understood: we use weights and replicates in making any estimates or inferences. the weights used in PISA are Balanced Repeated Replicate in addition to Fay [i don't understand what that is] while in TIMSS the calculation of wieghts is different. weights is part of the Discriminate Function Analysis. Kline talked about it in her boos of phychometrics in the chapter of validity
10.9.3.5.1. I found this explanation of the codes to be so helpful
10.9.3.5.2. this one is also helpful
10.9.3.5.3. The technical baground 2012 says: In Iceland, Liechtenstein, Luxembourg, Macao-China and Qatar, all PISA-eligible students were selected for participation in PISA. It might be unexpected that the PISA data should reflect any sampling variance in these countries/economies, but students have been assigned to variance strata and variance units, and the BRR method does provide a positive estimate of sampling variance for two reasons.
10.9.4. Proficiency Scale Construction Introduction-- chapter 15 in PISA 2012 technical report
10.9.4.1. described proficiency scales OR learning metrics
10.9.4.2. For many years, the Australian Council for Educational Research (ACER) has used and progressively refined an approach to substantive interpretation of scales based on item calibration, employing a reporting mechanism generally known as “described proficiency scales”,
10.9.4.3. PISA has adopted an approach to reporting survey outcomes that involves the development of learning metrics, which are dimensions of educational progression. A learning metric is usually depicted as a line with numerical gradations that quantify how much of the measured variable is present. Locations along this metric can be specified by numerical ‘scores’, or can be described substantively, hence the label for these metrics used in PISA: described proficiency scales. The scales are called “proficiency scales” rather than “performance scales” because they report what students typically know and can do at given levels, rather than what the individuals who were tested actually did on a single occasion (the test administration). This is because PISA is interested in reporting general results, rather than the results of individuals. PISA uses samples of students and items to make estimates about populations: a sample of 15-year-old students is selected to represent all the 15-year-olds in a country, and a sample of test items from a large pool is administered to each student. Results are then analysed using statistical models that estimate the likely proficiency of the population, based on this sampling.
10.9.5. PISA 2012 Assessment and Analytical Framework
10.9.5.1. check the framework of designing questionnaire items. It's very useful in explaining the conceptual framework behind designing the items.
10.9.6. Scaling Procedures and Construct Validation of Context Questionnaire Data---- in the technical report
10.10. transforming data
10.10.1. HOW DO I STANDARDIZE VARIABLES IN STATA? | STATA FAQ
10.10.2. dummy variables in stata
11. Hi! I'm a Ph.D student in the Educational Leadership program. I've created this map to organize my learning of quantitative research. I hope you find it useful. If you have any comments regarding this map, please email me [email protected]
12. validity
12.1. structural validity
12.2. the use of factor analysis in validation
12.2.1. exploratory vs confirmatory factor analysis
12.2.1.1. .
12.2.1.2. exploratory factor analysis is not used to make a theory out of factor analysis statistics
12.2.1.3. the logic behind factor analysis is always confirmatory. Then what is the purpose of exploratory? the difference with the exploratory factor analysis is that we are not telling the computer what I want to find. I just give it the data and see if he can figure the model that I have in mind.
12.2.1.4. confirmatory factor analysis I tell the computer the structure that I have in mind and then ask it to tell me if the structure of the data "fit" the structure of inquiry
12.2.2. factor analysis and structural equation model
12.3. researching big data
12.3.1. inductionism
12.3.1.1. Dustbowl empricism
12.3.1.1.1. Dr. Suen says that Dustbowl empricism is similar to grounded theory, where the researcher comes without expectations
12.4. in research we are interested in "latent variables" and identify their "manifest variables"
12.5. validity generalization: challenges (look at the chapter of assessing validity in the psychometrics textbook (in particular the section of group differences and test bias)
12.5.1. seperate validity
12.5.2. sampling fluctuation (bouncing beta)
12.5.2.1. empirical bayes
12.5.3. .
12.5.4. scholars: Schmidt and Hunter
12.5.4.1. known V-model
12.5.5. it looks like a meta analysis of a group of validity studies
12.5.6. variance in (r)
12.5.6.1. unreliability of test scores
12.5.6.2. unreliability of criterion measure
12.5.6.3. limited range of scores
12.5.6.4. genuine difference
12.5.7. correction for reliability attentuation
12.5.7.1. check p. 215 in Kline, T. J. (2005). Psychological testing: A practical approach to design and evaluation. Sage Publications.
12.6. criterion related validity
12.6.1. concurrent validty
12.6.2. predictive validity
12.7. bias
12.7.1. differential item functioning (DIF)
12.7.1.1. simply: to check if the items behave in the same way when used for different groups of people.
12.7.1.2. the primary method to detect test bias today is through the detection and elimination or modification of biased items or tasks within the measurement tool. this is accomplished through a two-step process of differential item functioning, better known by its acronym DIF, which consists of analyses followed by sensitivity reviews. DIF analyses are a group of statistical techniques to identify items or tasks that have somehow led to different responses from respondents who have the same level of trait or ability but are member of different groups. sensitivity reviews is a judgmental exercise in which an independent panel of content area experts from different groups reviews the items that have shown DIF. the purpose is to discern whether bias is the cause of DIF for each of the items. Biased items would then be eliminated or modified as appropriate (p. 646)
12.7.1.3. Statistics versus reasoning As with all psychological research and psychometric evaluation, statistics play a vital role but should by no means be the sole basis for decisions and conclusions reached. Reasoned judgment is of critical importance when evaluating items for DIF. For instance, depending on the statistical procedure used for DIF detection, differing results may be yielded. Some procedures are more precise while others less so. For instance, the Mantel-Haenszel procedure requires the researcher to construct ability levels based on total test scores whereas IRT more effectively places individuals along the latent trait or ability continuum. Thus, one procedure may indicate DIF for certain items while others do not. Another issue is that sometimes DIF may be indicated but there is no clear reason why DIF exists. This is where reasoned judgment comes into play. The researcher must use common sense to derive meaning from DIF analyses. It is not enough to report that items function differently for groups, there needs to be a theoretical reason for why it occurs. Furthermore, evidence of DIF does not directly translate into unfairness in the test. It is common in DIF studies to identify some items that suggest DIF. This may be an indication of problematic items that need to be revised or omitted and not necessarily an indication of an unfair test. Therefore, DIF analysis can be considered a useful tool for item analysis but is more effective when combined with theoretical reasoning.
12.7.2. Adaptations Not Translations! very good justification of how the adaptation process is more appropriate for comparable tests rather than translation
12.8. resources
12.8.1. validity and reliability course
12.9. types of validity (there are many types. but here are some of them
12.9.1. face validity
12.9.1.1. this defenition that doesn't give a positive implication because it means that a test is valid because it looks like valid. in other words, the format and the wording of its items makes it look like being valid.
12.9.2. content validity
12.9.3. criterion validity
12.9.3.1. criterion related validity assessment is "a technique has been called in the past criterion validity and the correlations produced are called validity coefficients Kline, T. J. (2005). Psychological testing: A practical approach to design and evaluation. Sage Publications.
12.9.4. construct validity
12.9.4.1. Constructs are abstractions that are deliberately created by researchers in order to conceptualize the latent variable, which is the cause of scores on a given measure (although it is not directly observable). Construct validity examines the question: Does the measure behave like the theory says a measure of that construct should behave?
12.9.4.2. Evaluation of construct validity requires that the correlations of the measure be examined in regard to variables that are known to be related to the construct
12.9.4.3. methods of evaluating construct validity: multitrait multimethod matrix (MTMM), factor analysis, SEM
12.9.4.3.1. The multitrait-multimethod (MTMM) matrix
12.9.4.4. It is important to note that a single study does not prove construct validity. Rather it is a continuous process of evaluation, reevaluation, refinement, and development.
12.9.4.5. Most researchers attempt to test the construct validity before the main research. To do this pilot studies may be utilized. Pilot studies are small scale preliminary studies aimed at testing the feasibility of a full-scale test. These pilot studies establish the strength of their research and allow them to make any necessary adjustments.
12.9.4.6. convergent and discriminate validity
12.9.4.6.1. Convergent and discriminant validity are the two subtypes of validity that make up construct validity. Convergent validity refers to the degree to which two measures of constructs that theoretically should be related, are in fact related. In contrast discriminant validity tests whether concepts or measurements that are supposed to be unrelated are, in fact, unrelated.[14] Take, for example, a construct of general happiness. If a measure of general happiness had convergent validity, then constructs similar to happiness (satisfaction, contentment, cheerfulness, etc.) should relate closely to the measure of general happiness. If this measure has discriminate validity, then constructs that are not supposed to be related to general happiness (sadness, depression, despair, etc.) should not relate to the measure of general happiness. Measures can have one of the subtypes of construct validity and not the other. Using the example of general happiness, a researcher could create an inventory where there is a very high correlation between general happiness and contentment, but if there is also a significant correlation between happiness and depression, then the measure's construct validity is called into question. The test has convergent validity but not discriminant validity.
12.9.4.6.2. Convergent evidence is best interpreted relative to discriminant evidence. That is, patterns of intercorrelations between two dissimilar measures should be low while correlations with similar measures should be substantially greater. This evidence can be organized as a multitrait-multimethod matrix
12.10. Salkind, N. J. (Ed.). (2008). Encyclopedia of educational psychology. Sage Publications.
12.10.1. the chapter of validity in this book (p. 646) contains many of the concepts discussed in EDSPY 555
12.11. sytemic validity
12.11.1. it's not about the validity of the test but it is about the validity of the interpretations of the test.
12.11.1.1. the interpretation of the test maybe is based on the goals of assessment
12.11.2. consequential validity
13. reliability
13.1. reliability Difference from validity
13.1.1. Reliability does not imply validity. That is, a reliable measure that is measuring something consistently is not necessarily measuring what you want to be measuring. For example, while there are many reliable tests of specific abilities, not all of them would be valid for predicting, say, job performance. While reliability does not imply validity, a lack of reliability does place a limit on the overall validity of a test. A test that is not perfectly reliable cannot be perfectly valid, either as a means of measuring attributes of a person or as a means of predicting scores on a criterion. While a reliable test may provide useful valid information, a test that is not reliable cannot possibly be valid.
13.1.2. reliability is about precision. validity is about accuracy
13.1.3. often the analyses to assess an instrument's psychometric soundness will provide evidence for both reliability and validity. in many instances, the two issues are strongly tied. however, from a pedagogical perspective, it is useful to separate those analyses most closely linked with reliability from those most closely linked with validity. Keep in mind, though, that the two psychometric properties are not mutually exclusive. Kline, T. J. (2005). Psychological testing: A practical approach to design and evaluation. Sage Publications.
13.2. The goal of estimating reliability is to determine how much of the variability in test scores is due to errors in measurement and how much is due to variability in true scores. A true score is the replicable feature of the concept being measured. It is the part of the observed score that would recur across different measurement occasions in the absence of error. Errors of measurement are composed of both random error and systematic error. It represents the discrepancies between scores obtained on tests and the corresponding true scores. This conceptual breakdown is typically represented by the simple equation: Observed test score = true score + errors of measurement
13.2.1. The goal of reliability theory is to estimate errors in measurement and to suggest ways of improving tests so that errors are minimized.
13.3. two sources of variation
13.3.1. true variation
13.3.2. measurement error
13.3.2.1. random error
13.3.2.2. systematic error
13.4. reliability of a composite score: combining multiple tests
14. Item Response theory
14.1. tutorial video in Arabic language
14.2. This tutorial, which is a practical introduction to Item Response Theory (IRT)
14.3. Theory of conjoint measurement
14.4. test equating
14.5. best simple description of IRT
14.5.1. the explanation of the Data Anlyhsis Manual of PISA 2009 is even better
14.6. Measurement Essentials 2nd Edition by Benjamin Wright & Mark Stone
14.7. Royal, K. D. (2010). Making meaningful measurement in survey research: A demonstration of the utility of the Rasch model. IR Applications, 28, pp. 1-16.
14.7.1. Item parameters might include factors such as difficulty, discrimination, and guessing. From the Rasch perspective, factors such as discrimination and guessing violate the strict theoretical underpinnings of the model, as a requirement for objective measurement is to measure only one construct at a time.
14.7.2. Today, the Rasch model is the most popular and widely used IRT technique. This is due largely to the Rasch model’s concern with only one parameter (such as ability on a test, or in the case of a survey, the strength of one’s attitude), as two and three parameters (which control for factors such as discrimination and guessing) often do not apply. Further, 2PL and 3PL approaches are very complex, require significantly larger sample sizes than the 1PL Rasch model, and 2PL and 3PL models require a great deal of technical expertise to perform the analyses. It is, in part, for these reasons that this paper will focus on the application of the Rasch model
14.7.3. unidimetionality
14.7.3.1. Typically, resistance to the Rasch model is met with criticisms of unidimensionality.
14.7.3.2. a unidimensional construct seems too simplistic. These critics are usually unaware that unidimensionality is a requirement of objective measurement.
14.7.4. Rasch model is the only model that is considered objective measurement.
14.7.5. Rasch vs factor analysis
14.7.5.1. Although factor analysis is useful for reducing data in an exploratory manner, it is bound by the characteristics of the sample and requires larger sample sizes (Kline, 1994). Rasch measurement, on the other hand, is not a sample- dependent technique.
14.7.5.2. h Rasch and factor analysis resulted in similar results, but Rasch results were more informative, more stable, and easier to interpret. The author found factor analysis is good for identifying proximity to the underlying variable, but not so good at identifying location in a vector space of other variables. Rasch analysis, on the other hand, provided locations for both persons and items on the variable. This is especially helpful in developing a construct theory
14.7.6. Rasch vs SEM
14.7.6.1. SEM allows researchers to specify which variables they want to investigate (usually based on some theoretical reason), as well as specify the relationships between the variables along with associated error components. The data are then analyzed to determine the extent to which they fit a given structure. An investigation of residuals can be extremely useful in identifying areas of the theory that need to be improved, modified, or removed. In some ways, Rasch modeling can be considered a form of SEM. They share a similar philosophy in that data should fit the model, as opposed to generating models that describe data. This is certainly a step in the right direction with regard to making meaningful measurement.
14.7.7. fitting the data
14.7.7.1. Rasch models require data to fit the model. An investigation of fit statistics largely determines whether the data are unidimensional in nature.
14.7.7.2. Both infit and outfit statistics are evaluated to determine how data-to-model fit occurs for each item and person. Infit statistics are fit statistics that are sensitive to the inlier pattern of observations. Outfit statistics are sensitive to outlier observations.
14.7.7.3. "fit" as an indicator of validity
14.7.7.3.1. In survey research, infit and outfit statistics are incredibly useful for identifying problematic items or persons who appear to have “flat-lined” by randomly marking items or simply marking all items with a particular rating. Investigating fit statistics is an excellent quality control element as evidence of data adequately fitting the model is a key indicator of validity.
14.7.7.4. A Principal Components Analysis (PCA) can detect multidimensionality by explaining the variance associated with both persons and items.
14.7.8. item person/ Wright Map
14.7.8.1. To exhibit the power and utility of Rasch measurement, a demonstration of one of its powerful techniques, particularly the use of person and item maps, will be provided in this section. These maps are extremely valuable as they illustrate the construct hierarchy that is being measured by an assessment. These maps are useful for exposing the empirical hierarchy of the dataset, which lends to testing and evaluating existing theories, or possibly generating new theories. It should be noted that under CTT models and traditional statistical software packages, this technique cannot be performed.
14.7.8.2. These maps have the ability to place both persons and items on the same scale, demonstrating how individuals and groups of persons interact with each of the items. This is paramount for making truly meaningful comparisons of results.
14.7.9. keyform/
14.8. Wright map
14.8.1. A powerful yet simple graphical tool available in the field of psychometrics is the Wright Map, which presents the location of both respondents and items on the same scale.
14.8.2. Wright Maps are commonly used to present the results of dichotomous or polytomous item response models.
14.8.3. Using The Very Useful Wright Map
14.9. STATA ITEM RESPONSE THEORY REFERENCE MANUAL
14.9.1. running IRT analysis in STATA
14.10. Green, K. E., & Frantom, C. G. (2002, November). Survey development and validation with the Rasch model. In International Conference on Questionnaire Development, Evaluation, and Testing, Charleston, SC.
14.10.1. model fit
14.10.1.1. fit statistics
14.10.1.1.1. Fit statistics provide the indices of fit of the data to the model and usefulness of the measure. Fit statistics include the average fit (mean square and standardized) of persons and items, and fit statistics reflecting the appropriateness of rating scale category use. The fit statistics are calculated by differencing each pair of observed and model-expected responses, squaring the differences, summing over all pairs, averaging, and standardizing to approximate a unit normal (z) distribution. The expected values of the mean square and standardized fit indices are 1.0 and 0.0, respectively, if the data fit the model. Fit is expressed as "infit" (weighted by the distance between the person position and item difficulty) and as "outfit" (an unweighted measure). Infit is less sensitive than outfit to extreme responses.
14.10.1.1.2. Person fit to the Rasch model is an index of whether individuals are responding to items in a consistent manner or if responses are idiosyncratic or erratic. Responses may fail to be consistent when people are bored and inattentive to the task, when they are confused, or when an item evokes an unusually salient response from an individual
14.10.1.1.3. item fit is an index of whether items function logically and provide a continuum useful for all respondents. An item may "misfit" because it is too complex, confusing, or because it actually measures a different construct
14.10.1.1.4. Values for differentiating "fit" and "misfit" are arbitrary and should be sufficiently flexible to allow for researcher judgment. Also,
14.10.1.2. The chi- squares in common use are known as OUTFIT and INFIT. These are reported as mean-squares, chi-square statistics divided by their degrees of freedom, so that they have a ratio-scale form with expectation 1 and range 0 to +infinity. They are also reported in various interval-scale forms in which their expected value is zero.
14.10.1.3. infit vs outfit
14.10.1.3.1. Fit statistics are formulated to test particular hypotheses. OUTFIT is dominated by unexpected outlying, off-target, low information responses and so is outlier-sensitive. INFIT is dominated by unexpected inlying patterns among informative, on-target observations and so is inlier-sensitive.
14.10.1.3.2. determine the fit of persons and items to the construct, Rasch analysis produces both infit and outfit statistics, which have two forms: one unstand- ardized (mean squares) and one standardized (z-scores) (Linacre, 2002). produces both infit and outfit statistics, which have two forms: one unstand- ardized (mean squares) and one standardized (z-scores)
14.10.1.3.3. Researchers typically pay more attention to infit in the interests of deter-mining the quality of items as they apply to the majority of respondents
14.10.1.3.4. Misfit diagnosis: infit outfit mean-square standardized (very useful)
14.10.1.3.5. Dichotomous Infit and Outfit Mean-Square Fit Statistics
14.10.1.3.6. What do Infit and Outfit, Mean-square and Standardized mean?
14.10.1.4. Yu, C. H. (2011). A simple guide to the item response theory (IRT) and Rasch modeling. Retrieved on, 14.
14.10.1.4.1. this article has very detailed explanation of the fit vs misfit and infit vs outfit
14.10.1.4.2. misfit: In the context of classical test theory, this type of items is typically detected by either point-biserial correlation or factor analysis. In IRT it is identified by examining the misfit indices.
14.10.1.4.3. the distribution of standardized residuals informs us about the goodness or badness of the model fit.
14.10.1.4.4. The objective of computing item fit indices is to spot misfits.
14.10.1.5. tutorial of fit vs misfit
14.10.1.6. Rasch Power Analysis: Size vs. Significance: Infit and Outfit Mean-Square and Standardized Chi-Square Fit Statistic
14.10.1.7. Evaluating Model Fit with IRT
14.10.1.7.1. We hope that the chi-square test will NOT be significant: be significant: – This indicates that the differences between observed and expected is small observed and expected is small. – Significant differences would mean that observed proportions are far from what the observed proportions are far from what the model predicted…and that’s bad.
14.10.1.8. Reise, S. P. (1990). A comparison of item-and person-fit methods of assessing model-data fit in IRT. Applied Psychological Measurement, 14(2), 127-137.
14.10.2. instrument reliability
14.10.2.1. instrument’s reliability was estimated using four statistics: (a) person reliability (to determine the consistency of person responses), (b) person separation (to estimate the ability of the instrument to separate participants into different levels of the construct), (c) item reli-ability (to estimate how well the items cohered), and (d) item separation (to estimate the ability of the participants to distinguish between items meas-uring different levels of the construct)
14.10.3. separation index
14.10.3.1. Person and item separation and reliability of separation assess instrument spread across the trait continuum. “Separation” measures the spread of both items and persons in standard error units. It can be thought of as the number of levels into which the sample of items and persons can be separated. For an instrument to be useful, separation should exceed 1.0, with higher values of separation representing greater spread of items and persons along a continuum. Lower values of separation indicate redundancy in the items and less variability of persons on the trait. To operationalize a variable with items, each item should mark a different amount of the trait, as for instance, the way marks on a ruler form a measure of length. Separation, in turn, determines reliability. Higher separation in concert with variance in person or item position yields higher reliability. Reliability of person separation is conceptually equivalent to Cronbach's alpha, though the formulas are different.
14.10.3.2. Rating categories within items should form a continuum of less to more. That is, endorsing a lower category should represent being lower on the trait, e.g., “a little like me,” than endorsing a higher category, which would be, e.g., “a lot like me”. Lack of order in rating scale categories suggests a lack of common understanding of use of the rating scale between the researcher and the participants. Inconsistent use of the rating scale affects item fit and placement.
14.10.3.3. A "good" test shows an even spread of items along the variable void of gaps and targeted to person ability
14.10.4. Data Requirements for Design and Analysis with the Rasch Model
14.10.4.1. A panel of experts can be a valuable resource for judging the difficulty level of items through a sorting process. A hierarchical ordering of items by the panel of experts that is similar to the ordering determined by the primary researchers would suggest that they have a common understanding of the construct. The empirical item order would be expected to conform to a similar pattern. An instrument best defines a trait when the items written to support it function consistently throughout the instrument development process. Inconsistencies can suggest areas for reconsideration.
14.10.4.2. A sample size of at least 100 and a minimum of at least 20 items are suggested for obtaining stable indices when using Rasch analysis.
14.10.4.3. The Rasch model can be used with categorical data, rating scale data, or frequency count data.
14.11. ten Holt, J. C., van Duijn, M. A., & Boomsma, A. (2010). Scale construction and evaluation in practice: A review of factor analysis versus item response theory applications. Psychological Test and Assessment Modeling.
14.11.1. Factor analysis (FA) and item response theory (IRT) are two types of models used for scale analysis.
14.11.2. Of primary interest is the researchers’ motivation for choosing either methodology. It is of secondary (methodological) interest to investigate how the chosen analysis is performed and what results are reported.
14.11.3. FA and IRT have also been compared in their usefulness for investiga-tions of measurement equivalence
14.11.4. It seems that, in practice, IRT is primarily applied to investigate unidimensional scales.
14.11.5. the guidelines for applying quantitative methods in the social sciences in a book edited by Hancock and Mueller (2010) might be a useful reference for both authors and reviewers. These guidelines concern model choice as well as reporting practice.
14.11.6. it can be concluded that EFA performs reasonably well at recovering a hypothesized factor structure. Results of CFA and EFA may often be different, with CFA fit measures indicating an unsatisfactory fit of structures uncovered by EFA.
14.12. practical examples of IRT (Rasch Model)
14.12.1. Apple, M. (2013). Using Rasch Analysis to Create and Evaluate a Measurement Instrument for Foreign Language Classroom Speaking Anxiety. JALT J, 35(1), 5-28.
14.12.2. MUSA, N. A. C., MAHMUD, Z., & GHANI, N. A. M. Application of Rasch Measurement Model in Validation and Analysis of Measurement Instruments in Statistical Education Research.
14.13. IRT in PISA. Check PISA 2012 technical report, p. 132. the section of 16 SCALING PROCEDURES AND CONSTRUCT VALIDATION OF CONTEXT QUESTIONNAIRE DATA SCALING METHODOLOGY AND CONSTRUCT VALIDATION
14.14. detect cheating in instrument that use IRT
14.15. partially credit Rasch Model
14.15.1. Reise, S. P. (1990). A comparison of item-and person-fit methods of assessing model-data fit in IRT. Applied Psychological Measurement, 14(2), 127-137.
14.15.1.1. it explains why factor analysis doesn't work with likert scale responses. Factor analysis assume that the responses are ratio or interval
14.15.2. Kelderman, H. (1996). Multidimensional Rasch models for partial-credit scoring. Applied Psychological Measurement, 20(2), 155-168.
14.15.3. Salzberger, T. (2015). The validity of polytomous items in the Rasch model–The role of statistical evidence of the threshold order. Psychological Test and Assessment Modeling, 3, 377-395.
14.15.4. Baglin, J. (2014). Improving your exploratory factor analysis for ordinal data: a demonstration using FACTOR. Practical Assessment, Research & Evaluation, 19(5), 2.
15. data visualization
15.1. 7 Data Visualization Types You Should be Using More (and How to Start)
15.1.1. .
15.2. Data Visualization Field Subgroups
15.2.1. .
15.3. What are good ways of visualizing many effects ordered in groups and subgroups?
15.3.1. .
15.4. Vehkalahti, K. (2008). Handbook of Data Visualization edited by Chun‐houh Chen, Wolfgang Härdle, Antony Unwin. International Statistical Review, 76(3), 442-443.
15.4.1. available online
15.5. story telling with data
16. calculus and algebra for stat
16.1. Statistics Symbol Sheet
16.1.1. another symbol sheet
16.2. density function
16.3. continuous random variable
16.4. discrete variables
16.5. modeling with functions
16.5.1. .
16.5.2. linear and non linear equations
16.5.3. Eight graphs of different types of functions
16.5.4. Functions versus Relations
16.6. Using Logarithms in the Real World
16.7. notations
16.7.1. THE ALGEBRA OF SUMMATION NOTATION
17. sampling
17.1. sampling with replacement/without replacement
17.2. confidence intervals for the population mean
17.2.1. standard error vs standard deviation
17.2.2. what's the difference between margin of error and confidence intervals
17.3. sample size
17.3.1. selecting a sample size
17.4. population proportion
17.5. difference between population and sample
17.5.1. Inferential statistics enables you to make an educated guess about a population parameter based on a statistic computed from a sample randomly drawn from that population
17.5.2. While the population characteristic remains fixed, the estimate of it depends on which sample is selected. (Thompson)
17.6. sampling design: the procedure by which the sampled units is selected from the population Thompson (p. 25)
17.6.1. A trick performed with many of the most useful sampling designs—cleverer than it may appear at first glance—is that this variability from sample to sample is estimated using only the single sample selected.
17.6.2. simple random sampling
17.6.2.1. With simple random sampling, the sample mean y is an unbiased estimator of the population mean μ. (Thompson)
17.6.3. cluster sampling
17.6.3.1. primary and secondary units
17.6.3.1.1. from STAT 506
17.6.3.2. Cluster Sampling
17.7. proportion sampling
17.8. a simplified introduction to sampling
17.9. auxilary variables
17.10. types of samples
17.10.1. non probability sampling: These samples focus on volunteers, easily available units, or those that just happen to be present when the research is done. Non-probability samples are useful for quick and cheap studies, for case studies, for qualitative research, for pilot studies, and for developing hypotheses for future research.
17.10.1.1. Convenience sample: also called an "accidental" sample or "man-in-the-street" samples. The researcher selects units that are convenient, close at hand, easy to reach, etc.
17.10.1.2. Purposive sample: the researcher selects the units with some purpose in mind, for example, students who live in dorms on campus, or experts on urban development.
17.10.1.3. Quota sample: the researcher constructs quotas for different types of units. For example, to interview a fixed number of shoppers at a mall, half of whom are male and half of whom are female.
17.10.1.4. Other samples that are usually constructed with non-probability methods include library research, participant observation, marketing research, consulting with experts, and comparing organizations, nations, or governments.
17.10.2. Probability-based (random) samples: These samples are based on probability theory. Every unit of the population of interest must be identified, and all units must have a known, non-zero chance of being selected into the sample.
17.10.2.1. Simple random sample: Each unit in the population is identified, and each unit has an equal chance of being in the sample. The selection of each unit is independent of the selection of every other unit. Selection of one unit does not affect the chances of any other unit.
17.10.2.2. Systematic random sampling: Each unit in the population is identified, and each unit has an equal chance of being in the sample.
17.10.2.3. Stratified random sampling: Each unit in the population is identified, and each unit has a known, non-zero chance of being in the sample. This is used when the researcher knows that the population has sub-groups (strata) that are of interest.
17.10.2.4. Stratified random sampling: Each unit in the population is identified, and each unit has a known, non-zero chance of being in the sample. This is used when the researcher knows that the population has sub-groups (strata) that are of interest.